基于氨基酸序列及药物化合物分子指纹的药物-靶标相互作用预测研究
项目介绍
AI项目解读
基本信息
- 批准号:61572506
- 项目类别:面上项目
- 资助金额:64.0万
- 负责人:
- 依托单位:
- 学科分类:F0213.生物信息计算与数字健康
- 结题年份:2019
- 批准年份:2015
- 项目状态:已结题
- 起止时间:2016-01-01 至2019-12-31
- 项目参与者:夏战国; 闫秋艳; 张辰; 安计勇; 李雯; 王伟东; 孙金亮;
- 关键词:
项目摘要
Identifying potential drug targets is very important for modern drug discovery, drug side effects evaluation and new uses for old drugs. However, the biological experiments are usually low-throughput, inaccurate and expensive. Therefore, there is an urgent need to develop novel in silicon prediction approaches capable of identifying potential drug–target interactions in a timely manner.In this project, we aim at predicting the drug-target interactions by employing the amino acids sequence information and the fingerprints for drugs. Firstly, mapping each protein sequence into a matrix built on all kinds of adjacent amino acids and applying the low rank approximation model to the obtained matrix to extract its feature representation. Then, drug molecules are encoded with fingerings which are a kind of binary fragment descriptors. Finally, two computational approaches are generally employed for studying the drug-target relations: the multiple classifiers learning algorithms and the machine learning algorithms based on Hadoop distributed platform. Our research will provide a new theoretical guidance to drug research and development.
药物潜在靶标的识别对于创新药物的研发、药物副作用评价及旧药新用等领域都有着非常重要的意义。然而受制于通量、精度和成本的限制,生物实验方法通常难以广泛开展。作为一种快速而低成本的手段,计算机辅助的药物-靶标识别方法正受到越来越多的重视。如何发展快速、精确的药物-靶标相互作用预测方法,成为信息学家和药物学家面临的新问题。本项目研究基于氨基酸序列信息及药物化合物分子指纹信息,并结合机器学习方法和模式识别理论,对药物与靶标相互作用进行预测研究。首先,研究氨基酸序列的矩阵表示及基于低秩逼近的特征编码新方法;研究基于分子指纹的药物化合物结构数值序列描述新方法,从而抽取出它们所蕴含的能定量刻画其内在本质属性的特征信息。然后,基于非均衡数据集的多分类器集成方法和基于Hadoop的分布式机器学习框架被用来快速、精确的预测药物-靶标之间的相互作用关系。本项目的研究成果将为靶向药物的设计提供理论指导。
结项摘要
药物潜在靶标的识别对于创新药物的研发、药物副作用评价及旧药新用等领域都有着非常重要的意义。然而受制于通量、精度和成本的限制,生物实验方法通常难以广泛开展。作为一种快速而低成本的手段,计算机辅助的药物-靶标识别方法正受到越来越多的重视。如何发展快速、精确的药物-靶标相互作用预测方法,成为信息学家和药物学家面临的新问题。本项目从氨基酸序列信息及药物化合物分子指纹信息出发,并结合机器学习方法和模式识别理论,在药物与靶标相互作用预测研究方面进行了深入研究。主要研究包括:1)通过自动挖掘蛋白质序列中隐藏的信息和药物分子结构,项目提出了一种新的采用堆叠自编码深度学习的计算方法用于预测药物-靶标相互作用。(2)结合药物化合物结构信息和蛋白质氨基酸序列信息,项目提出一种基于集合分类器的方法来预测潜在药物-靶标相互作用。(3)通过整合已知人类miRNA与疾病相互关系、miRNA功能类似性、疾病语义类似性以及基于高斯相互关系谱核类似性,提出了基于路径的miRNA与疾病关系预测模型(PBMDA)。本项目的研究成果将为靶向药物的设计提供理论指导。
项目成果
期刊论文数量(40)
专著数量(0)
科研奖励数量(0)
会议论文数量(0)
专利数量(0)
A Rotation Forest-based Predictor for Predicting Drug-TargetInteractions using Drug Structure and Protein Sequence Information
基于旋转森林的预测器,用于使用药物结构和蛋白质序列信息预测药物-靶标相互作用
- DOI:--
- 发表时间:2017
- 期刊:Current Protein & Peptide Science
- 影响因子:2.8
- 作者:Lei Wang;Zhu-Hong You;Xing Chen
- 通讯作者:Xing Chen
LMTRDA: Using logistic model tree to predict MiRNA-disease associations by fusing multi-source information of sequences and similarities
LMTRDA:通过融合序列和相似性的多源信息,使用逻辑模型树来预测 miRNA 与疾病的关联
- DOI:10.1371/journal.pcbi.1006865
- 发表时间:2019-03-01
- 期刊:PLOS COMPUTATIONAL BIOLOGY
- 影响因子:4.3
- 作者:Wang, Lei;You, Zhu-Hong;Zheng, Kai
- 通讯作者:Zheng, Kai
PSPEL: In Silico Prediction of Self-Interacting Proteins from Amino Acids Sequences Using Ensemble Learning
PSPEL:使用集成学习从氨基酸序列中进行自相互作用蛋白质的计算机预测
- DOI:10.1109/tcbb.2017.2649529
- 发表时间:2017-09-01
- 期刊:IEEE-ACM TRANSACTIONS ON COMPUTATIONAL BIOLOGY AND BIOINFORMATICS
- 影响因子:4.5
- 作者:Li, Jian-Qiang;You, Zhu-Hong;Chen, Xing
- 通讯作者:Chen, Xing
An improved sequence-based prediction protocol for protein-protein interactions using amino acids substitution matrix and rotation forest ensemble classifiers
使用氨基酸替换矩阵和旋转森林集成分类器改进的基于序列的蛋白质-蛋白质相互作用预测协议
- DOI:10.1016/j.neucom.2016.10.042
- 发表时间:2017-03-08
- 期刊:NEUROCOMPUTING
- 影响因子:6
- 作者:You, Zhu-Hong;Li, Xiao;Chan, Keith C. C.
- 通讯作者:Chan, Keith C. C.
Learning Multimodal Networks From Heterogeneous Data for Prediction of lncRNA-miRNA Interactions
从异构数据中学习多模态网络以预测 lncRNA-miRNA 相互作用
- DOI:10.1109/tcbb.2019.2957094
- 发表时间:2020-09-01
- 期刊:IEEE-ACM TRANSACTIONS ON COMPUTATIONAL BIOLOGY AND BIOINFORMATICS
- 影响因子:4.5
- 作者:Hu, Pengwei;Huang, Yu-An;You, Zhu-Hong
- 通讯作者:You, Zhu-Hong
数据更新时间:{{ journalArticles.updateTime }}
{{
item.title }}
{{ item.translation_title }}
- DOI:{{ item.doi || "--"}}
- 发表时间:{{ item.publish_year || "--" }}
- 期刊:{{ item.journal_name }}
- 影响因子:{{ item.factor || "--"}}
- 作者:{{ item.authors }}
- 通讯作者:{{ item.author }}
数据更新时间:{{ journalArticles.updateTime }}
{{ item.title }}
- 作者:{{ item.authors }}
数据更新时间:{{ monograph.updateTime }}
{{ item.title }}
- 作者:{{ item.authors }}
数据更新时间:{{ sciAawards.updateTime }}
{{ item.title }}
- 作者:{{ item.authors }}
数据更新时间:{{ conferencePapers.updateTime }}
{{ item.title }}
- 作者:{{ item.authors }}
数据更新时间:{{ patent.updateTime }}
其他文献
A Novel Robust Geometric Approach to Model Protein-Protein Interaction Networks
一种新颖的稳健几何方法来模拟蛋白质-蛋白质相互作用网络
- DOI:--
- 发表时间:--
- 期刊:Plos One
- 影响因子:3.7
- 作者:尤著宏
- 通讯作者:尤著宏
基于深度学习的 miRNA 与疾病相关性预测算法
- DOI:--
- 发表时间:2019
- 期刊:电子学报
- 影响因子:--
- 作者:王磊;徐涛;宋传东;王海峰;尤著宏;宋克俭;闫欣
- 通讯作者:闫欣
其他文献
{{
item.title }}
{{ item.translation_title }}
- DOI:{{ item.doi || "--" }}
- 发表时间:{{ item.publish_year || "--"}}
- 期刊:{{ item.journal_name }}
- 影响因子:{{ item.factor || "--" }}
- 作者:{{ item.authors }}
- 通讯作者:{{ item.author }}
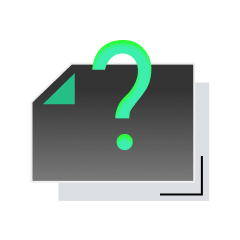
内容获取失败,请点击重试
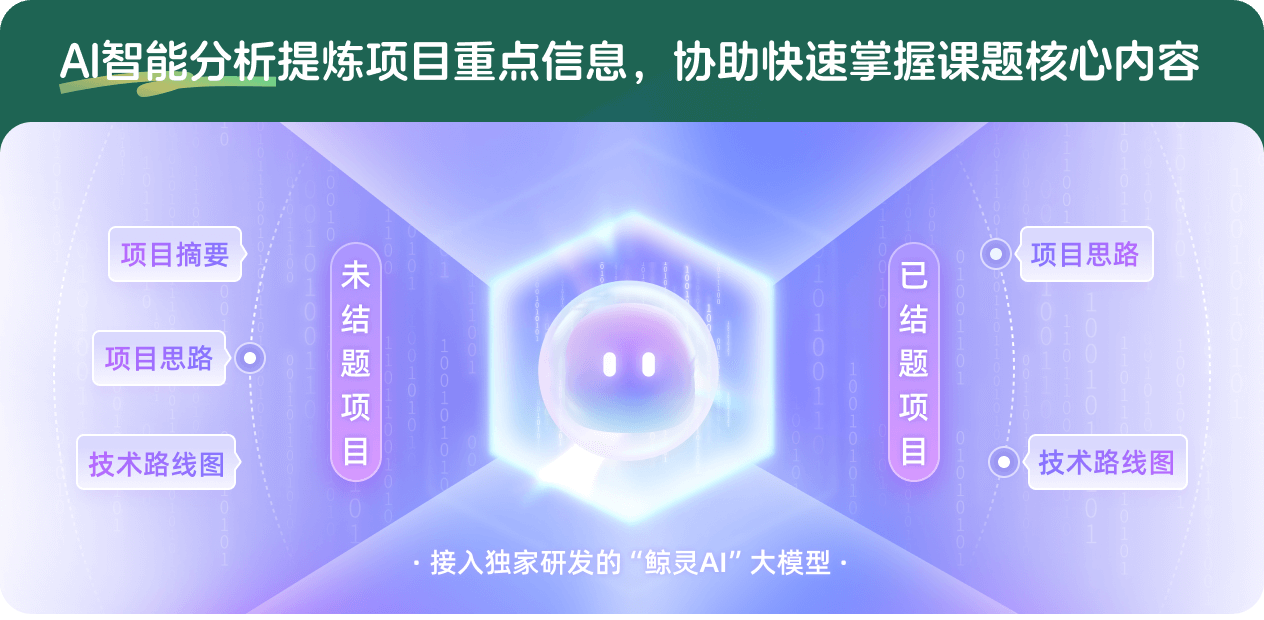
查看分析示例
此项目为已结题,我已根据课题信息分析并撰写以下内容,帮您拓宽课题思路:
AI项目摘要
AI项目思路
AI技术路线图
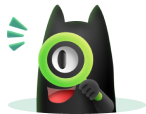
请为本次AI项目解读的内容对您的实用性打分
非常不实用
非常实用
1
2
3
4
5
6
7
8
9
10
您认为此功能如何分析更能满足您的需求,请填写您的反馈:
相似国自然基金
{{ item.name }}
- 批准号:{{ item.ratify_no }}
- 批准年份:{{ item.approval_year }}
- 资助金额:{{ item.support_num }}
- 项目类别:{{ item.project_type }}
相似海外基金
{{
item.name }}
{{ item.translate_name }}
- 批准号:{{ item.ratify_no }}
- 财政年份:{{ item.approval_year }}
- 资助金额:{{ item.support_num }}
- 项目类别:{{ item.project_type }}