针对时间序列聚类问题的特征学习与集成学习研究
项目介绍
AI项目解读
基本信息
- 批准号:61663046
- 项目类别:地区科学基金项目
- 资助金额:40.0万
- 负责人:
- 依托单位:
- 学科分类:F0603.机器学习
- 结题年份:2020
- 批准年份:2016
- 项目状态:已结题
- 起止时间:2017-01-01 至2020-12-31
- 项目参与者:Xiao-Jun Zeng; 李昕洁; 何臻力; 谢仲文; 刘俊晖; 刘金卓; 朱锐; 杜飞; 胡健;
- 关键词:
项目摘要
Although conventional static data clustering has been studied for many years, time series clustering recently has been becoming quite popular in various fields due to that such underpinning techniques can discover the intrinsic structures and condense or summarize information contained in growing time series datasets. Unlike static data, time series have many distinct characteristics, including high dimensionality, complex time dependency, and large volume, all of which make the clustering of time series more challenging than static data clustering. In this proposal, we intensively study the time series data mining from the perspective of clustering analysis, and proposed an representation learning-based ensemble learning approach via multiple weighting scheme for time series clustering problems. Three key issues are explored in this proposal: (1) the representation learning/deep learning technique is used to optimally capture important features of time series such as dynamic behaviors and temporal coherence. (2) A novel multiple weighting scheme is proposed to optimally reconcile the input partitions into a single consolidated solution that significantly improve the robustness and accuracy of clustering analysis on time series via ensemble learning approach (3) The proposed approaches have out-standing ability in automatic detection of cluster number. Sum of all, we will carry out the forefront research of time series clustering in association of clustering ensemble techniques, the research results will not only contribute to the theoretical analysis, but also applications of time series data mining and pattern recognition.
传统静态数据的聚类方法已经得到了较为深入的研究,然而现实生活中越来越多的应用领域涉及到时间序列数据的聚类分析。但时间序列数据具有复杂的动态特性、高维度和海量性等特点,使得传统的聚类算法无法获得较为理想的结果。本课题将深入研究时间序列数据挖掘技术中的聚类问题,拟提出了一种基于特征学习的复合式加权聚类集成学习模型,以解决以下主要问题:(1)通过提出基于深度学习的特征提取方法,有效地捕捉时间序列的动态特性与时间片段的关联性,并使其根据不同目标时间序列数据集自适应提取特征信息(2)通过引入新的复合式加权机制,优化集成学习模型的融合方式,使得时间序列聚类分析的鲁棒性与精确度得到进一步的提高。(3)在时间序列聚类分析中能够有效地捕捉类簇的本征结构,自动识别类数。综上所述本课题将在特征学习及集成学习的基础上,对时间序列数据聚类分析提出较为前沿的理论研究,其研究成果将具有较高的理论和实用应用价值。
结项摘要
传统静态数据的聚类方法已经得到了较为深入的研究,然而现实生活中越来越多的应用领域涉及到时间序列数据的聚类分析。但时间序列数据具有复杂的动态特性、高维度和海量性等特点,使得传统的聚类算法无法获得较为理想的结果。.本课题深入研究时间序列数据挖掘技术中的聚类问题,提出了基于特征学习的复合式加权聚类集成学习模型,并取得了以下成果:(1)通过提出基于深度学习的特征提取方法,有效地捕捉时间序列的动态特性与时间片段的关联性,并使其根据不同目标时间序列数据集自适应提取特征信息;(2)通过引入新的复合式加权机制,优化集成学习模型的融合方式,使得时间序列聚类分析的鲁棒性与精确度得到进一步的提高;(3)在时间序列聚类分析中能够有效地捕捉类簇的本征结构,自动识别类数。.综上所述本课题将在特征学习及集成学习的基础上,对时间序列数据聚类分析提出较为前沿的理论研究,其研究成果将具有较高的理论和实用应用价值。
项目成果
期刊论文数量(22)
专著数量(2)
科研奖励数量(2)
会议论文数量(18)
专利数量(8)
A Novel Parallel Distance Metric-based Approach for Diversified Ranking on Large Graphs
一种新颖的基于并行距离度量的方法,用于大图上的多样化排名
- DOI:10.1016/j.future.2018.05.031
- 发表时间:2018-11
- 期刊:Future Generation Computer Systems
- 影响因子:--
- 作者:Li J.;Yang Y.;Wang X.;Zhao Z.;Li T.
- 通讯作者:Li T.
基于特征补偿的单目标跟踪算法
- DOI:--
- 发表时间:2020
- 期刊:计算机工程与设计
- 影响因子:--
- 作者:白扬;曹丽娟;胡媛媛;杨云
- 通讯作者:杨云
An adaptive semi-supervised clustering approach via multiple density-based information
通过基于多个密度的信息的自适应半监督聚类方法
- DOI:10.1016/j.neucom.2016.11.061
- 发表时间:2017-09
- 期刊:Neurocomputing
- 影响因子:6
- 作者:Yang Y.;Li Z.;Wang W.;Tao D.
- 通讯作者:Tao D.
Novel Word Similarity Measure Method for IoT-enabled Healthcare Applications, Future Generation Computer Systems
适用于物联网医疗保健应用、下一代计算机系统的新颖单词相似度测量方法
- DOI:--
- 发表时间:2020
- 期刊:Future Generation Computer Systems
- 影响因子:--
- 作者:Dehai Zhang;Xiaoqiang Xia;Yun Yang;Po Yang;Cheng Xie;Menglong Cui;Qing Liu
- 通讯作者:Qing Liu
User Profiling in Elderly Healthcare Services in China: Scalper Detection
中国老年医疗服务用户画像:黄牛检测
- DOI:10.1109/jbhi.2018.2852495
- 发表时间:2018-07
- 期刊:IEEE Journal of Biomedical and Health Informatics
- 影响因子:7.7
- 作者:Xie C.;Cai H.;Yang Y.;Jiang L.;Yang P.
- 通讯作者:Yang P.
数据更新时间:{{ journalArticles.updateTime }}
{{
item.title }}
{{ item.translation_title }}
- DOI:{{ item.doi || "--"}}
- 发表时间:{{ item.publish_year || "--" }}
- 期刊:{{ item.journal_name }}
- 影响因子:{{ item.factor || "--"}}
- 作者:{{ item.authors }}
- 通讯作者:{{ item.author }}
数据更新时间:{{ journalArticles.updateTime }}
{{ item.title }}
- 作者:{{ item.authors }}
数据更新时间:{{ monograph.updateTime }}
{{ item.title }}
- 作者:{{ item.authors }}
数据更新时间:{{ sciAawards.updateTime }}
{{ item.title }}
- 作者:{{ item.authors }}
数据更新时间:{{ conferencePapers.updateTime }}
{{ item.title }}
- 作者:{{ item.authors }}
数据更新时间:{{ patent.updateTime }}
其他文献
基于能量法的摆线针轮传动啮合刚度理论研究
- DOI:10.16578/j.issn.1004.2539.2018.09.002
- 发表时间:2018
- 期刊:机械传动
- 影响因子:--
- 作者:杨云;李朝阳;李轩
- 通讯作者:李轩
12份苜蓿农艺性状的主成分及聚类分析
- DOI:--
- 发表时间:--
- 期刊:草原与草坪
- 影响因子:--
- 作者:武自念;魏臻武;雷艳芳;甘欣;刘高军;杨云;WU Zi-nian1,WEI Zhen-wu1,2,LEI Yan-fang2,GAN Xin1,;2.College of Animal Science & Technique,Yang Zhou
- 通讯作者:2.College of Animal Science & Technique,Yang Zhou
Ce/Tb/Mn共掺杂ZnO-SrO-P_2O_5玻璃陶瓷的制备与白光发射调控
- DOI:--
- 发表时间:2014
- 期刊:中国有色金属学报
- 影响因子:--
- 作者:王家鑫;陈国华;李旭琼;何志毅;袁昌来;杨会娟;杨云
- 通讯作者:杨云
Mn掺杂Ca_(0.16)Sr_(0.04)Li_(0.4)Nd_(0.4)TiO_3微波介质陶瓷介电性能的研究
- DOI:--
- 发表时间:2014
- 期刊:人工晶体学报
- 影响因子:--
- 作者:周秀娟;袁昌来;陈国华;周昌荣;杨云;蒙柳方
- 通讯作者:蒙柳方
一种基于连通域标记的纸病检测算法
- DOI:--
- 发表时间:2018
- 期刊:中国造纸学报
- 影响因子:--
- 作者:赵晓;何立风;姚斌;高启航;杨云
- 通讯作者:杨云
其他文献
{{
item.title }}
{{ item.translation_title }}
- DOI:{{ item.doi || "--" }}
- 发表时间:{{ item.publish_year || "--"}}
- 期刊:{{ item.journal_name }}
- 影响因子:{{ item.factor || "--" }}
- 作者:{{ item.authors }}
- 通讯作者:{{ item.author }}
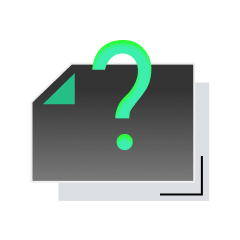
内容获取失败,请点击重试
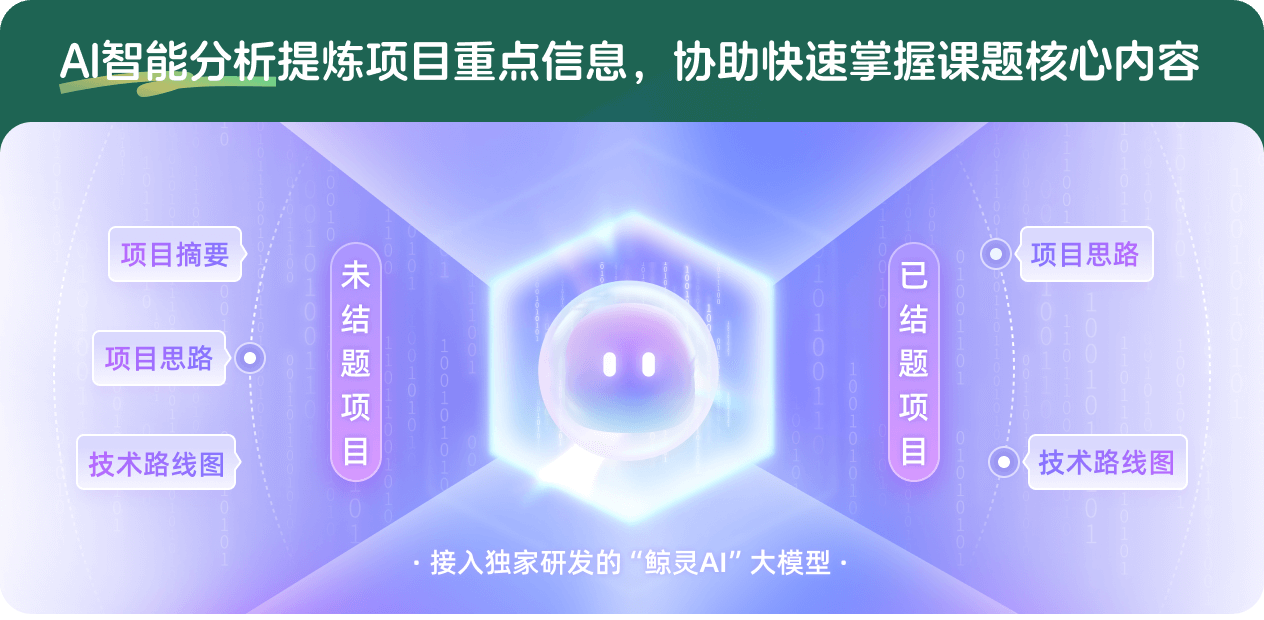
查看分析示例
此项目为已结题,我已根据课题信息分析并撰写以下内容,帮您拓宽课题思路:
AI项目摘要
AI项目思路
AI技术路线图
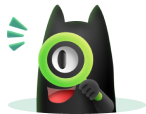
请为本次AI项目解读的内容对您的实用性打分
非常不实用
非常实用
1
2
3
4
5
6
7
8
9
10
您认为此功能如何分析更能满足您的需求,请填写您的反馈:
杨云的其他基金
基于自适应迭代模式的终生学习研究
- 批准号:62366055
- 批准年份:2023
- 资助金额:32 万元
- 项目类别:地区科学基金项目
多源域集成迁移学习的研究
- 批准号:61876166
- 批准年份:2018
- 资助金额:62.0 万元
- 项目类别:面上项目
混合式聚类集成算法的研究
- 批准号:61402397
- 批准年份:2014
- 资助金额:26.0 万元
- 项目类别:青年科学基金项目
相似国自然基金
{{ item.name }}
- 批准号:{{ item.ratify_no }}
- 批准年份:{{ item.approval_year }}
- 资助金额:{{ item.support_num }}
- 项目类别:{{ item.project_type }}
相似海外基金
{{
item.name }}
{{ item.translate_name }}
- 批准号:{{ item.ratify_no }}
- 财政年份:{{ item.approval_year }}
- 资助金额:{{ item.support_num }}
- 项目类别:{{ item.project_type }}