高阶非线性多智能体系统分布式资源分配算法研究及应用
项目介绍
AI项目解读
基本信息
- 批准号:61803385
- 项目类别:青年科学基金项目
- 资助金额:25.0万
- 负责人:
- 依托单位:
- 学科分类:F0301.控制理论与技术
- 结题年份:2021
- 批准年份:2018
- 项目状态:已结题
- 起止时间:2019-01-01 至2021-12-31
- 项目参与者:孙妙平; 楚晓艳; 李世玲; 王新英; 胡扬; 熊红云; 付新燃; 周志豪; 周文孝;
- 关键词:
项目摘要
High-order nonlinear multi-agent systems (MASs) appear in many fields, such as industry, agriculture and military, and there are a large number of resource allocation problems (RAPs) in those fields. The research about the RAPs of high-order nonlinear MASs is conducive to not only accelerating the development of control theory and operations research theory, but also providing the theoretical support for cyber-physical systems to automatically complete resource allocation tasks in engineering practices. The main works of this project are to investigate the RAPs of high-order nonlinear MASs with and without local feasibility constraints, respectively. We develop distributed resource allocation algorithms for those two problems, respectively, analyze the convergence of the designed algorithms, and obtain the sufficient conditions guaranteeing the convergence of the systems to the optimal solution. Moreover, we study the RAPs of high-order nonlinear MASs with general digraphs, nonsmooth cost functions and local general convex constraints, and exploit distributed algorithms with easy-to-calculate parameters and initial conditions, which can protect the privacy of agents, reduce the burden of communication and gradient sampling, and guarantee the convergence of the systems to the exact optimal solution. Besides, the obtained results are applied to the distributed generation scheduling of smart grids. Based on the dynamics of power generation systems, we propose a distributed optimal generation scheduling algorithm, which controls the power generation systems to automatically regulate their output powers to the optimal solution.
高阶非线性多智能体系统在工业、农业、军事等诸多领域有着重要应用,且在这些应用中存在大量的资源分配问题。研究高阶非线性多智能体系统资源分配问题不仅可以促进控制理论和运筹学理论的发展,且可为实际应用中信息物理系统自主完成资源分配任务提供理论依据。项目主要研究高阶非线性多智能体系统无局部约束资源分配问题和带局部约束资源分配问题。针对这两类资源分配问题分别设计分布式资源分配算法,分析算法收敛性,并得到系统收敛至最优分配的充分性条件;重点研究具有一般有向通信网络、非光滑代价函数、个体带广义凸集约束高阶非线性多智能体系统资源分配问题,并设计对初始条件要求不高、算法参数易于计算、个体隐私能有效保护、既能有效降低系统通信负担和梯度采样成本又能收敛到精确最优解的分布式资源分配算法;作为应用,研究智能电网分布式发电调度问题;基于发电系统动力学设计分布式最优发电调度算法,控制发电系统自主调整发电量趋于最优解。
结项摘要
大规模网络和复杂系统在工业、农业、军事等领域中有着广泛的应用,且在这些网络系统中存在大量的优化决策问题。随着信息物理系统和人工智能的快速发展,人们常用物理系统来完成这些大规模网络和复杂系统中的分布式优化任务。但在现有分布式优化算法研究中却很少考虑物理系统的动力学特性,使得设计的算法不能控制物理系统自主执行分布式优化决策任务。对此,本项目开展了多智能体系统分布式资源分配问题方面的研究;根据物理系统自主执行分布式优化任务机理,建立了相关问题的数学模型;利用凸优化理论、图论、现代控制理论、分布式控制技术、投影法、原始对偶法等方法,基于智能体动力学特性,设计了分布式资源分配算法,控制多智能体系统自主完成分布式资源分配任务;解决了高阶多智能体系统无局部约束资源分配问题、高阶多智能体系统带局部约束资源分配问题、具有时间/事件驱动通信及梯度采样和一般有向通信网络高阶多智能体系统非光滑资源分配问题;提出了一套针对高阶多智能体系统的分布式资源分配算法设计及分析方法;突破了复杂多智能体系统分布式控制与优化、分布式非光滑控制与优化、有向通信网络限制等关键技术;且为智能电网分布式发电管理提供了解决方案,使发电系统能自主实现最优的分布式发电调度。在项目资助下,共发表/录用了SCI期刊论文15篇,其中顶刊Automatica 3篇、IEEE Trans. 5篇、JCR一区论文12篇,发表EI会议论文6篇;培养硕士研究生2名。
项目成果
期刊论文数量(14)
专著数量(0)
科研奖励数量(0)
会议论文数量(6)
专利数量(0)
Distributed algorithm design for aggregative games of disturbed multiagent systems over weight-balanced digraphs
权重平衡有向图上受扰多智能体系统聚合博弈的分布式算法设计
- DOI:10.1002/rnc.4316
- 发表时间:2018
- 期刊:International Journal of Robust and Nonlinear Control
- 影响因子:3.9
- 作者:Deng Zhenhua;Nian Xiaohong
- 通讯作者:Nian Xiaohong
Distributed optimization of general linear multi-agent systems with external disturbance
具有外部扰动的一般线性多智能体系统的分布式优化
- DOI:10.1016/j.jfranklin.2021.05.024
- 发表时间:2021-05
- 期刊:Journal of the Franklin Institute
- 影响因子:--
- 作者:Li Shiling;Nian Xiaohong;Deng Zhenhua
- 通讯作者:Deng Zhenhua
Predefined-time distributed optimization of general linear multi-agent systems
一般线性多智能体系统的预定义时间分布式优化
- DOI:10.1016/j.ins.2021.10.060
- 发表时间:2021-11
- 期刊:Information Sciences
- 影响因子:8.1
- 作者:Li Shiling;Nian Xiaohong;Deng Zhenhua;Chen Zhao
- 通讯作者:Chen Zhao
Distributed Algorithm Design for Nonsmooth Resource Allocation Problems
非光滑资源分配问题的分布式算法设计
- DOI:10.1109/tcyb.2019.2901256
- 发表时间:2020-07
- 期刊:IEEE Transactions on Cybernetics
- 影响因子:11.8
- 作者:Deng Zhenhua;Nian Xiaohong;Hu Chen
- 通讯作者:Hu Chen
Distributed generalized Nash equilibrium seeking algorithm for nonsmooth aggregative games
非光滑聚合博弈的分布式广义纳什均衡寻求算法
- DOI:10.1016/j.automatica.2021.109794
- 发表时间:2021-07-09
- 期刊:AUTOMATICA
- 影响因子:6.4
- 作者:Deng, Zhenhua
- 通讯作者:Deng, Zhenhua
数据更新时间:{{ journalArticles.updateTime }}
{{
item.title }}
{{ item.translation_title }}
- DOI:{{ item.doi || "--"}}
- 发表时间:{{ item.publish_year || "--" }}
- 期刊:{{ item.journal_name }}
- 影响因子:{{ item.factor || "--"}}
- 作者:{{ item.authors }}
- 通讯作者:{{ item.author }}
数据更新时间:{{ journalArticles.updateTime }}
{{ item.title }}
- 作者:{{ item.authors }}
数据更新时间:{{ monograph.updateTime }}
{{ item.title }}
- 作者:{{ item.authors }}
数据更新时间:{{ sciAawards.updateTime }}
{{ item.title }}
- 作者:{{ item.authors }}
数据更新时间:{{ conferencePapers.updateTime }}
{{ item.title }}
- 作者:{{ item.authors }}
数据更新时间:{{ patent.updateTime }}
其他文献
其他文献
{{
item.title }}
{{ item.translation_title }}
- DOI:{{ item.doi || "--" }}
- 发表时间:{{ item.publish_year || "--"}}
- 期刊:{{ item.journal_name }}
- 影响因子:{{ item.factor || "--" }}
- 作者:{{ item.authors }}
- 通讯作者:{{ item.author }}
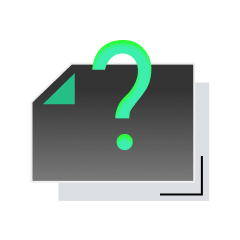
内容获取失败,请点击重试
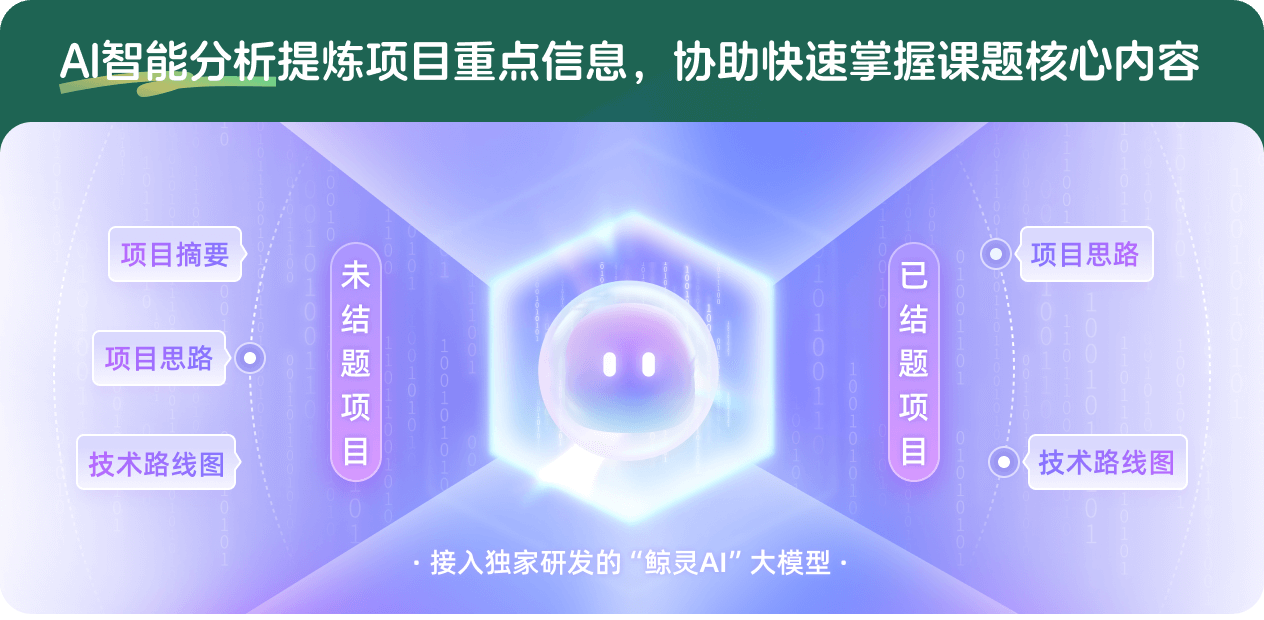
查看分析示例
此项目为已结题,我已根据课题信息分析并撰写以下内容,帮您拓宽课题思路:
AI项目摘要
AI项目思路
AI技术路线图
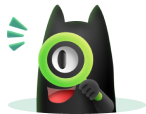
请为本次AI项目解读的内容对您的实用性打分
非常不实用
非常实用
1
2
3
4
5
6
7
8
9
10
您认为此功能如何分析更能满足您的需求,请填写您的反馈:
相似国自然基金
{{ item.name }}
- 批准号:{{ item.ratify_no }}
- 批准年份:{{ item.approval_year }}
- 资助金额:{{ item.support_num }}
- 项目类别:{{ item.project_type }}
相似海外基金
{{
item.name }}
{{ item.translate_name }}
- 批准号:{{ item.ratify_no }}
- 财政年份:{{ item.approval_year }}
- 资助金额:{{ item.support_num }}
- 项目类别:{{ item.project_type }}