基于DNA甲基化的肿瘤异质性分析及应用算法研究
项目介绍
AI项目解读
基本信息
- 批准号:61572327
- 项目类别:面上项目
- 资助金额:63.0万
- 负责人:
- 依托单位:
- 学科分类:F0213.生物信息计算与数字健康
- 结题年份:2019
- 批准年份:2015
- 项目状态:已结题
- 起止时间:2016-01-01 至2019-12-31
- 项目参与者:王军; 吴华君; 房云; 朱杰; 张乃千; 王法有; 张伟伟; 董作利; 刘晓明;
- 关键词:
项目摘要
Tumor heterogeneity is one of the causal factors for drug resistance in cancer therapy. Current researches of tumor heterogeneity are mainly based on genomic information such as gene expression, mutation, but hardly see analysis based on epigenetic information. DNA methylation is an important epigenetic mark controlling gene expression. Its alteration in cell is served as one of the fundamental hallmarks in cancer. The present project aims to study inter heterogeneity of tumor cells based on DNA methylation data using various bioinformatics tools, and develop applied algorithms. The detailed contents include: 1) construction of the multi-subclone mixture model of tumor tissues based on DNA methylation data including 450K array and high-throughput bisulfite sequencing. Then, we develop deconvolution algorithms based on matrix decomposition and optimization methods to infer the composition of each subclone and its specific methylation pattern; 2) Using machine-learning tools such as regression, clustering and correlation analysis, study the relationships between subclone structure of tumor tissue with sample survival and different genomic information, in an effort to elucidate its function in tumorigenesis and clinical treatment; 3) Taken lung, breast and colorectal cancers as examples, infer the evolutionary history of tumor subclone structure based on DNA methylation data. Our research could further elucidate the epigenetic mechanism of tumorigenesis, and help to design better molecular target therapies for personalized medicine.
肿瘤异质性是癌症放化疗和药物靶向治疗时产生机体抗药性的重要原因。目前,针对肿瘤异质性的研究大多基于基因突变、表达等遗传信息,从表观遗传层面上的研究尚属少见。DNA甲基化是一类重要的表观遗传修饰,其表达异常是肿瘤与正常细胞最本质的区别之一。本项目将从DNA甲基化的角度对肿瘤异质性开展生物信息学研究,开发应用算法。内容包括:(1) 以DNA甲基化的450K芯片和重亚硫酸盐测序数据为基础,建立肿瘤组织的多亚群混合模型,开发基于矩阵分解和最优化的反卷积算法,得到不同亚群的组成和特异甲基化模式;(2) 利用回归、聚类、关联分析等机器学习方法,研究肿瘤亚群结构与样本生存率、基因组信息之间的关系,探索其在肿瘤发育和临床治疗中的作用;(3) 以肺癌、乳腺癌、结直肠癌等多发癌为例,建立基于DNA甲基化的肿瘤亚群演化模型。本项目的研究对进一步揭示肿瘤发育的表观遗传机理,设计合理的分子靶向药物具有指导意义。
结项摘要
癌症之所以难以诊断和治疗,主要原因在于肿瘤组织内部高度的异质性,即除少数癌症类型外,恶性肿瘤组织往往由不同的细胞亚群落 (sub-population或sub-clone) 组成。不同的肿瘤亚群落细胞具有不同的细胞形态、基因表达谱、代谢水平、增殖和转移能力。这些亚群落与侵润的免疫细胞和周边的基质细胞、正常细胞等相互作用,构成复杂的肿瘤微环境。普遍认为,肿瘤异质性是肿瘤组织中亚群演化的结果,其与肿瘤的生长、转移、复发、耐药性的产生等临床指标密切相关。.本项目拟从肿瘤组织的甲基化数据出发,以信息理论、机器学习方法为工具,研究肿瘤细胞在表观遗传层面上的异质性。通过建立肿瘤组织甲基化组的混合概率模型和矩阵分解模型,求解出不同亚群在混合组织中所占的比例和其特异性甲基化区域,开发相关应用算法。项目开展期间,我们开发了基于DNA甲基化450K芯片数据的肿瘤纯度估计方法InfiniumPurify,利用广义最小二乘方法提出了考虑肿瘤纯度的差异甲基化分析方法InfiniumDMC,利用最大似然估计方法提出了考虑纯度信息的肿瘤样本聚类方法InfiniumClust。针对肿瘤异质性分析问题,我们提出了基于部分参考信息的肿瘤异质性分解算法PREDE(Partial REference Deconvolution algorithm),并研究了其在肿瘤免疫学研究中的应用,发现了一些免疫细胞类型和癌症细胞类型,其在肿瘤组织中的组成成分与肿瘤样本的生存率显著相关。.在本项目的资助下,已发表SCI论文9篇,其中Genome Biology (IF=13.214) 两篇,Bioinformatics (IF=5.481) 一篇,PLoS Computational Biology (IF=3.955) 一篇等,从数量和质量上均超额完成预定目标。 在人才培养指标上,在本项目的资助下,课题组共毕业博士生3名,研究生5名,顺利完成了预定目标。.准确估计肿瘤异质性的程度,包括肿瘤组织中所包含的细胞亚群、各细胞亚群的分子标签及其在肿瘤组织中所占的比例,对于理解肿瘤发育和转移的分子机理,并进而设计有效的临床治疗方案具有重要的意义。
项目成果
期刊论文数量(9)
专著数量(0)
科研奖励数量(0)
会议论文数量(0)
专利数量(0)
Estimating and accounting for tumor purity in the analysis of DNA methylation data from cancer studies.
在分析癌症研究中的 DNA 甲基化数据时估计和解释肿瘤纯度
- DOI:10.1186/s13059-016-1143-5
- 发表时间:2017-01-25
- 期刊:Genome biology
- 影响因子:12.3
- 作者:Zheng X;Zhang N;Wu HJ;Wu H
- 通讯作者:Wu H
From structure to function, how bioinformatics help to reveal functions of our genomes.
从结构到功能,生物信息学如何帮助揭示我们基因组的功能
- DOI:10.1186/s13059-017-1320-1
- 发表时间:2017-09-26
- 期刊:Genome biology
- 影响因子:12.3
- 作者:Zheng X;Zhong S
- 通讯作者:Zhong S
Accounting for tumor purity improves cancer subtype classification from DNA methylation data
考虑肿瘤纯度可根据 DNA 甲基化数据改进癌症亚型分类
- DOI:10.1093/bioinformatics/btx303
- 发表时间:2017-09-01
- 期刊:BIOINFORMATICS
- 影响因子:5.8
- 作者:Zhang, Weiwei;Feng, Hao;Zheng, Xiaoqi
- 通讯作者:Zheng, Xiaoqi
A systematic study on drug-response associated genes using baseline gene expressions of the Cancer Cell Line Encyclopedia.
使用癌细胞系百科全书的基线基因表达对药物反应相关基因进行系统研究
- DOI:10.1038/srep22811
- 发表时间:2016-03-10
- 期刊:Scientific reports
- 影响因子:4.6
- 作者:Liu X;Yang J;Zhang Y;Fang Y;Wang F;Wang J;Zheng X;Yang J
- 通讯作者:Yang J
Predicting Anticancer Drug Responses Using a Dual-Layer Integrated Cell Line-Drug Network Model.
使用双层集成细胞系-药物网络模型预测抗癌药物反应
- DOI:10.1371/journal.pcbi.1004498
- 发表时间:2015
- 期刊:PLoS computational biology
- 影响因子:4.3
- 作者:Zhang N;Wang H;Fang Y;Wang J;Zheng X;Liu XS
- 通讯作者:Liu XS
数据更新时间:{{ journalArticles.updateTime }}
{{
item.title }}
{{ item.translation_title }}
- DOI:{{ item.doi || "--"}}
- 发表时间:{{ item.publish_year || "--" }}
- 期刊:{{ item.journal_name }}
- 影响因子:{{ item.factor || "--"}}
- 作者:{{ item.authors }}
- 通讯作者:{{ item.author }}
数据更新时间:{{ journalArticles.updateTime }}
{{ item.title }}
- 作者:{{ item.authors }}
数据更新时间:{{ monograph.updateTime }}
{{ item.title }}
- 作者:{{ item.authors }}
数据更新时间:{{ sciAawards.updateTime }}
{{ item.title }}
- 作者:{{ item.authors }}
数据更新时间:{{ conferencePapers.updateTime }}
{{ item.title }}
- 作者:{{ item.authors }}
数据更新时间:{{ patent.updateTime }}
其他文献
拟南芥基因转录调控关系识别研究进展
- DOI:--
- 发表时间:2013
- 期刊:广东农业科学
- 影响因子:--
- 作者:秦玉芳;郑小琪
- 通讯作者:郑小琪
其他文献
{{
item.title }}
{{ item.translation_title }}
- DOI:{{ item.doi || "--" }}
- 发表时间:{{ item.publish_year || "--"}}
- 期刊:{{ item.journal_name }}
- 影响因子:{{ item.factor || "--" }}
- 作者:{{ item.authors }}
- 通讯作者:{{ item.author }}
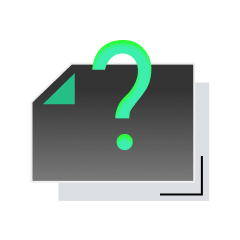
内容获取失败,请点击重试
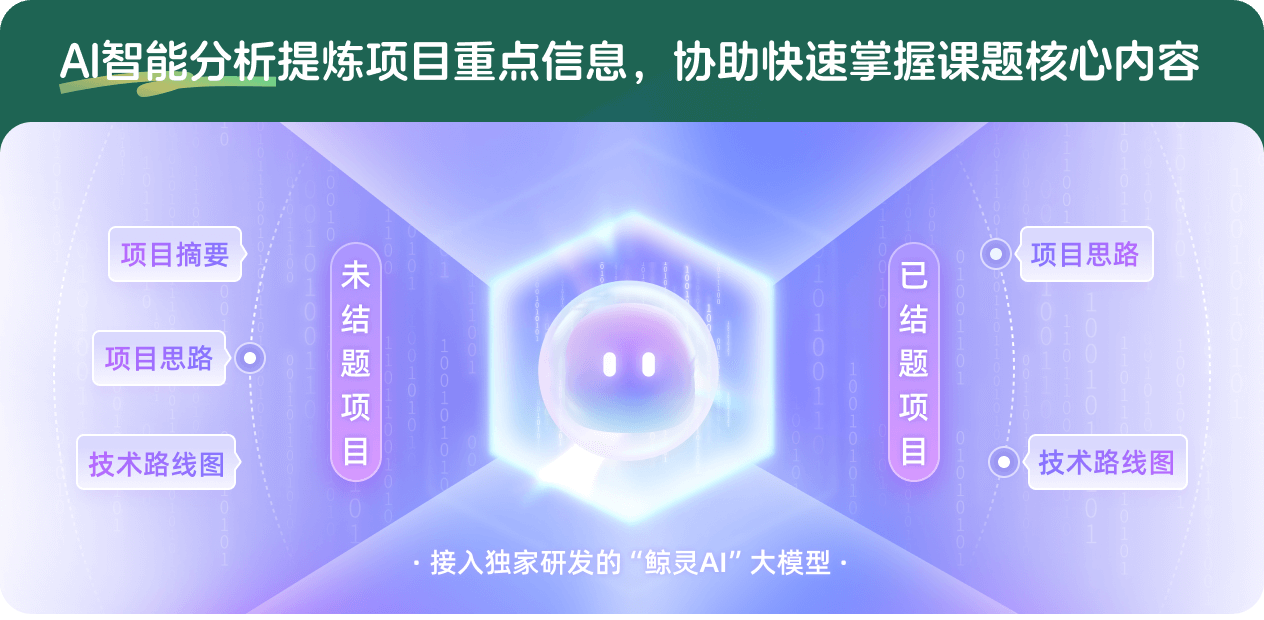
查看分析示例
此项目为已结题,我已根据课题信息分析并撰写以下内容,帮您拓宽课题思路:
AI项目摘要
AI项目思路
AI技术路线图
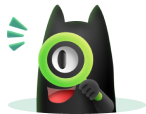
请为本次AI项目解读的内容对您的实用性打分
非常不实用
非常实用
1
2
3
4
5
6
7
8
9
10
您认为此功能如何分析更能满足您的需求,请填写您的反馈:
郑小琪的其他基金
融合单细胞转录组数据的肿瘤异质性分析方法研究
- 批准号:
- 批准年份:2019
- 资助金额:60 万元
- 项目类别:面上项目
拟南芥基因转录调控关系的识别方法研究
- 批准号:31100953
- 批准年份:2011
- 资助金额:20.0 万元
- 项目类别:青年科学基金项目
相似国自然基金
{{ item.name }}
- 批准号:{{ item.ratify_no }}
- 批准年份:{{ item.approval_year }}
- 资助金额:{{ item.support_num }}
- 项目类别:{{ item.project_type }}
相似海外基金
{{
item.name }}
{{ item.translate_name }}
- 批准号:{{ item.ratify_no }}
- 财政年份:{{ item.approval_year }}
- 资助金额:{{ item.support_num }}
- 项目类别:{{ item.project_type }}