面向肿瘤异质性的亚群特异性驱动基因计算表征及预测研究
项目介绍
AI项目解读
基本信息
- 批准号:61901322
- 项目类别:青年科学基金项目
- 资助金额:24.5万
- 负责人:
- 依托单位:
- 学科分类:F0124.生物电子学与生物信息处理
- 结题年份:2022
- 批准年份:2019
- 项目状态:已结题
- 起止时间:2020-01-01 至2022-12-31
- 项目参与者:--
- 关键词:
项目摘要
Subgroup-specific driver genes are critical for revealing the pathogenesis of heterogeneous cancers and for developing the precision diagnosis and treatment of cancers. Although current studies have made considerable progress in the prediction of common driver genes from multiple samples, the prediction of subgroup-specific driver genes is still limited in the ideal situation that subgroup categories are already known. When the subgroup category is unknown, the current studies cannot identify the interacting driver genes, i.e., the driver genes which are abnormally affected by its interacting genes. Furthermore, for multi-subgroup coexisting driver genes, the current studies do not yet have the predictive ability. Therefore, to address the issue that the subgroup categories are unknown, this project aims to explore the subgroup-specific inducing representation strategy for tumor heterogeneous data, and build the vectorization representation to infer the subgroup affiliation of driver genes. Based on the representation results above, to predict the interacting driver genes, we develop the mechanism of the correlation simulation for the vectorization representation points, and then enhance the predictive ability of the interacting driver genes. For the recognition of multi-subgroup coexisting driver genes, we establish an outlier detection method for the multimodal distribution in the representation space, and achieve the synchronous prediction of both single-subgroup specific/multi-subgroup coexisting driver genes. This project will provide new ideas for subgroup-specific driver gene analysis, and support the precision diagnosis and treatment in cancer clinical trials.
亚群特异性驱动基因对揭示异质性癌症发病机理及发展癌症精准诊疗极为关键。虽然现有研究在多样本的共有驱动基因预测方面取得了较多进展,但对亚群特异性驱动基因的预测,目前仍局限于亚群类别已知的理想情况。在亚群类别未知时,现有研究无法识别其中的关联性驱动基因,即受相互作用基因影响而异常的驱动基因。此外,对于多亚群共存驱动基因,现有研究尚不具备预测能力。为此,针对亚群类别未知问题,本项目拟探索面向肿瘤异质性数据的亚群特异性指引表征策略,通过向量化表征推断出驱动基因的亚群从属关系。在此基础上,针对关联性驱动基因的预测,发展表征向量点关联性模拟机制,进而强化对关联性驱动基因的预测能力。针对多亚群共存驱动基因的识别,构建表征空间多峰分布的离群点判定方法,实现单亚群特异性/多亚群共存驱动基因的同步化预测。本项目将为亚群特异性驱动基因分析提供新思路,为癌症临床的精准化诊断治疗提供支持。
结项摘要
亚群特异性驱动基因不仅可指示癌症样本在发病机理层面的分歧,还能有效反映癌症病灶在临床治疗中的耐药性差异。然而,前期研究对亚群特异性驱动基因的预测,目前仍局限于亚群类别已知的理想情况。在亚群类别未知时,前期研究不仅难以识别亚群特异性的关联性驱动基因,还不具备对多类型变异共存的亚群特异性驱动基因的预测能力。对此,本研究开展了面向肿瘤异质性的亚群特异性驱动基因计算表征及预测研究,取得进展:1)亚群特异性驱动基因的向量空间表征预测模型方面,针对驱动基因的亚群从属关系未知问题,通过亚群特异性指引表征策略推断驱动基因亚群从属,开发了亚群指示子空间表征的亚群特异性驱动基因推断算法软件,实现了肿瘤内异质性的单细胞变异数据聚类参数表征与亚群挖掘。2)基因互作关系对局部基因驱动关联性模拟预测方面,针对亚群特异驱动基因局部样本关联性,研究了相互作用基因表征关联机制,完成了异质性癌症局部样本的驱动基因相互作用关联建模与预测,开展了基因互作关联模块的空间表征概念学习癌症驱动基因识别,提升了关联性驱动基因的预测性能。3)多类型变异共存亚群特异性驱动基因判别方法方面,针对多类型变异共存驱动基因漏检,实现了共存多类型变异整合动态知识图谱空间亚群特异驱动识别,开发了面向癌症良恶性超声影像的临床报告知识图谱张量化建模,发展了临床数据缺失场景下的癌症知识图谱诊断与抗癌药物推理,促进了多类型亚群特异性驱动基因的同步预测。本项目研究成果发表SCI期刊论文9篇(含中科院分区顶刊2篇),EI会议论文4篇(含CCF A类与B类),专利申请3项并授权1项。依托项目协助培养硕士生4名。国际学术会议特邀报告1次、分组报告4次,在国内学术会议做特邀报告3次。项目负责人以第一完成人荣获西安市第十八届自然科学优秀学术论文奖,获SEBM杰出审稿人奖。研究成果不仅为揭示异质性癌症发病机理提供分析工具,还具有癌症精准医疗的临床应用价值。
项目成果
期刊论文数量(9)
专著数量(0)
科研奖励数量(2)
会议论文数量(4)
专利数量(4)
Editorial: Unsupervised Learning Models for Unlabeled Genomic, Transcriptomic & Proteomic Data.
社论:未标记基因组、转录组的无监督学习模型
- DOI:10.3389/fgene.2021.781698
- 发表时间:2021
- 期刊:Frontiers in genetics
- 影响因子:3.7
- 作者:Xi J;Yu Z
- 通讯作者:Yu Z
Simultaneous Segmentation of Fetal Hearts and Lungs for Medical Ultrasound Images via an Efficient Multi-scale Model Integrated With Attention Mechanism
通过集成注意力机制的高效多尺度模型同时分割医学超声图像的胎儿心脏和肺部
- DOI:10.1177/01617346211042526
- 发表时间:2021-09
- 期刊:Ultrasonic Imaging
- 影响因子:2.3
- 作者:Xi Jianing;Chen Jiangang;Wang Zhao;Ta Dean;Lu Bing;Deng Xuedong;Li Xuelong;Huang Qinghua
- 通讯作者:Huang Qinghua
Cancer omic data based explainable AI drug recommendation inference: A traceability perspective for explainability
基于癌症组学数据的可解释人工智能药物推荐推断:可解释性的可追溯性视角
- DOI:10.1016/j.bspc.2022.104144
- 发表时间:2023-01
- 期刊:Biomedical Signal Processing and Control
- 影响因子:5.1
- 作者:Xi Jianing;Wang Dan;Yang Xuebing;Zhang Wensheng;Huang Qinghua
- 通讯作者:Huang Qinghua
An omics-to-omics joint knowledge association subtensor model for radiogenomics cross-modal modules from genomics and ultrasonic images of breast cancers
用于乳腺癌基因组学和超声图像的放射基因组学跨模态模块的组学到组学联合知识关联子张量模型
- DOI:10.1016/j.compbiomed.2023.106672
- 发表时间:2023-02-16
- 期刊:COMPUTERS IN BIOLOGY AND MEDICINE
- 影响因子:7.7
- 作者:Xi,Jianing;Sun,Donghui;Huang,Qinghua
- 通讯作者:Huang,Qinghua
Integrating multi-type aberrations from DNA and RNA through dynamic mapping gene space for subtype-specific breast cancer driver discovery.
通过动态映射基因空间整合 DNA 和 RNA 的多类型畸变,以发现亚型特异性乳腺癌驱动因素
- DOI:10.7717/peerj.14843
- 发表时间:2023
- 期刊:PEERJ
- 影响因子:2.7
- 作者:Xi, Jianing;Deng, Zhen;Liu, Yang;Wang, Qian;Shi, Wen
- 通讯作者:Shi, Wen
数据更新时间:{{ journalArticles.updateTime }}
{{
item.title }}
{{ item.translation_title }}
- DOI:{{ item.doi || "--"}}
- 发表时间:{{ item.publish_year || "--" }}
- 期刊:{{ item.journal_name }}
- 影响因子:{{ item.factor || "--"}}
- 作者:{{ item.authors }}
- 通讯作者:{{ item.author }}
数据更新时间:{{ journalArticles.updateTime }}
{{ item.title }}
- 作者:{{ item.authors }}
数据更新时间:{{ monograph.updateTime }}
{{ item.title }}
- 作者:{{ item.authors }}
数据更新时间:{{ sciAawards.updateTime }}
{{ item.title }}
- 作者:{{ item.authors }}
数据更新时间:{{ conferencePapers.updateTime }}
{{ item.title }}
- 作者:{{ item.authors }}
数据更新时间:{{ patent.updateTime }}
其他文献
基于多元方差分析的成对肿瘤SNP array数据分段算法
- DOI:--
- 发表时间:2014
- 期刊:科学通报
- 影响因子:--
- 作者:习佳宁;刘元宁;王明会;冯焕清;李骜
- 通讯作者:李骜
其他文献
{{
item.title }}
{{ item.translation_title }}
- DOI:{{ item.doi || "--" }}
- 发表时间:{{ item.publish_year || "--"}}
- 期刊:{{ item.journal_name }}
- 影响因子:{{ item.factor || "--" }}
- 作者:{{ item.authors }}
- 通讯作者:{{ item.author }}
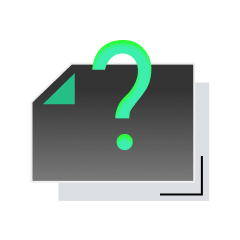
内容获取失败,请点击重试
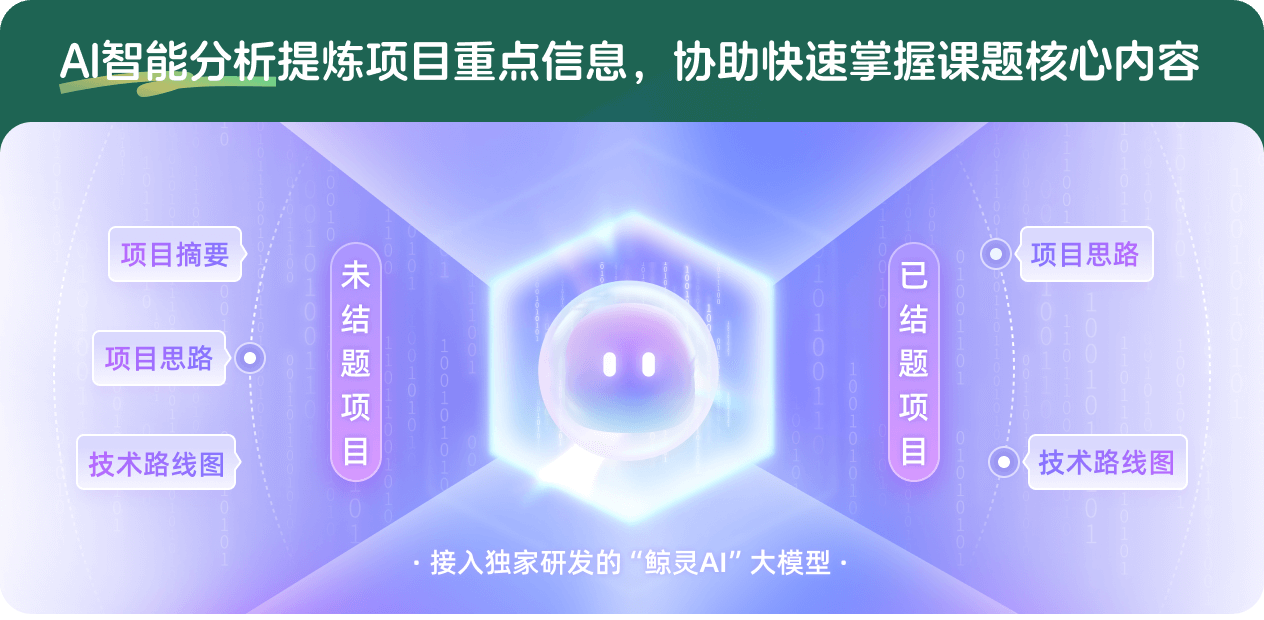
查看分析示例
此项目为已结题,我已根据课题信息分析并撰写以下内容,帮您拓宽课题思路:
AI项目摘要
AI项目思路
AI技术路线图
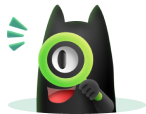
请为本次AI项目解读的内容对您的实用性打分
非常不实用
非常实用
1
2
3
4
5
6
7
8
9
10
您认为此功能如何分析更能满足您的需求,请填写您的反馈:
相似国自然基金
{{ item.name }}
- 批准号:{{ item.ratify_no }}
- 批准年份:{{ item.approval_year }}
- 资助金额:{{ item.support_num }}
- 项目类别:{{ item.project_type }}
相似海外基金
{{
item.name }}
{{ item.translate_name }}
- 批准号:{{ item.ratify_no }}
- 财政年份:{{ item.approval_year }}
- 资助金额:{{ item.support_num }}
- 项目类别:{{ item.project_type }}