基于深度迁移学习的微拟球藻蛋白质亚细胞定位预测方法研究
项目介绍
AI项目解读
基本信息
- 批准号:31900071
- 项目类别:青年科学基金项目
- 资助金额:24.0万
- 负责人:
- 依托单位:
- 学科分类:C0105.微生物学新技术与新方法
- 结题年份:2022
- 批准年份:2019
- 项目状态:已结题
- 起止时间:2020-01-01 至2022-12-31
- 项目参与者:--
- 关键词:
项目摘要
Nannochloropsis oceanica has become a model industrial oleaginous alga. For the vast number of proteins encoded in its genome, determination of their subcellular localization (SCL) is of great significance for not just understanding the carbon-fixation and oil production mechanisms but also molecular breeding for superior microalgae. Conventional experimental approaches for SCL are based on fluorescence fusion protein, and suffer from the drawback of time-consuming and low-throughput operation; as a result, there were no sufficient training samples for establishing organism-specific SCL prediction tools. On the other hand, existing SCL prediction tools for microalgae are of low accuracy when applied in Nannochloropsis spp., as they are all trained using phylogenetically distant species. To tackle this challenge, this project proposed to design SCL prediction tools specifically for Nannochloropsis based on deep transfer neural network and high-throughput experimental localization. In N. oceanica, firstly, we will construct a plasmid library for the proteome, which is tagged with fluorescence protein, and establish high-throughput screening technique for detecting and discriminating particular SCL signals. Secondly, we will develop and optimize organism-specific SCL prediction tools, based on deep transfer learning method and by utilizing both the aforementioned high-throughput localization data and also from cross-species SCL datasets. Finally, by further validating and comparing the results of SCL, we hope to unravel previously unknown signaling peptide or regulatory mechanisms of SCL in N. oceanica. These efforts are expected to establish a high-accuracy SCL prediction tool for Nannochloropsis spp., and thus facilitate the mechanistic dissection of key traits and the precise delivery of foreign proteins for trait engineering, both of which key to the molecular breeding of superior industrial oleaginous microalgae.
海洋微拟球藻是工业产油微藻的模式物种之一,其蛋白质亚细胞定位对于固碳产油机制的理解和工业微藻分子育种具有重要意义。传统的荧光蛋白融合蛋白实验定位法耗时费力且通量极低,无法获取足够的训练样本以建立物种特异性的定位预测工具。而现有的微藻蛋白亚细胞定位预测工具都是基于亲缘关系较远的物种,在微拟球藻中准确率很低。因此,本项目拟基于深度迁移神经网络和高通量实验定位法,开发大幅提高该预测准确率的计算工具。在海洋微拟球藻中,首先,构建带荧光标记的蛋白质组质粒文库,并建立针对亚细胞定位的高通量筛选技术。然后,基于深度迁移学习方法,并利用高通量实验定位数据和跨物种的亚细胞定位数据集,建立并优化定位预测模型。最后,通过对亚细胞定位的深度验证和比较,挖掘其调控序列与机制。本项目建立的蛋白亚细胞定位预测方法,将促进工业产油微藻中关键表型的机制研究和基于外源蛋白精确定点表达的代谢工程,从而服务于工业微藻分子育种。
结项摘要
微拟球藻是重要的工业产油微藻,可以用来大规模生产甘油三酯(TAG)和二十碳五烯酸(EPA)。微拟球藻的油脂合成代谢途径较为复杂,需要多种细胞器的参与,高效、准确地确定微拟球藻重要蛋白质的亚细胞定位对固碳产油机制的理解和分子育种具有重要意义。由于蛋白质亚细胞定位的实验验证耗时、费力且通量低,目前微拟球藻中大多数蛋白质的亚细胞定位仍依赖于机器学习模型的预测,但现有预测工具对于类似微拟球藻的非模式物种准确率较低。本项目以微拟球藻为模式体系,验证了基于深度迁移学习方法构建物种专用的蛋白质亚细胞定位预测模型的可行性。首先,本项目全面收集整理了微拟球藻的多组学数据,建立了微拟球藻设计与合成数据库(NanDeSyn),并构建了基于序列扩增的训练数据集、迁移数据集和测试数据集。然后,本项目基于时间卷积神经网络与层次注意力机制建立了真核生物蛋白质亚细胞定位预测工具(PSCLSCA)。在此基础上,利用注意力机制回溯重要的肽段,验证了模型的有效性,并为预测结果提供了一种解释;另外,基于深度神经网络的随机失活机制建立了预测结果置信度与不确定性指标的量化方法。PSCLSCA的结构简单、预测准确率高,并且能够给出预测结果的不确定性估计。应用迁移数据集后,PSCLSCA的准确度显著提高,证明了迁移方法的有效性。再次,本项目验证了高通量实验验证方案主要环节的可行性。最后,本项目通过跨物种比较挖掘到微拟球藻中若干具有特殊亚细胞定位的蛋白质,并结合多组学分析,揭示了脂筏、高丝氨酸O-乙酰转移酶、碳酸酐酶、磷酸烯醇丙酮酸羧化激酶等对于微拟球藻固碳产油的潜在重要功能。本项目设计的预测工具已经借助NanDeSyn数据库网站提供给广大研究群体,成为工业产油微藻中关键表型的机制解析和工业微藻分子育种的重要支撑工具。
项目成果
期刊论文数量(1)
专著数量(0)
科研奖励数量(0)
会议论文数量(0)
专利数量(0)
The NanDeSyn database for Nannochloropsis systems and synthetic biology
微拟球藻系统和合成生物学的 NanDeSyn 数据库
- DOI:10.1111/tpj.15025
- 发表时间:2020-11-27
- 期刊:PLANT JOURNAL
- 影响因子:7.2
- 作者:Gong, Yanhai;Kang, Nam K.;Xu, Jian
- 通讯作者:Xu, Jian
数据更新时间:{{ journalArticles.updateTime }}
{{
item.title }}
{{ item.translation_title }}
- DOI:{{ item.doi || "--"}}
- 发表时间:{{ item.publish_year || "--" }}
- 期刊:{{ item.journal_name }}
- 影响因子:{{ item.factor || "--"}}
- 作者:{{ item.authors }}
- 通讯作者:{{ item.author }}
数据更新时间:{{ journalArticles.updateTime }}
{{ item.title }}
- 作者:{{ item.authors }}
数据更新时间:{{ monograph.updateTime }}
{{ item.title }}
- 作者:{{ item.authors }}
数据更新时间:{{ sciAawards.updateTime }}
{{ item.title }}
- 作者:{{ item.authors }}
数据更新时间:{{ conferencePapers.updateTime }}
{{ item.title }}
- 作者:{{ item.authors }}
数据更新时间:{{ patent.updateTime }}
其他文献
其他文献
{{
item.title }}
{{ item.translation_title }}
- DOI:{{ item.doi || "--" }}
- 发表时间:{{ item.publish_year || "--"}}
- 期刊:{{ item.journal_name }}
- 影响因子:{{ item.factor || "--" }}
- 作者:{{ item.authors }}
- 通讯作者:{{ item.author }}
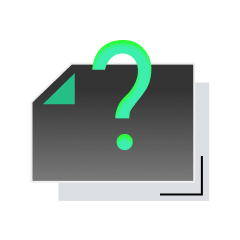
内容获取失败,请点击重试
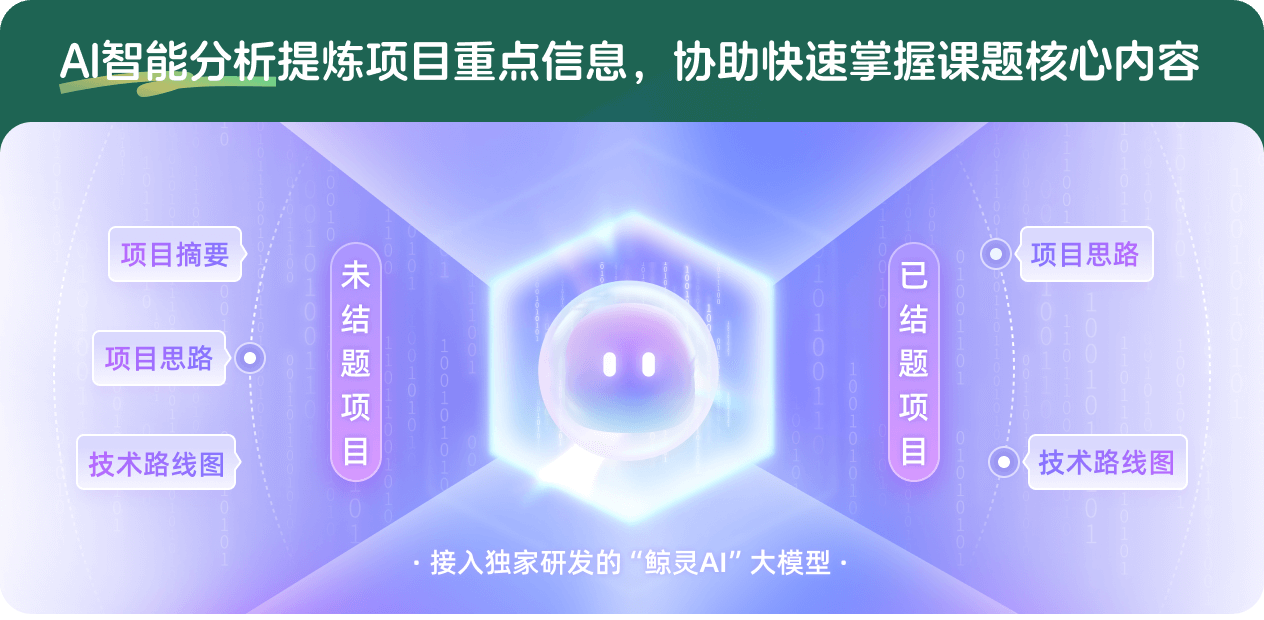
查看分析示例
此项目为已结题,我已根据课题信息分析并撰写以下内容,帮您拓宽课题思路:
AI项目摘要
AI项目思路
AI技术路线图
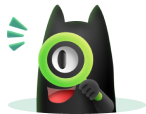
请为本次AI项目解读的内容对您的实用性打分
非常不实用
非常实用
1
2
3
4
5
6
7
8
9
10
您认为此功能如何分析更能满足您的需求,请填写您的反馈:
公衍海的其他基金
宏基因组生物标志物驱动的菌群单细胞基因组-表型组关联分析方法研究
- 批准号:32370097
- 批准年份:2023
- 资助金额:50 万元
- 项目类别:面上项目
相似国自然基金
{{ item.name }}
- 批准号:{{ item.ratify_no }}
- 批准年份:{{ item.approval_year }}
- 资助金额:{{ item.support_num }}
- 项目类别:{{ item.project_type }}
相似海外基金
{{
item.name }}
{{ item.translate_name }}
- 批准号:{{ item.ratify_no }}
- 财政年份:{{ item.approval_year }}
- 资助金额:{{ item.support_num }}
- 项目类别:{{ item.project_type }}