基于知识图谱的社交媒体大数据主题发现与趋势预测关键问题研究
项目介绍
AI项目解读
基本信息
- 批准号:61772366
- 项目类别:面上项目
- 资助金额:63.0万
- 负责人:
- 依托单位:
- 学科分类:F0211.信息检索与社会计算
- 结题年份:2021
- 批准年份:2017
- 项目状态:已结题
- 起止时间:2018-01-01 至2021-12-31
- 项目参与者:李江峰; 向阳; 刘春梅; 鄂世嘉; 袁书寒; 吕东东; 贾圣宾; 赵振岐; 荣光辉;
- 关键词:
项目摘要
Social media big data contains abundant information resources, which enables people more efficiently to discover deep-level topics and forecast their future evolution trend. However, social media big data is usually multi-mode, high degree of fragmentation, strong correlation and high noise. And these characteristics make the existing methods of discovering and forecasting topics have great disadvantages in usability, accuracy, timeliness and self-adaptability. Based on the above facts, we use the research mentality of “theoretical analysis→numerical calculation→application validatation”. Firstly, we automatically construct and optimize the large-scale probability knowledge graph, and use it as the cornerstone of topic discovery and trend forecasting. Secondly, we make full use of the merits of multi-order tensor representation, and propose theories and methods of the integrated tensor representation for social media big data. On the basis of which, the temporal hierarchical feature model is constructed to model multi-granularity features, and then the deep features of social media big data are effectively extracted by means of the deep learning technology. Thereby, using the implied data semantic information and semantic reasoning ability of our constructed knowledge graph, we achieve the discovery of deep-level topics and accurate prediction of their evolution trends. We expect that our research findings will play an important role on the domains of social public security, E-commerce, public health care, and internet deep information services, etc.
社交媒体大数据中蕴含着丰富的信息资源,这使得人们能够更有效地发现深层主题以及预测其未来的发展趋势。然而由于社交媒体大数据所具有的多模态、碎片化程度高、强关联和高噪声等特性,使得现有方法在可用性、准确性、时效性与自适应能力等方面存在较大的不足。为此,本项目拟以“理论分析→数值计算→应用验证”为思路展开研究,首先自动化构建与优化大规模概率知识图谱,并将其作为主题发现与趋势预测的语义基石;其次,充分利用多阶张量表示的优点,提出社交媒体大数据的一体化张量表示理论及方法,在此基础上,构建时序层次特征模型进行多粒度特征建模,并借助深度学习技术有效提取社交媒体大数据的深层特征;从而利用概率知识图谱所隐含的数据语义信息以及强大的语义推理能力来实现深层次主题的发现与发展趋势的精准预测。我们预计,本项目的研究成果将在社会公共安全、电子商务、民众医疗健康和互联网深度信息服务等领域发挥重大作用。
结项摘要
随着日积月累,社交媒体大数据,蕴含着丰富的社会信息,其中包含着大量重要社会事件线索信息的网络映射,而这些网络映射信息通常看似杂乱无章的。深度分析和挖掘社交媒体大数据,快速精准地发现其中所隐含的深层次主题,进而在现有被发现主题的基础上,有效预测社会事件未来发展的态势,是促进国家社会治安稳定和知识经济可持续发展的重要手段。然而我们发现,社交媒体大数据有着许多自身的特点,包括多模态、时效性强、内容简短、碎片化程度高以及关联性强等。这些特点,使得现有方法在深层次主题发现和趋势预测方面面临着一些新的挑战。为了有效解决上述问题和挑战,本项目以“理论分析数值计算应用验证”三个层次展开,首先对大规模知识图谱的构建过程进行深度优化,并将知识图谱作为社交媒体大数据主题发现、演化与趋势预测的语义基石;其次,针对社交媒体大数据的自身特点,充分利用多阶张量表示的优点,提出社交媒体大数据的一体化张量表示理论;在此基础上,构建时序层次特征模型进行多粒度特征建模,并借助深度学习技术有效提取社交媒体大数据的深层特征,从而利用知识图谱所隐含的数据语义信息以及强大的语义推理能力来实现社交媒体大数据深层次主题的发现和发展趋势的精准预测。本项目的研究成果在社会公共安全、民众医疗健康、互联网深度信息服务和电子商务等领域能够发挥积极作用。
项目成果
期刊论文数量(19)
专著数量(0)
科研奖励数量(1)
会议论文数量(2)
专利数量(9)
A multi-criteria detection scheme of collusive fraud organization for reputation aggregation in social networks
社交网络中声誉聚合的共谋欺诈组织多标准检测方案
- DOI:10.1016/j.future.2017.09.027
- 发表时间:2018-02
- 期刊:Future Generation Computer Systems-The International Journal of eScience
- 影响因子:7.5
- 作者:Zhang Bo;Zhang Qian;Huang Zhenhua;Li Meizi;Li Luqun
- 通讯作者:Li Luqun
A Novel Group Recommendation Model With Two-Stage Deep Learning
一种新颖的两阶段深度学习群体推荐模型
- DOI:10.1109/tsmc.2021.3131349
- 发表时间:2021-12-08
- 期刊:IEEE TRANSACTIONS ON SYSTEMS MAN CYBERNETICS-SYSTEMS
- 影响因子:8.7
- 作者:Huang, Zhenhua;Liu, Yajun;Chen, Yunwen
- 通讯作者:Chen, Yunwen
A novel method for detecting new overlapping community in complex evolving networks
一种在复杂演化网络中检测新重叠社区的新方法
- DOI:10.1109/tsmc.2017.2779138
- 发表时间:2019
- 期刊:IEEE Transactions on Systems, Man, and Cybernetics: Systems
- 影响因子:--
- 作者:Jiujun Cheng;Xiao Wu;Mengchu Zhou;Shangce Gao;Zhenhua Huang;Cong Liu
- 通讯作者:Cong Liu
Multimodal Representation Learning for Recommendation in Internet of Things
物联网推荐的多模态表示学习
- DOI:10.1109/jiot.2019.2940709
- 发表时间:2019-12-01
- 期刊:IEEE INTERNET OF THINGS JOURNAL
- 影响因子:10.6
- 作者:Huang, Zhenhua;Xu, Xin;Wang, Cheng
- 通讯作者:Wang, Cheng
Materialized Views Selection in Distributed Networks
分布式网络中的物化视图选择
- DOI:10.3966/160792642019032002011
- 发表时间:2019-03
- 期刊:Journal of Internet Technology
- 影响因子:1.6
- 作者:Huang Zhenhua;Zhao Zhenqi;Cheng Jiujun
- 通讯作者:Cheng Jiujun
数据更新时间:{{ journalArticles.updateTime }}
{{
item.title }}
{{ item.translation_title }}
- DOI:{{ item.doi || "--"}}
- 发表时间:{{ item.publish_year || "--" }}
- 期刊:{{ item.journal_name }}
- 影响因子:{{ item.factor || "--"}}
- 作者:{{ item.authors }}
- 通讯作者:{{ item.author }}
数据更新时间:{{ journalArticles.updateTime }}
{{ item.title }}
- 作者:{{ item.authors }}
数据更新时间:{{ monograph.updateTime }}
{{ item.title }}
- 作者:{{ item.authors }}
数据更新时间:{{ sciAawards.updateTime }}
{{ item.title }}
- 作者:{{ item.authors }}
数据更新时间:{{ conferencePapers.updateTime }}
{{ item.title }}
- 作者:{{ item.authors }}
数据更新时间:{{ patent.updateTime }}
其他文献
云环境下top-n 推荐算法
- DOI:--
- 发表时间:2015
- 期刊:电子学报
- 影响因子:--
- 作者:黄震华
- 通讯作者:黄震华
EAPSC:有效聚类skyline对象集方法
- DOI:--
- 发表时间:--
- 期刊:模式识别与人工智能
- 影响因子:--
- 作者:黄震华;向阳;林琛
- 通讯作者:林琛
一种基于语义的决策服务协作自组织方法
- DOI:--
- 发表时间:2013
- 期刊:控制与决策
- 影响因子:--
- 作者:张波;向阳;黄震华
- 通讯作者:黄震华
span style=font-family:微软雅黑;font-size:14px;line-height:21px;background-color:#FFFFFF;缓存模式下的轮廓查询优化方法/span
- DOI:--
- 发表时间:2014
- 期刊:同济大学学报 (自然科学版)
- 影响因子:--
- 作者:黄震华;张波;张佳雯
- 通讯作者:张佳雯
一种分布式网络中轮廓推荐的有效方法
- DOI:--
- 发表时间:2015
- 期刊:电子与信息学报
- 影响因子:--
- 作者:黄震华;张波
- 通讯作者:张波
其他文献
{{
item.title }}
{{ item.translation_title }}
- DOI:{{ item.doi || "--" }}
- 发表时间:{{ item.publish_year || "--"}}
- 期刊:{{ item.journal_name }}
- 影响因子:{{ item.factor || "--" }}
- 作者:{{ item.authors }}
- 通讯作者:{{ item.author }}
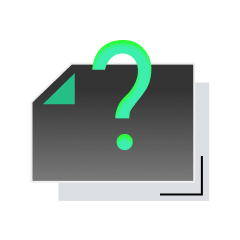
内容获取失败,请点击重试
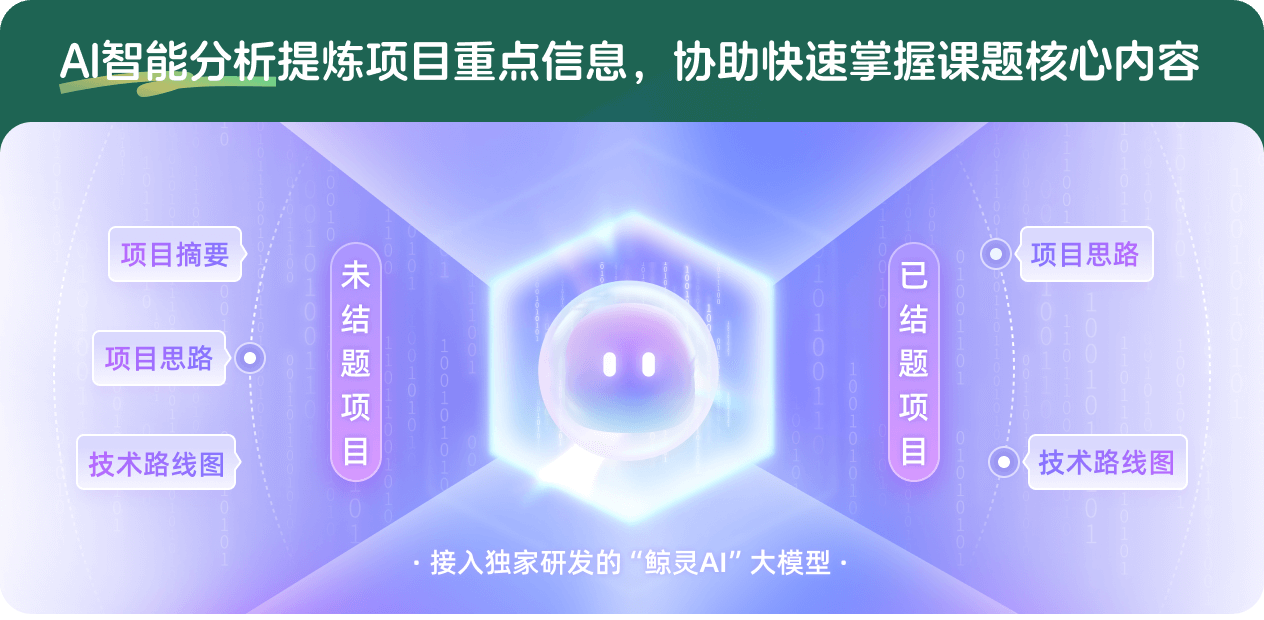
查看分析示例
此项目为已结题,我已根据课题信息分析并撰写以下内容,帮您拓宽课题思路:
AI项目摘要
AI项目思路
AI技术路线图
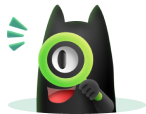
请为本次AI项目解读的内容对您的实用性打分
非常不实用
非常实用
1
2
3
4
5
6
7
8
9
10
您认为此功能如何分析更能满足您的需求,请填写您的反馈:
黄震华的其他基金
基于深度表示—度量学习的推荐方法关键问题研究
- 批准号:62172166
- 批准年份:2021
- 资助金额:60.00 万元
- 项目类别:面上项目
基于深度表示-度量学习的推荐方法关键问题研究
- 批准号:
- 批准年份:2021
- 资助金额:60 万元
- 项目类别:
相似国自然基金
{{ item.name }}
- 批准号:{{ item.ratify_no }}
- 批准年份:{{ item.approval_year }}
- 资助金额:{{ item.support_num }}
- 项目类别:{{ item.project_type }}
相似海外基金
{{
item.name }}
{{ item.translate_name }}
- 批准号:{{ item.ratify_no }}
- 财政年份:{{ item.approval_year }}
- 资助金额:{{ item.support_num }}
- 项目类别:{{ item.project_type }}