基于对抗表示学习的知识迁移关键技术研究
项目介绍
AI项目解读
基本信息
- 批准号:61876208
- 项目类别:面上项目
- 资助金额:62.0万
- 负责人:
- 依托单位:
- 学科分类:F0607.知识表示与处理
- 结题年份:2022
- 批准年份:2018
- 项目状态:已结题
- 起止时间:2019-01-01 至2022-12-31
- 项目参与者:吴国宝; 吴琦; 闫玉光; 吴汉瑞; 毕朝阳; 叶宇中; 陈仁洁; 张一帆; 张世昊;
- 关键词:
项目摘要
In the last decade, deep learning has achieved great development. However, deep learning relies on a large number of labeled training data. To handle this issue, it has attracted much attention that how to leverage knowledge extracted from related domains to assist the learning task in a target domain with limited labeled training data. The major challenge in deep transfer learning is that the data distributions of source and target domains are different. In order to build an effective deep transfer learning model, it is important to learn the latent representation shared by source and target domains. In this project, based on generative adversarial networks, we study adversarial representation learning for knowledge transfer algorithms: 1) based on adversarial learning and the manifold structure of data, we study how to learn common representations shared by source and target domains, and how to generate high-quality target data based on the learned representations. 2) Based on label information of training data, we study how to apply adversarial learning to transfer knowledge from a source domain to enhance the learning performance in the target domain, and how to adaptive reweight training data to boost the performance. 3) Based on the parameter-shared strategy, we study how to leverage multiple source domains to assist in target learning tasks. 4) Based on a new batch normalization approach with a memory mechanism, we study stable training algorithms for adversarial networks. The proposed algorithms can be evaluated and applied in real-world applications, such as medical image recognition and language translation.
深度学习在近10年得到了巨大发展,然而,深度学习的训练依赖于大量有标注的数据,如何利用相关领域学到的知识来辅助只有少量标注数据的目标领域的学习任务,已经成为广泛关注的问题。其关键难点是:不同领域的数据分布不同,必须对领域间的潜在共性或表示进行学习,从而构建高效的深度迁移学习模型。本课题在生成对抗网络的基础上,研究基于对抗表示学习的知识迁移关键算法:1)基于对抗学习和流形结构的领域共享特征表示学习算法和数据生成算法,学习有效的领域共享表示并生成高质量的目标领域数据;2)基于条件对抗表示的迁移学习算法,利用标注信息进行对抗迁移学习,并自适应地选择重要的源领域数据进行知识迁移;3)基于参数共享机制的多领域协同对抗迁移学习算法,提升多源迁移学习的性能;4)基于记忆批量归一化的对抗网络训练方法,提高生成对抗网络训练的稳定性。项目所研发的技术将在医疗影像识别、多语言翻译等任务上进行测试验证和应用。
结项摘要
整个项目期间,针对本工作的主要研究内容,发表了共41篇论文,其中SCI期刊论文29篇(包括IEEE Transaction论文16篇),EI会议论文12篇(包括CCF-A论文8篇),国内发明专利受理9项,发表论文完成情况是预期目标的273%,申请专利完成情况是预期目标的150%。. 本课题研究基于对抗表示学习的知识迁移关键算法,包括以下研究内容:1)基于对抗学习和流形结构的领域共享特征表示学习算法和数据生成算法,学习有效的领域共享表示并生成高质量的目标领域数据。项目负责人基于生成对抗网络创新式地提出了一种协作式无监督自适应算法,为数据生成提供有效解决方案;2)基于条件对抗表示的迁移学习算法,利用标注信息进行对抗迁移学习,并自适应地选择重要的源领域数据进行知识迁移。项目负责人提出了一种由浅层到深层的迁移学习模型,有效提高了网络训练速度;3)基于参数共享机制的多领域协同对抗迁移学习算法,项目负责人提出了基于参数共享的多领域协同学习,构建多个分类器的集成学习等算法,提升多源迁移学习的性能;4)基于记忆批量归一化的对抗网络训练方法,项目负责人提出了双前向传播算法,提高了生成对抗网络训练的稳定性。.
项目成果
期刊论文数量(29)
专著数量(0)
科研奖励数量(0)
会议论文数量(12)
专利数量(9)
Learning Sparse PCA with Stabilized ADMM Method on Stiefel Manifold
在 Stiefel 流形上使用稳定 ADMM 方法学习稀疏 PCA
- DOI:10.1109/tkde.2019.2935449
- 发表时间:2021
- 期刊:IEEE TRANSACTIONS ON KNOWLEDGE AND DATA ENGINEERING
- 影响因子:8.9
- 作者:Tan Mingkui;Hu Zhibin;Yan Yuguang;Cao Jiezhang;Gong Dong;Wu Qingyao
- 通讯作者:Wu Qingyao
Online Heterogeneous Transfer Learning by Knowledge Transition
通过知识转移进行在线异构迁移学习
- DOI:10.1145/3309537
- 发表时间:2019-05
- 期刊:ACM Transactions on Intelligent Systems and Technology
- 影响因子:5
- 作者:Hanrui Wu;Yuguang Yan;Yuzhong Ye;Huaqing Min;Michael K.Ng;Qingyao Wu
- 通讯作者:Qingyao Wu
Oversampling for Imbalanced Data via Optimal Transport
通过最佳传输对不平衡数据进行过采样
- DOI:10.1609/aaai.v33i01.33015605
- 发表时间:2019
- 期刊:Association for the Advancement of Artificial Intelligence (AAAI)
- 影响因子:--
- 作者:Yuguang Yan;Mingkui Tan;Yanwu Xu;Jiezhang Cao;Michael K. Ng;Huaqing Min;Qingyao Wu
- 通讯作者:Qingyao Wu
Graph neural network for 6D object pose estimation
用于 6D 物体姿态估计的图神经网络
- DOI:10.1016/j.knosys.2021.106839
- 发表时间:2021
- 期刊:KNOWLEDGE-BASED SYSTEMS
- 影响因子:8.8
- 作者:Yin Pengshuai;Ye Jiayong;Lin Guoshen;Wu Qingyao
- 通讯作者:Wu Qingyao
Iterative Refinement for Multi-Source Visual Domain Adaptation
多源视觉域适应的迭代细化
- DOI:10.1109/tkde.2020.3014697
- 发表时间:2022-06
- 期刊:IEEE Transactions on Knowledge and Data Engineering
- 影响因子:8.9
- 作者:Hanrui Wu;Yuguang Yan;Guosheng Lin;Min Yang;Michael Kwok-Po Ng;Qingyao Wu
- 通讯作者:Qingyao Wu
数据更新时间:{{ journalArticles.updateTime }}
{{
item.title }}
{{ item.translation_title }}
- DOI:{{ item.doi || "--"}}
- 发表时间:{{ item.publish_year || "--" }}
- 期刊:{{ item.journal_name }}
- 影响因子:{{ item.factor || "--"}}
- 作者:{{ item.authors }}
- 通讯作者:{{ item.author }}
数据更新时间:{{ journalArticles.updateTime }}
{{ item.title }}
- 作者:{{ item.authors }}
数据更新时间:{{ monograph.updateTime }}
{{ item.title }}
- 作者:{{ item.authors }}
数据更新时间:{{ sciAawards.updateTime }}
{{ item.title }}
- 作者:{{ item.authors }}
数据更新时间:{{ conferencePapers.updateTime }}
{{ item.title }}
- 作者:{{ item.authors }}
数据更新时间:{{ patent.updateTime }}
其他文献
Online Transfer Learning by Leveraging Multiple Source Domains
利用多个源域进行在线迁移学习
- DOI:10.1007/s10115-016-1021-1
- 发表时间:2017
- 期刊:Knowledge and Information Systems
- 影响因子:2.7
- 作者:吴庆耀
- 通讯作者:吴庆耀
其他文献
{{
item.title }}
{{ item.translation_title }}
- DOI:{{ item.doi || "--" }}
- 发表时间:{{ item.publish_year || "--"}}
- 期刊:{{ item.journal_name }}
- 影响因子:{{ item.factor || "--" }}
- 作者:{{ item.authors }}
- 通讯作者:{{ item.author }}
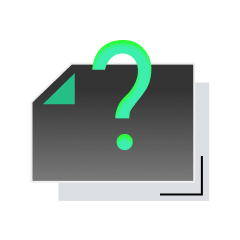
内容获取失败,请点击重试
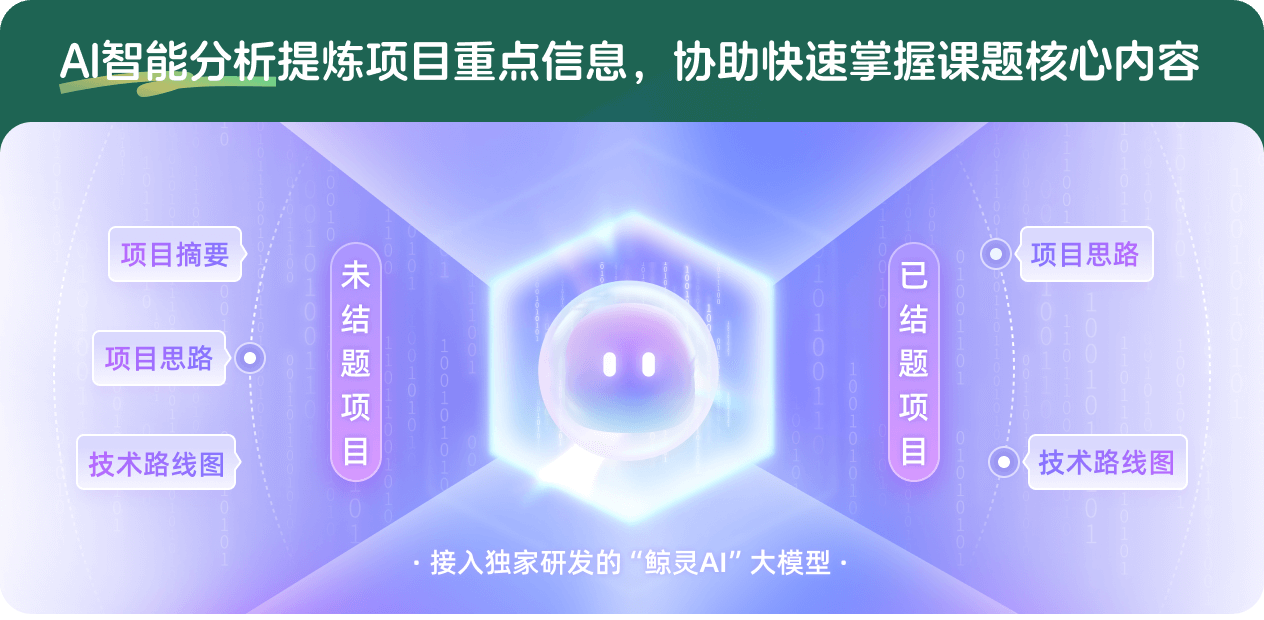
查看分析示例
此项目为已结题,我已根据课题信息分析并撰写以下内容,帮您拓宽课题思路:
AI项目摘要
AI项目思路
AI技术路线图
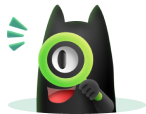
请为本次AI项目解读的内容对您的实用性打分
非常不实用
非常实用
1
2
3
4
5
6
7
8
9
10
您认为此功能如何分析更能满足您的需求,请填写您的反馈:
吴庆耀的其他基金
基于3D骨架自监督学习的视频动作表示学习研究
- 批准号:
- 批准年份:2022
- 资助金额:54 万元
- 项目类别:面上项目
基于3D骨架自监督学习的视频动作表示学习研究
- 批准号:62272172
- 批准年份:2022
- 资助金额:54.00 万元
- 项目类别:面上项目
基于概率语义分析的多关系图多类标分类方法研究
- 批准号:61502177
- 批准年份:2015
- 资助金额:21.0 万元
- 项目类别:青年科学基金项目
相似国自然基金
{{ item.name }}
- 批准号:{{ item.ratify_no }}
- 批准年份:{{ item.approval_year }}
- 资助金额:{{ item.support_num }}
- 项目类别:{{ item.project_type }}
相似海外基金
{{
item.name }}
{{ item.translate_name }}
- 批准号:{{ item.ratify_no }}
- 财政年份:{{ item.approval_year }}
- 资助金额:{{ item.support_num }}
- 项目类别:{{ item.project_type }}