用于运动想象的脑机接口深度学习模型的高效算法研究
项目介绍
AI项目解读
基本信息
- 批准号:61701270
- 项目类别:青年科学基金项目
- 资助金额:25.0万
- 负责人:
- 依托单位:
- 学科分类:F0125.医学信息检测与处理
- 结题年份:2020
- 批准年份:2017
- 项目状态:已结题
- 起止时间:2018-01-01 至2020-12-31
- 项目参与者:张迎春; 袁琦; 刘海英; 崔丽霞; 孙孟玉; 鞠宏宝;
- 关键词:
项目摘要
The feature extraction of electroencephalogram (EEG) and its motor imagery classification have become the bottleneck of performance improvement of Brain Computer Interface (BCI) technology. The project will provide direct theoretical and technical support for motor imagery based BCI system by employing deep learning theory combining with probabilistic collaborative representation and channel selection. The features of EEG signals will be described based on advantages of Long Short-Term Memory (LSTM). A deep learning network will be built to fully describe motor imagery EEG by enhancing the modeling ability of the sequential data and optimizing the topological structure of network. Additionally, adaptive channel selection method is proposed to extract EEG features based on partial channel information. A probabilistic collaborative representation based classifier is used to improve the discrimination of motor imagery EEG features, and then improve the classification accuracy and reduce computational complexity. Eventually, the motor imagery based BCI system can satisfy the requirements of real-time communication. This project will provide novel ideas and methods for EEG feature extraction and classification, and promotes the application of deep learning in BCI analysis field. It has an important theory significance and good application prospect.
脑电(EEG)信号特征提取和运动想象任务分类识别已成为阻碍脑机接口(BCI)技术性能提高的瓶颈。本项目拟基于深度学习理论,结合概率协作表示与信道选择,为基于深度学习网络模型的运动想象BCI系统提供直接的理论基础和技术支撑。利用长短时记忆元递归神经网络的优势来描述运动想象EEG,优化网络拓扑结构,增强对序列数据的建模能力,构建更适合描述运动想象EEG的特征深度学习网络,充分刻画EEG数据包含的丰富信息;同时,结合自适应信道选择方法,构造基于部分信道的EEG特征表示,然后利用概率协作表示方法,提高运动想象EEG特征的可分性,有效提高分类准确率的同时降低算法复杂度,使基于运动想象的BCI系统满足实时通信需求。本项目的开展将为EEG特征提取和分类识别研究提供新思路与新方法,有助于推动深度学习在BCI领域中的应用,具有重要的理论意义和应用前景。
结项摘要
脑电信号特征提取和分类识别是制约脑机接口(BCI)技术性能提高的瓶颈。针对人工挖掘脑电信号特征的困难,本项目基于深度学习理论,提出基于长短时记忆-全连接-梯度提升的脑电信号特征提取与分类识别算法,有效解决神经网络权值在小样本中无法得到有效训练的问题,将该算法用于BCI国际公开竞赛数据集可以达到分类准确率100%。针对脑卒中患者脑电信号分析提出基于迁移学习的脑卒中患者运动想象任务识别算法,采用EEGNet模型和微调结合,将该算法用于脑卒中患者脑电数据中可以达到分类准确率65.91%。为了解决脑卒中患者脑电数据稀缺的问题,提出基于循环一致对抗网络扩大脑卒中患者脑电数据量的算法。本项目同时探索传统方法的改进,提出基于改进S变换的运动想象分类识别优化算法,结合包裹式信道选择方法可以有效提高算法性能和降低算法复杂度。将该算法用于BCI国际公开竞赛数据集可以达到分类准确率98%和信息传输速率0.8586bit/trial。本项目的研究为脑电信号分析提供新思路与新方法,有助于推进BCI技术性能的提高,具有重要的理论意义及广阔的临床应用前景。
项目成果
期刊论文数量(11)
专著数量(0)
科研奖励数量(0)
会议论文数量(6)
专利数量(9)
Representation Learning for Motor Imagery Recognition with Deep Neural Network
使用深度神经网络进行运动意象识别的表示学习
- DOI:10.3390/electronics10020112
- 发表时间:2021-01
- 期刊:electronics
- 影响因子:2.9
- 作者:Xu Fangzhou;Rong Fenqi;Miao Yunjing;Sun Yanan;Dong Gege;Li Han;Li Jincheng;Wang Yu;ong;Leng Jiancai
- 通讯作者:Leng Jiancai
The Automatic Detection of Seizure Based on Tensor Distance And Bayesian Linear Discriminant Analysis
基于张量距离和贝叶斯线性判别分析的癫痫发作自动检测
- DOI:10.1142/s0129065721500064
- 发表时间:2021-05-01
- 期刊:INTERNATIONAL JOURNAL OF NEURAL SYSTEMS
- 影响因子:8
- 作者:Ma, Delu;Yuan, Shasha;Xu, Fangzhou
- 通讯作者:Xu, Fangzhou
Decoding spectro-temporal representation for motor imagery recognition using ECoG-based brain-computer interfaces
使用基于 ECoG 的脑机接口解码运动意象识别的光谱时间表示
- DOI:10.31083/j.jin.2020.02.1269
- 发表时间:2020-06-30
- 期刊:JOURNAL OF INTEGRATIVE NEUROSCIENCE
- 影响因子:1.8
- 作者:Xu,Fangzhou;Zheng,Wenfeng;Zhou,Weidong
- 通讯作者:Zhou,Weidong
Automatic seizure detection based on kernel robust probabilistic collaborative representation
基于核鲁棒概率协作表示的自动癫痫检测
- DOI:10.1007/s11517-018-1881-5
- 发表时间:2018-08
- 期刊:Medical & Biological Engineering & Computing
- 影响因子:3.2
- 作者:Yu Zuyi;Zhou Weidong;Zhang Fan;Xu Fangzhou;Yuan Shasha;Leng Yan;Li Yang;Yuan Qi
- 通讯作者:Yuan Qi
Scalp EEG classification using deep Bi-LSTM network for seizure detection
使用深度 Bi-LSTM 网络进行头皮脑电图分类以检测癫痫发作
- DOI:10.1016/j.compbiomed.2020.103919
- 发表时间:2020-09-01
- 期刊:COMPUTERS IN BIOLOGY AND MEDICINE
- 影响因子:7.7
- 作者:Hu, Xinmei;Yuan, Shasha;Yuan, Qi
- 通讯作者:Yuan, Qi
数据更新时间:{{ journalArticles.updateTime }}
{{
item.title }}
{{ item.translation_title }}
- DOI:{{ item.doi || "--"}}
- 发表时间:{{ item.publish_year || "--" }}
- 期刊:{{ item.journal_name }}
- 影响因子:{{ item.factor || "--"}}
- 作者:{{ item.authors }}
- 通讯作者:{{ item.author }}
数据更新时间:{{ journalArticles.updateTime }}
{{ item.title }}
- 作者:{{ item.authors }}
数据更新时间:{{ monograph.updateTime }}
{{ item.title }}
- 作者:{{ item.authors }}
数据更新时间:{{ sciAawards.updateTime }}
{{ item.title }}
- 作者:{{ item.authors }}
数据更新时间:{{ conferencePapers.updateTime }}
{{ item.title }}
- 作者:{{ item.authors }}
数据更新时间:{{ patent.updateTime }}
其他文献
其他文献
{{
item.title }}
{{ item.translation_title }}
- DOI:{{ item.doi || "--" }}
- 发表时间:{{ item.publish_year || "--"}}
- 期刊:{{ item.journal_name }}
- 影响因子:{{ item.factor || "--" }}
- 作者:{{ item.authors }}
- 通讯作者:{{ item.author }}
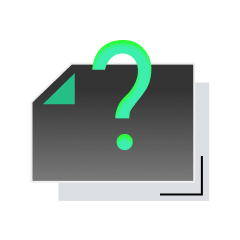
内容获取失败,请点击重试
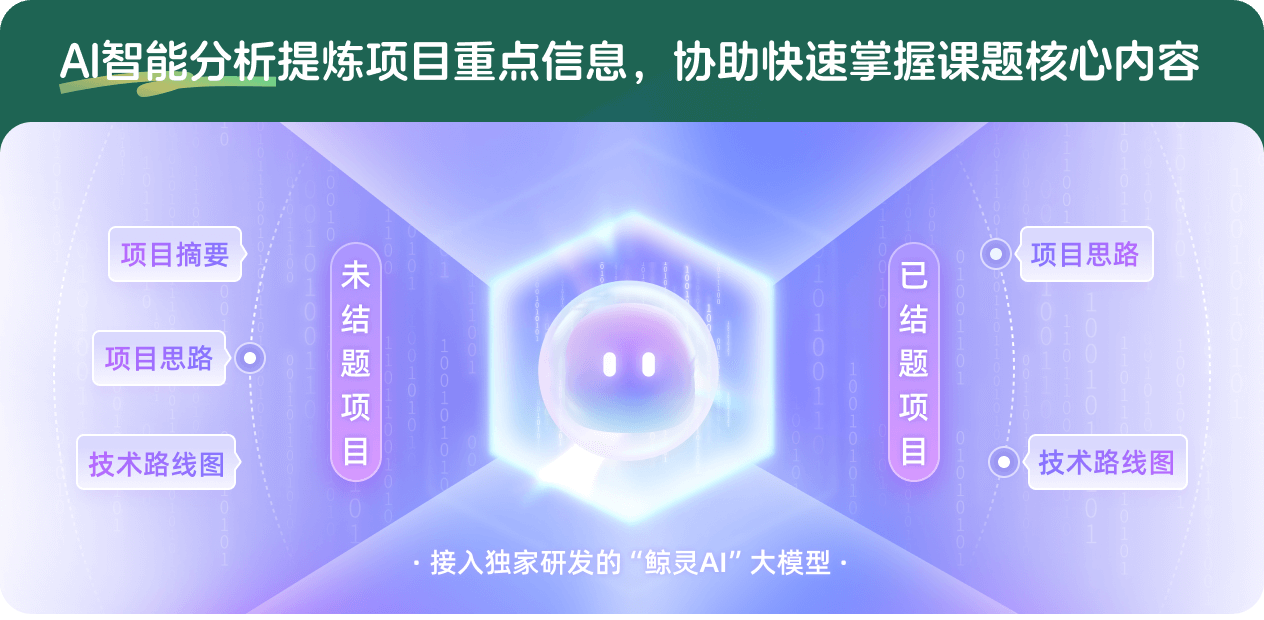
查看分析示例
此项目为已结题,我已根据课题信息分析并撰写以下内容,帮您拓宽课题思路:
AI项目摘要
AI项目思路
AI技术路线图
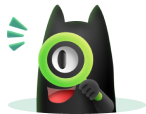
请为本次AI项目解读的内容对您的实用性打分
非常不实用
非常实用
1
2
3
4
5
6
7
8
9
10
您认为此功能如何分析更能满足您的需求,请填写您的反馈:
相似国自然基金
{{ item.name }}
- 批准号:{{ item.ratify_no }}
- 批准年份:{{ item.approval_year }}
- 资助金额:{{ item.support_num }}
- 项目类别:{{ item.project_type }}
相似海外基金
{{
item.name }}
{{ item.translate_name }}
- 批准号:{{ item.ratify_no }}
- 财政年份:{{ item.approval_year }}
- 资助金额:{{ item.support_num }}
- 项目类别:{{ item.project_type }}