若干图划分问题基于学习的多层进化算法研究
项目介绍
AI项目解读
基本信息
- 批准号:61703213
- 项目类别:青年科学基金项目
- 资助金额:22.0万
- 负责人:
- 依托单位:
- 学科分类:F0307.导航、制导与控制
- 结题年份:2020
- 批准年份:2017
- 项目状态:已结题
- 起止时间:2018-01-01 至2020-12-31
- 项目参与者:张慧峰; 翁盛煊; 陈剑波; 程子豪; 杨天宝; 陈磊; 孙孝魁; 单延逍; 葛阳鸣;
- 关键词:
项目摘要
Graph partitioning problems are a kind of NP-hard combinatorial optimization problems with a number of real-world applications, such as parallel computing, very large scale integration (VLSI), image segmentation, etc. At present, the existing algorithms need to be further improved in the performance. This project is dedicated to investigating systemically four graph partitioning problems which are closely related to each other, i.e., the balanced graph partitioning problem with a minimum cut, the balanced graph partitioning problem minimizing the maximum domain boundary, the non-uniform graph partitioning problem with a minimum cut, and the balanced graph partitioning problem with a lower bound constraint on the capacities of subsets of vertex set, and devising respectively a learning-based multi-level evolutionary algorithm for each of them. The main researching contents in the algorithm design are as follows: (1) the coarsening methods of graph for the multi-level optimization methods; (2) the local search methods, the crossover operators, and the population updating strategies for the evolutionary algorithms; (3) the learning mechanisms for the evolutionary algorithms. This project is not only able to promote the study and development of the learning-based intelligent optimization algorithms, but also to provide the highly efficient optimization tools for many real-world applications.
图划分问题是一类具有大量现实应用的、NP难的组合优化问题,其应用背景包括并行计算、大规模集成电路设计(VLSI)、图像分割等。目前,现有图划分算法的性能有待进一步改进。本项目致力于系统地研究以下4个紧密相关的图划分问题:最小割的均衡图划分、最小最大块边界的均衡图划分、最小割的非均衡图划分、有容量下界约束的均衡图划分,并为其分别设计基于学习的多层进化算法。算法的主要研究内容包括:(1) 多层优化算法的图粗化方法;(2) 进化算法的局部搜索程序、杂交算子与种群更新策略;(3) 进化算法的学习机制。本项目不仅能促进基于学习的智能优化算法的研究与发展,也能为许多现实应用提供高效的优化工具。
结项摘要
图划分问题与0-1组合优化问题是运筹学与计算机领域的两类经典的优化问题,具有重要的理论价值和应用背景。本项目对若干图划分问题和0-1组合优化问题进行了深入的研究,这些问题包括最大多样性分组问题、电网解列问题、约束的聚类问题、多维背包问题、多需求多维背包问题和公平疏散问题。本项目的主要研究成果包括:1)为图划分问题和聚类问题提出了一种具有较高通用性的邻域分解技术,并基于该技术提出了若干高性能的优化算法;2)为0-1组合优化问题发展了若干基于解的禁忌搜索策略;3)为0-1组合优化问题提出了若干高性能的混合进化算法。本项目提出的优化策略和优化技术具有较好的通用性,可为其他组合优化问题的求解提供借鉴作用。本项目提出的图划分算法、0-1组合优化算法可应用于并行计算、大规模集成电路设计、设施选址等领域。
项目成果
期刊论文数量(8)
专著数量(0)
科研奖励数量(0)
会议论文数量(3)
专利数量(2)
A Tabu Search Approach With Dynamical Neighborhood Size for Solving the Maximum Min-Sum Dispersion Problem
求解最大最小和色散问题的动态邻域大小禁忌搜索方法
- DOI:10.1109/access.2019.2959315
- 发表时间:2019
- 期刊:IEEE Access
- 影响因子:3.9
- 作者:Lai Xiangjing;Fu Zhang-Hua
- 通讯作者:Fu Zhang-Hua
Intensification-driven tabu search for the minimum differential dispersion problem
集约化驱动的禁忌搜索最小差分色散问题
- DOI:10.1016/j.knosys.2019.01.010
- 发表时间:2019-03
- 期刊:Knowledge-Based Systems
- 影响因子:8.8
- 作者:Lai Xiangjing;Hao Jin Kao;Glover Fred;Yue Dong
- 通讯作者:Yue Dong
Neighborhood decomposition based variable neighborhood search and tabu search for maximally diverse grouping
基于邻域分解的变量邻域搜索和最大多样化分组的禁忌搜索
- DOI:10.1016/j.ejor.2020.07.048
- 发表时间:2021-03-16
- 期刊:EUROPEAN JOURNAL OF OPERATIONAL RESEARCH
- 影响因子:6.4
- 作者:Lai, Xiangjing;Hao, Jin-Kao;Yue, Dong
- 通讯作者:Yue, Dong
A study of two evolutionary/tabu search approaches for the generalized max-mean dispersion problem
广义最大均值色散问题的两种进化/禁忌搜索方法的研究
- DOI:10.1016/j.eswa.2019.112856
- 发表时间:2020
- 期刊:Expert Systems with Applications
- 影响因子:8.5
- 作者:Lai Xiangjing;Hao Jin-Kao;Glover Fred
- 通讯作者:Glover Fred
Solution-based tabu search for the maximum min-sum dispersion problem
基于解的禁忌搜索最大最小和色散问题
- DOI:10.1016/j.ins.2018.02.006
- 发表时间:2018-05-01
- 期刊:INFORMATION SCIENCES
- 影响因子:8.1
- 作者:Lai, Xiangjing;Yue, Dong;Glover, Fred
- 通讯作者:Glover, Fred
数据更新时间:{{ journalArticles.updateTime }}
{{
item.title }}
{{ item.translation_title }}
- DOI:{{ item.doi || "--"}}
- 发表时间:{{ item.publish_year || "--" }}
- 期刊:{{ item.journal_name }}
- 影响因子:{{ item.factor || "--"}}
- 作者:{{ item.authors }}
- 通讯作者:{{ item.author }}
数据更新时间:{{ journalArticles.updateTime }}
{{ item.title }}
- 作者:{{ item.authors }}
数据更新时间:{{ monograph.updateTime }}
{{ item.title }}
- 作者:{{ item.authors }}
数据更新时间:{{ sciAawards.updateTime }}
{{ item.title }}
- 作者:{{ item.authors }}
数据更新时间:{{ conferencePapers.updateTime }}
{{ item.title }}
- 作者:{{ item.authors }}
数据更新时间:{{ patent.updateTime }}
其他文献
数学史在数学专业课程教学中的应用研究
- DOI:--
- 发表时间:2013
- 期刊:宜春学院学报
- 影响因子:--
- 作者:李师煜;赖向京;王观石
- 通讯作者:王观石
Lennard-Jones团簇最低能量构型的预测
- DOI:--
- 发表时间:2011
- 期刊:中国科学:化学
- 影响因子:--
- 作者:赖向京;许如初;黄文奇
- 通讯作者:黄文奇
An artificial bee algorithm with a leading group and its application into image registration
一种带引导群的人工蜜蜂算法及其在图像配准中的应用
- DOI:10.1007/s11042-019-7211-6
- 发表时间:2019-01
- 期刊:Multimedia Tools and Applications
- 影响因子:3.6
- 作者:胡海东;Chi-Man Pun;刘烨;赖向京;杨泽宇;高浩
- 通讯作者:高浩
其他文献
{{
item.title }}
{{ item.translation_title }}
- DOI:{{ item.doi || "--" }}
- 发表时间:{{ item.publish_year || "--"}}
- 期刊:{{ item.journal_name }}
- 影响因子:{{ item.factor || "--" }}
- 作者:{{ item.authors }}
- 通讯作者:{{ item.author }}
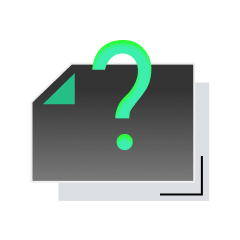
内容获取失败,请点击重试
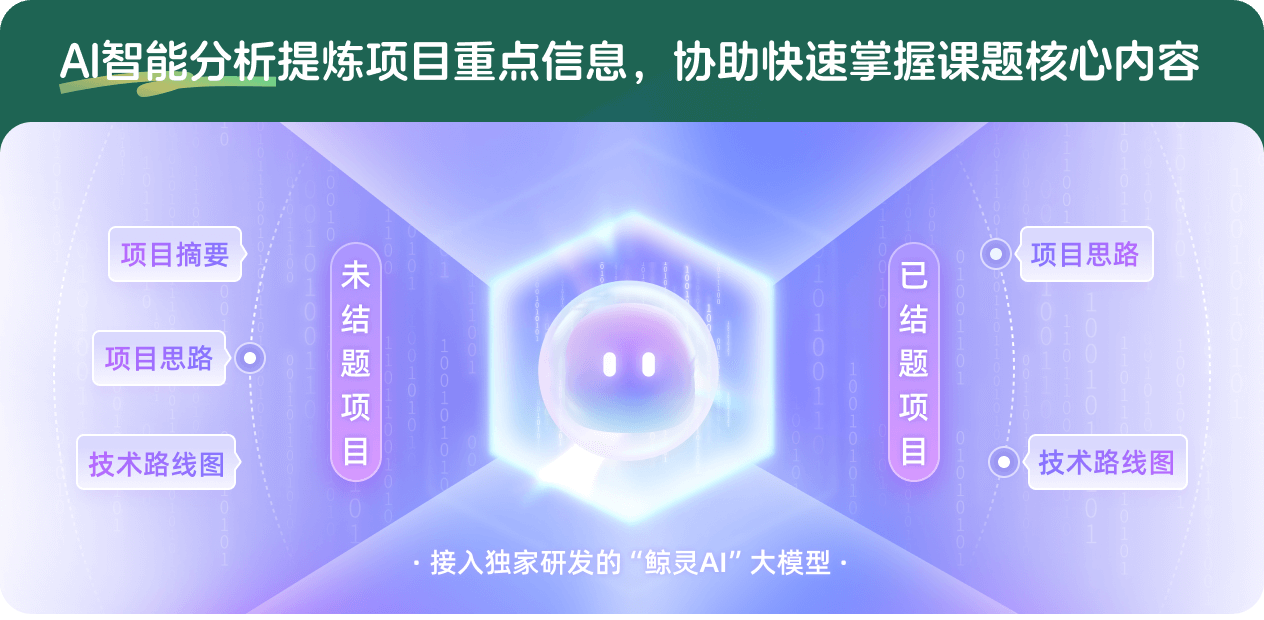
查看分析示例
此项目为已结题,我已根据课题信息分析并撰写以下内容,帮您拓宽课题思路:
AI项目摘要
AI项目思路
AI技术路线图
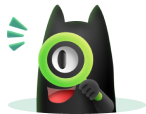
请为本次AI项目解读的内容对您的实用性打分
非常不实用
非常实用
1
2
3
4
5
6
7
8
9
10
您认为此功能如何分析更能满足您的需求,请填写您的反馈:
相似国自然基金
{{ item.name }}
- 批准号:{{ item.ratify_no }}
- 批准年份:{{ item.approval_year }}
- 资助金额:{{ item.support_num }}
- 项目类别:{{ item.project_type }}
相似海外基金
{{
item.name }}
{{ item.translate_name }}
- 批准号:{{ item.ratify_no }}
- 财政年份:{{ item.approval_year }}
- 资助金额:{{ item.support_num }}
- 项目类别:{{ item.project_type }}