基于强化学习的数据中心网络自适应拥塞控制方法研究
项目介绍
AI项目解读
基本信息
- 批准号:61802263
- 项目类别:青年科学基金项目
- 资助金额:25.0万
- 负责人:
- 依托单位:
- 学科分类:F0207.计算机网络
- 结题年份:2021
- 批准年份:2018
- 项目状态:已结题
- 起止时间:2019-01-01 至2021-12-31
- 项目参与者:刘志丹; 姚俊梅; 陈昭; 黄明亮; 龚增阳; 阎宏铭; 陈滢滢;
- 关键词:
项目摘要
In data center networks, the congestion control algorithms play a key role in providing efficient data transmission and improving application performance. However, current congestion control algorithms are facing performance issues. They cannot satisfy the performance requirements of different types of traffic, and encounter the incast problem. Although researchers have proposed many improvement approaches, there is one approach not yet been researched sufficiently, that is the adaptive congestion control. This approach dynamically adapts a congestion control algorithm to network and traffic conditions, improving transmission performance. A powerful way to adjust the behavior of a congestion control algorithm is modifying its critical parameters. The existing researches on this topic are still very rare and have a lot of shortcomings: 1) only one parameter is adjusted; 2) only heuristics or simple rules are designed which cannot achieve optimal performance; 3) multiple conflicting objectives cannot be optimized jointly. To overcome these disadvantages, we research on an adaptive congestion control method, which can support the adjustment of multiple parameters, can optimize transmission performance and balance conflicting objectives. However, it is very challenging to formulate the effect of a congestion control algorithm on the transmission performance. Therefore, we propose to leverage the recent reinforcement learning techniques to address the adaptive congestion control problem. Moreover, we propose a full suite of solutions, including theoretical method and system implementation.
在数据中心网络里,拥塞控制算法在提供高效数据传输和提高应用性能方面发挥着关键作用。然而,目前的拥塞控制算法面临着性能不佳的问题:无法同时满足不同类型流量的不同性能需求,和聚播问题。尽管研究学者已提出了很多改善方法,但仍有一种方法尚未得到充分的研究:自适应拥塞控制方法。这一方法通过动态调节拥塞控制算法使其适应网络和流量的变化,进而改善传输性能。调节拥塞控制算法的重要手段之一就是调节其关键参数。已有的相关研究还很少,且有很多不足:1)只涉及单个调节参数;2)只是启发式算法,无法最优化性能;3)无法同时优化多个冲突的目标。为了克服这些不足,我们将研究一种自适应拥塞控制方法,其支持多变量的联合调节,并以提高传输性能为优化目标,且能够权衡冲突的目标。然而,分析拥塞控制算法对传输性能的影响是个难题。对此我们提出利用强化学习技术解决自适应拥塞控制方法,并研究一整套完整的解决方案,包括理论方法和系统实现。
结项摘要
拥塞控制在提供高效数据传输和提高应用性能方面发挥着关键作用。本项目围绕自适应拥塞控制问题展开研究。我们主要研究了如何动态调节初始拥塞窗口,使得流传输时间最短,并且造成的拥塞最低。我们也研究了如何提高深度学习决策模型的运行效率,并维持预测的准确度。我们提出了一个基于深度强化学习的自适应在线控制方法,通过有效且高效的深度学习决策模型和自适应学习方法,在动态网络状况下,能够自主地、稳定地、快速地学习出最优控制策略。我们探索了提高深度学习决策模型运行效率的不同方法,为提升基于深度学习的拥塞控制方法的运行效率提供了理论基础。此外,我们将研究延伸至低时延应用的成本管理问题,提出了一种共享感知的在线任务卸载方法,使得每位用户的延迟要求得到满足,同时最小化所需服务器的成本。本项目研究有助于提升云计算系统和边缘计算系统中应用的传输效率和性能。
项目成果
期刊论文数量(7)
专著数量(0)
科研奖励数量(0)
会议论文数量(0)
专利数量(3)
Cross-Technology Communication for Heterogeneous Wireless Devices through Symbol-Level Energy Modulation
通过符号级能量调制实现异构无线设备的跨技术通信
- DOI:10.1109/tmc.2021.3065998
- 发表时间:2022
- 期刊:IEEE Transactions on Mobile Computing
- 影响因子:7.9
- 作者:Junmei Yao;Xiaolong Zheng;Ruitao Xie;Kaishun Wu
- 通讯作者:Kaishun Wu
Adaptive Offloading for Time-Critical Tasks in Heterogeneous Internet of Vehicles
异构车联网中时间关键型任务的自适应卸载
- DOI:10.1109/jiot.2020.2997720
- 发表时间:2020-05
- 期刊:IEEE Internet of Things Journal
- 影响因子:10.6
- 作者:Chunhui Liu;Kai Liu;Songtao Guo;Ruitao Xie;Victor C. S. Lee;Sang H. Son
- 通讯作者:Sang H. Son
Adaptive Online Decision Method for Initial Congestion Window in 5G Mobile Edge Computing Using Deep Reinforcement Learning
使用深度强化学习的 5G 移动边缘计算初始拥塞窗口自适应在线决策方法
- DOI:10.1109/jsac.2019.2959187
- 发表时间:2020-02
- 期刊:IEEE Journal on Selected Areas in Communications
- 影响因子:16.4
- 作者:Ruitao Xie;Xiaohua Jia;Kaishun Wu
- 通讯作者:Kaishun Wu
Blockchain-Based Decentralized Public Auditing for Cloud Storage
基于区块链的云存储去中心化公共审计
- DOI:10.1109/tcc.2021.3051622
- 发表时间:2022-10-01
- 期刊:IEEE TRANSACTIONS ON CLOUD COMPUTING
- 影响因子:6.5
- 作者:Shu, Jiangang;Zou, Xing;Xie, Ruitao
- 通讯作者:Xie, Ruitao
ENERGY EFFICIENCY ENHANCEMENT FOR CNN BASED DEEP MOBILE SENSING
基于 CNN 的深度移动传感的能源效率增强
- DOI:10.1109/mwc.2019.1800321
- 发表时间:2019-06-01
- 期刊:IEEE WIRELESS COMMUNICATIONS
- 影响因子:12.9
- 作者:Xie, Ruitao;Jia, Xiaohua;Wu, Kaishun
- 通讯作者:Wu, Kaishun
数据更新时间:{{ journalArticles.updateTime }}
{{
item.title }}
{{ item.translation_title }}
- DOI:{{ item.doi || "--"}}
- 发表时间:{{ item.publish_year || "--" }}
- 期刊:{{ item.journal_name }}
- 影响因子:{{ item.factor || "--"}}
- 作者:{{ item.authors }}
- 通讯作者:{{ item.author }}
数据更新时间:{{ journalArticles.updateTime }}
{{ item.title }}
- 作者:{{ item.authors }}
数据更新时间:{{ monograph.updateTime }}
{{ item.title }}
- 作者:{{ item.authors }}
数据更新时间:{{ sciAawards.updateTime }}
{{ item.title }}
- 作者:{{ item.authors }}
数据更新时间:{{ conferencePapers.updateTime }}
{{ item.title }}
- 作者:{{ item.authors }}
数据更新时间:{{ patent.updateTime }}
其他文献
其他文献
{{
item.title }}
{{ item.translation_title }}
- DOI:{{ item.doi || "--" }}
- 发表时间:{{ item.publish_year || "--"}}
- 期刊:{{ item.journal_name }}
- 影响因子:{{ item.factor || "--" }}
- 作者:{{ item.authors }}
- 通讯作者:{{ item.author }}
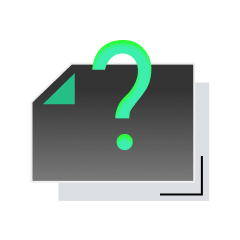
内容获取失败,请点击重试
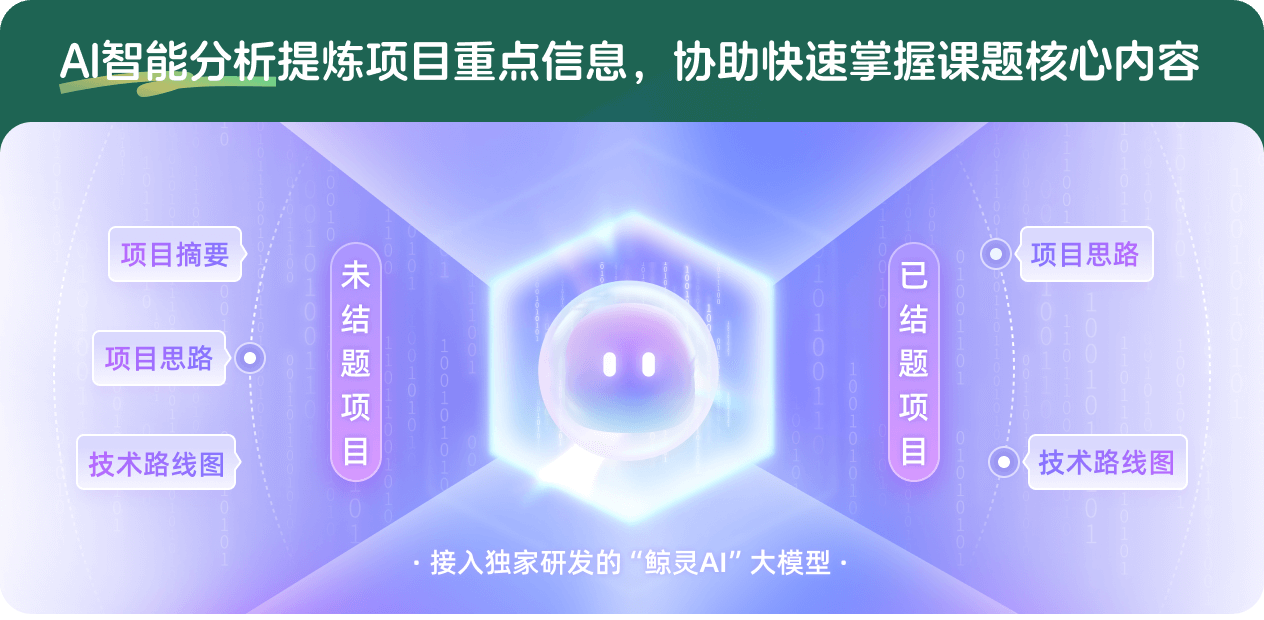
查看分析示例
此项目为已结题,我已根据课题信息分析并撰写以下内容,帮您拓宽课题思路:
AI项目摘要
AI项目思路
AI技术路线图
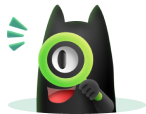
请为本次AI项目解读的内容对您的实用性打分
非常不实用
非常实用
1
2
3
4
5
6
7
8
9
10
您认为此功能如何分析更能满足您的需求,请填写您的反馈:
谢瑞桃的其他基金
面向交互式多媒体应用的云边计算关键技术研究
- 批准号:
- 批准年份:2022
- 资助金额:54 万元
- 项目类别:面上项目
面向交互式多媒体应用的云边计算关键技术研究
- 批准号:62272316
- 批准年份:2022
- 资助金额:54.00 万元
- 项目类别:面上项目
相似国自然基金
{{ item.name }}
- 批准号:{{ item.ratify_no }}
- 批准年份:{{ item.approval_year }}
- 资助金额:{{ item.support_num }}
- 项目类别:{{ item.project_type }}
相似海外基金
{{
item.name }}
{{ item.translate_name }}
- 批准号:{{ item.ratify_no }}
- 财政年份:{{ item.approval_year }}
- 资助金额:{{ item.support_num }}
- 项目类别:{{ item.project_type }}