具有采样约束的最快检测问题在分布式传感器网络中的应用
项目介绍
AI项目解读
基本信息
- 批准号:61601144
- 项目类别:青年科学基金项目
- 资助金额:22.0万
- 负责人:
- 依托单位:
- 学科分类:F0111.信号理论与信号处理
- 结题年份:2019
- 批准年份:2016
- 项目状态:已结题
- 起止时间:2017-01-01 至2019-12-31
- 项目参与者:冀振元; 吴小川; 曹明阳; 石运梅; 姚国伟; 张柏强;
- 关键词:
项目摘要
Quickest detection is a framework that focuses on the design of sequential detection algorithms to identify changes in the distributions of stochastic processes as quickly and reliably as possible. This framework has a wide range of applications in wireless sensor networks. In most of existing works, it is assumed that there is no constraint on how many samples can be taken. In this project, taking physical limitations of distributed sensor networks into account, we propose to study the quickest detection problem with fixed sampling right constraints and stochastic sampling right constraints, respectively. . These sampling right constraints present several challenges and unique features to quickest detection problems. It is crucial to design adaptive sensing strategies that rely on information extracted from samples taken so far to make sampling and detection decisions. To this end, using tools from optimal stopping time, sequential analysis and renewal process, we aim to achieve the following goals in the project: 1) to characterize the optimal detection schemes for problems with these additional constraints. 2) to understand the performance loss caused by these additional constraints. 3) to design low-complexity but asymptotically optimal detection schemes. . The research, if successful, will make substantial contributions to both theory and applications. On the theoretical level, the proposed project will advance the state of the art of sequential analysis and detection theory. On the application level, our research has the potential to substantially improve the efficiency and robustness of detection algorithms utilized in various sensor networks.
最快检测问题的目的是在满足可靠性的前提下,以最短的检测延时在线检测出所观测随机过程发生的突发变化。这个问题在传感器网络监测周围环境的异常变化中具有重要的应用。目前多数的研究工作假定传感器可以观测无限多个采样样本。在本项目中,我们考虑传感器网络的物理限制,对最快检测问题分别施加确定性的采样约束和随机性的采样约束,提出新的算法。. 研究问题中的新挑战在于如何根据观测样本自适应地改变传感器的采样策略。针对此关键问题,研究者拟用最优停止时间,序贯分析,更新过程等工具,完成以下研究目标:1) 求解最优检测方法;2) 分析采样约束对检测性能的影响;3) 设计低复杂度,鲁棒性强的次优或渐进最优的检测方法。. 本项目的研究意义在于:在理论层面上,本研究成果可以进一步丰富现有的序贯分析和信号检测理论的内容;在应用层面上,可以大大提高传感器网络在对周围环境的突发变化检测中的检测能力。
结项摘要
最快检测问题的目的是在满足可靠性的前提下,以最短的检测延时在线实时检测出所观测随机过程发生的突发变化。这个问题在传感器网络监测周围环境的异常变化中具有重要的应用。本课题以研究传感器网络中的最快检测问题为背景,在研究过程分别考虑了线性回归模型的最快检测问题;变化后参数未知的最快检测问题;多数据源的最快检测问题和高维未知向量的快速估计问题等四个子问题。对所研究的四个子问题分别提出了并行和算法、M-SR/M-CUSUM算法、CUSUM算法和自适应正交基投影算法等低复杂度、鲁棒性强的次优算法或渐进最优算法,并通过仿真数据或实测数据等手段验证了所提出算法的正确性,有效性和可用性。在研究过程中,研究者使用了最优停止时间,序贯分析,更新过程等高等数学工具,从理论上证明分析了所提出算法的性能, 本项目的研究意义在于:在理论层面上,本研究成果进一步丰富现有的序贯分析、信号检测与估计理论、最快检测理论、以及传感器网络的理论研究内容;在应用层面上,本研究所提出的一系列低计算复杂度的算法对最快检测理论的实际应用产生了一定的推动作用,并且本研究的结果可以被其他领域所借鉴,存在一定的参考价值。
项目成果
期刊论文数量(1)
专著数量(0)
科研奖励数量(0)
会议论文数量(3)
专利数量(0)
Online Change-Point Detection of Linear Regression Models
线性回归模型的在线变点检测
- DOI:10.1109/tsp.2019.2914893
- 发表时间:2019-05
- 期刊:IEEE Transactions on Signal Processing
- 影响因子:5.4
- 作者:Geng Jun;Zhang Bingwen;Huie Lauren M;Lai Lifeng
- 通讯作者:Lai Lifeng
数据更新时间:{{ journalArticles.updateTime }}
{{
item.title }}
{{ item.translation_title }}
- DOI:{{ item.doi || "--"}}
- 发表时间:{{ item.publish_year || "--" }}
- 期刊:{{ item.journal_name }}
- 影响因子:{{ item.factor || "--"}}
- 作者:{{ item.authors }}
- 通讯作者:{{ item.author }}
数据更新时间:{{ journalArticles.updateTime }}
{{ item.title }}
- 作者:{{ item.authors }}
数据更新时间:{{ monograph.updateTime }}
{{ item.title }}
- 作者:{{ item.authors }}
数据更新时间:{{ sciAawards.updateTime }}
{{ item.title }}
- 作者:{{ item.authors }}
数据更新时间:{{ conferencePapers.updateTime }}
{{ item.title }}
- 作者:{{ item.authors }}
数据更新时间:{{ patent.updateTime }}
其他文献
其他文献
{{
item.title }}
{{ item.translation_title }}
- DOI:{{ item.doi || "--" }}
- 发表时间:{{ item.publish_year || "--"}}
- 期刊:{{ item.journal_name }}
- 影响因子:{{ item.factor || "--" }}
- 作者:{{ item.authors }}
- 通讯作者:{{ item.author }}
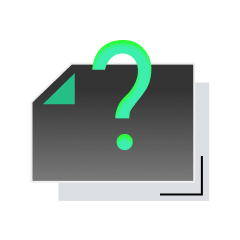
内容获取失败,请点击重试
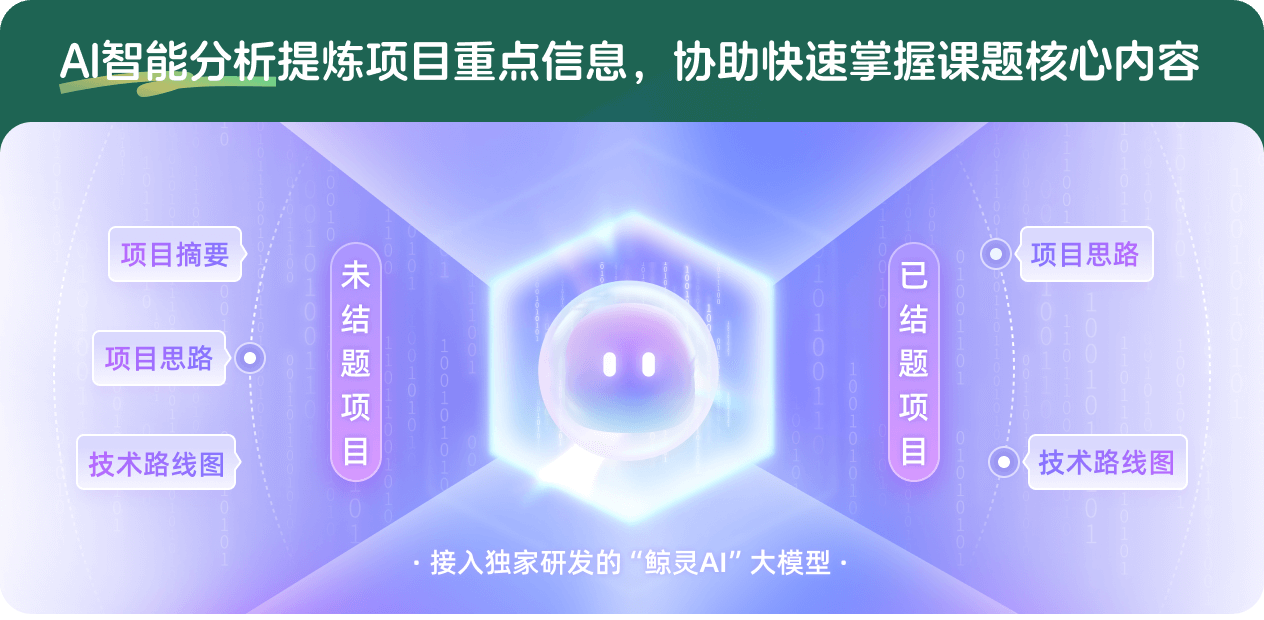
查看分析示例
此项目为已结题,我已根据课题信息分析并撰写以下内容,帮您拓宽课题思路:
AI项目摘要
AI项目思路
AI技术路线图
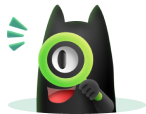
请为本次AI项目解读的内容对您的实用性打分
非常不实用
非常实用
1
2
3
4
5
6
7
8
9
10
您认为此功能如何分析更能满足您的需求,请填写您的反馈:
相似国自然基金
{{ item.name }}
- 批准号:{{ item.ratify_no }}
- 批准年份:{{ item.approval_year }}
- 资助金额:{{ item.support_num }}
- 项目类别:{{ item.project_type }}
相似海外基金
{{
item.name }}
{{ item.translate_name }}
- 批准号:{{ item.ratify_no }}
- 财政年份:{{ item.approval_year }}
- 资助金额:{{ item.support_num }}
- 项目类别:{{ item.project_type }}