子采样排序与相关熵排序的学习理论研究
项目介绍
AI项目解读
基本信息
- 批准号:11671161
- 项目类别:面上项目
- 资助金额:48.0万
- 负责人:
- 依托单位:
- 学科分类:A0602.信息技术与不确定性的数学理论与方法
- 结题年份:2020
- 批准年份:2016
- 项目状态:已结题
- 起止时间:2017-01-01 至2020-12-31
- 项目参与者:邓小炎; 潘志斌; 熊慧娟; 杨文宇; 宋必芹; 刘绍轩; 袁佩佩; 沈世健; 来会会;
- 关键词:
项目摘要
In this project, we will study the mathematical theory of learning to rank. Considering the computation difficulty of ranking with a large number of data, we introduce Nyström approximation and Markov sampling approaches for the ranking model and provide the fast optimization algorithms. We focus on the study of learning theory foundations of the subsampling ranking, and provide the theory analysis including consistency and convergence rate in terms of error analysis associated with the operator approximation and the capacity estimation of assumption space. This study will demonstrate the role of sampling method on generalization ability and computation complexity. Meanwhile, considering the non-Gaussian noises and outliers of the ranking data, we introduce the information theoretic learning to the ranking problem and construct the ranking model under maximum correntropy criterion, and provide the theory analysis on its generalization ability and robustness. Finally, the proposed algorthms are applied to bioinformatic data, and their effectiveness is verified on the drug discovery and the protein homology detection. This project is expected to break through the limitation of traditional ranking for a large number of data, and establish the mathematical foundations of learning to rank.
本项目研究排序机器学习的数学理论基础。针对大规模数据下排序算法的计算难题,将Nyström逼近和Markov采样引入排序学习模型,构建快速排序算法,重点探讨其学习理论基础,分别利用基于容量估计和基于算子逼近的误差分析方法建立其一致性和收敛速度的分析,阐明采样方法对排序学习泛化能力和计算复杂性的影响机制。同时,针对数据中非高斯噪声和噪点问题,将信息理论学习引入排序模型,构建基于最大相关熵准则的排序学习算法,探讨其泛化能力和鲁棒性能。最后,将设计算法应用于生物信息数据,探索其在药物发现和蛋白质同源检测等排序任务中的有效性。本项目期望在面向大规模数据的排序算法设计和数学理论分析方面取得突破,建立排序机器学习的数学理论基础。
结项摘要
本项目对正则排序相关算法的学习理论与应用进行了深入的研究。项目组按照研究计划,有序推进研究工作,圆满完成了研究目标。主要研究成果包括三个部分:一是建立了几类正则排序算法学习率的刻画,分析了分布式、去偏置及交互策略对泛化能力的影响,通过数据实验验证了相关策略提升计算效率和预测性能的有效性; 二是证明了基于Nyström采样和Markov采样正则学习算法的误差界,阐明了采样机制与计算复杂性、泛化性的关系;三是设计了几类稀疏可加模型,给出了其泛化误差界和变量选择一致性分析,并在高维数据挖掘中验证了其良好的性能。本项目相关研究不仅发展和丰富了排序学习的数学基础,也为大规模数据情形的算法设计和应用提供了理论指导。
项目成果
期刊论文数量(13)
专著数量(1)
科研奖励数量(0)
会议论文数量(5)
专利数量(0)
Multiscale Gaussian convolution algorithm for estimate of Gaussian mixture model
高斯混合模型估计的多尺度高斯卷积算法
- DOI:10.1080/03610926.2018.1523431
- 发表时间:2018-12
- 期刊:Communications in Statistics - Theory and Methods
- 影响因子:--
- 作者:Xia Rui;Zhang Qiuyue;Deng Xiaoyan
- 通讯作者:Deng Xiaoyan
Modal Regression-Based Atomic Representation for Robust Face Recognition and Reconstruction
基于模态回归的原子表示,用于鲁棒人脸识别和重建
- DOI:10.1109/tcyb.2019.2903205
- 发表时间:2020
- 期刊:IEEE Transactions on Cybernetics
- 影响因子:--
- 作者:Wang Yulong;Tang Yuan Yan;Li Luoqing;Chen Hong
- 通讯作者:Chen Hong
Quantitative trait loci identification for brain endophenotypes via new additive model with random networks
通过随机网络的新加法模型对脑内表型进行定量性状位点识别
- DOI:10.1093/bioinformatics/bty557
- 发表时间:2018-09
- 期刊:Bioinformatics
- 影响因子:5.8
- 作者:Xiaoqian Wang;Hong Chen;Jingwen Yan;Kwangsik Nho;Shannon L Risacher;Andrew J Saykin;Li Shen;Heng Huang
- 通讯作者:Heng Huang
Sparse Modal Additive Model
稀疏模态相加模型
- DOI:10.1109/tnnls.2020.3005144
- 发表时间:2021-06-01
- 期刊:IEEE TRANSACTIONS ON NEURAL NETWORKS AND LEARNING SYSTEMS
- 影响因子:10.4
- 作者:Chen, Hong;Wang, Yingjie;Huang, Heng
- 通讯作者:Huang, Heng
Generalization Analysis of Fredholm Kernel Regularized Classifiers
Fredholm核正则化分类器的泛化分析
- DOI:10.1162/neco_a_00967
- 发表时间:2017-06
- 期刊:Neural Computation
- 影响因子:2.9
- 作者:Gong Tieliang;Xu Zongben;Chen Hong
- 通讯作者:Chen Hong
数据更新时间:{{ journalArticles.updateTime }}
{{
item.title }}
{{ item.translation_title }}
- DOI:{{ item.doi || "--"}}
- 发表时间:{{ item.publish_year || "--" }}
- 期刊:{{ item.journal_name }}
- 影响因子:{{ item.factor || "--"}}
- 作者:{{ item.authors }}
- 通讯作者:{{ item.author }}
数据更新时间:{{ journalArticles.updateTime }}
{{ item.title }}
- 作者:{{ item.authors }}
数据更新时间:{{ monograph.updateTime }}
{{ item.title }}
- 作者:{{ item.authors }}
数据更新时间:{{ sciAawards.updateTime }}
{{ item.title }}
- 作者:{{ item.authors }}
数据更新时间:{{ conferencePapers.updateTime }}
{{ item.title }}
- 作者:{{ item.authors }}
数据更新时间:{{ patent.updateTime }}
其他文献
Florisil固相萃取法联用气相色谱测定油脂中sn-2位脂肪酸
- DOI:--
- 发表时间:2015
- 期刊:中国油料作物学报
- 影响因子:--
- 作者:魏芳;吕昕;董绪燕;陈洪
- 通讯作者:陈洪
我国7 种典型热带木本油料加工研究现状
- DOI:--
- 发表时间:2019
- 期刊:热带农业科学
- 影响因子:--
- 作者:涂行浩;杜丽清;魏芳;吕昕;黄凤洪;陈洪
- 通讯作者:陈洪
采用枯草芽孢杆菌发酵菜籽饼粕生产溶栓酶条件的优化
- DOI:--
- 发表时间:2008
- 期刊:中国油料作物学报
- 影响因子:--
- 作者:陈洪;罗凡;刘笔锋;胡磊
- 通讯作者:胡磊
微波辅助分步酶解菜籽粕制备菜籽多肽的研究
- DOI:--
- 发表时间:2010
- 期刊:中国油脂
- 影响因子:--
- 作者:黄凤洪;赵元弟;李光明;陈洪
- 通讯作者:陈洪
北三台凸起周缘三叠系物源体系与沉积演化
- DOI:--
- 发表时间:2018
- 期刊:中国矿业大学学报
- 影响因子:--
- 作者:肖杰;王健;操应长;徐琦松;秦志军;陈洪
- 通讯作者:陈洪
其他文献
{{
item.title }}
{{ item.translation_title }}
- DOI:{{ item.doi || "--" }}
- 发表时间:{{ item.publish_year || "--"}}
- 期刊:{{ item.journal_name }}
- 影响因子:{{ item.factor || "--" }}
- 作者:{{ item.authors }}
- 通讯作者:{{ item.author }}
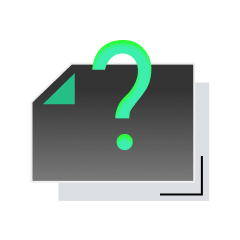
内容获取失败,请点击重试
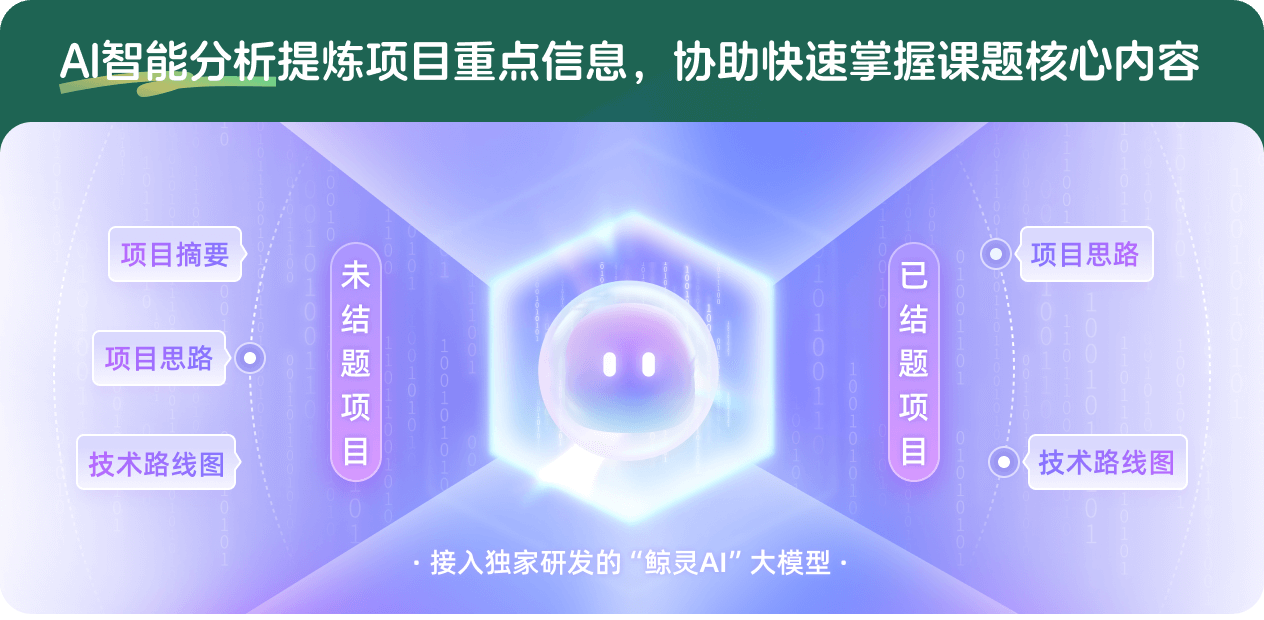
查看分析示例
此项目为已结题,我已根据课题信息分析并撰写以下内容,帮您拓宽课题思路:
AI项目摘要
AI项目思路
AI技术路线图
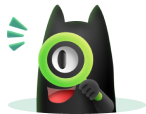
请为本次AI项目解读的内容对您的实用性打分
非常不实用
非常实用
1
2
3
4
5
6
7
8
9
10
您认为此功能如何分析更能满足您的需求,请填写您的反馈:
陈洪的其他基金
结构Knockoff推断算法及其理论研究
- 批准号:62376104
- 批准年份:2023
- 资助金额:49 万元
- 项目类别:面上项目
稳健可加模型的学习理论与应用
- 批准号:
- 批准年份:2020
- 资助金额:51 万元
- 项目类别:面上项目
基于逼近理论的半监督学习误差分析研究
- 批准号:11001092
- 批准年份:2010
- 资助金额:18.0 万元
- 项目类别:青年科学基金项目
相似国自然基金
{{ item.name }}
- 批准号:{{ item.ratify_no }}
- 批准年份:{{ item.approval_year }}
- 资助金额:{{ item.support_num }}
- 项目类别:{{ item.project_type }}
相似海外基金
{{
item.name }}
{{ item.translate_name }}
- 批准号:{{ item.ratify_no }}
- 财政年份:{{ item.approval_year }}
- 资助金额:{{ item.support_num }}
- 项目类别:{{ item.project_type }}