非结构化数据模式分析中的多核融合理论与学习方法研究
项目介绍
AI项目解读
基本信息
- 批准号:61202332
- 项目类别:青年科学基金项目
- 资助金额:25.0万
- 负责人:
- 依托单位:
- 学科分类:F0605.模式识别与数据挖掘
- 结题年份:2015
- 批准年份:2012
- 项目状态:已结题
- 起止时间:2013-01-01 至2015-12-31
- 项目参与者:付光远; 蔡艳宁; 郭金库; 杨晓君; 胡来红; 陈柏松; 杨东方;
- 关键词:
项目摘要
Multiple kernel learning is a new research focus in current machine learning field. In this project, the efficient multiple kernel fusion theories and learning methods will be studied facing two typical pattern analysis problems based on a large amount of unstructured data in the command information system. The two problems are the processing and automatic target recognition of SAR remote sensing information and the real-time state monitoring of the missile weapon system. In theory, the fusion of kernels with different scale effects, the fusion of multi-scale kernels, the fusion between multi-resolution feature extraction and multi-scale kernel method, and design of the corresponding kernel machines are regard as the key directions. The basic theoretical research mainly expands from three perspectives, which are the pattern classification with high real-time requirement, the universal algorithms on multiple kernels' adaptive learning and fast online learning of composite kernel machines. To meet the requirements of practical applications, and fully considering the influence factors, such as noise, high dimension and heterogeneity, local deletion and the singular value, multivariate and non-flat, the structured representation method is studied for unstructured data, on this basis, the algorithms are promoted from both theory and performance by investigating the adaptability, the stability and speed. In applications, relying on the software and hardware environment of the command automation lab, the effectiveness and advancement of algorithms will be verified through a comprehensive experiment using the real data. The relevant research and results will provide effective technical support and reliable decision basis for the command information system, which possesses important theoretical sense and practical values.
多核学习是当前机器学习领域一个新的研究热点。本项目基于指挥信息系统中大量的非结构化数据,面向SAR遥感情报信息的处理与自动目标识别、导弹武器系统实时状态监测两类典型模式分析问题,拟研究高效的多核融合理论及多核学习方法。在理论上,以具有不同尺度效应核函数的融合、多尺度核的融合、多分辨特征提取与多尺度核方法的融合及相应核机器的设计为重点方向,从高实时性模式分类,通用多核的自适应学习,合成核机器的快速在线学习等角度展开研究。并从实际需求入手,充分考查噪声、高维异构、局部缺失和奇异值、多元与非平坦等因素下的非结构化数据,研究其结构化表示方法,从适应性、稳定性及速度等方面对算法进行理论改进和性能提升。在应用方面,依托指挥自动化实验室软、硬件环境,利用真实数据进行综合实验,验证所提算法的有效性和先进性,为部队指挥信息系统提供有效的技术支撑和可靠的决策依据,具有重要的理论意义和实用价值。
结项摘要
非结构化数据的模式分析问题作战指挥面临的核心问题,对其进行合理的处理、识别、预测和利用,为部队指挥信息系统提供有效的技术支撑和可靠的决策依据,具有重要的理论意义和实用价值。为解决这一问题,本项目从多核融合理论及其学习方法入手,从模式分类和回归分析两方面展开研究。. 主要研究内容包括四个方面:. 一、非结构化数据的结构化表示方法,主要技术要点包括:基于局部特征的非结构化数据表示方法;基于数据修正的非结构化数据表示方法。. 二、基于非结构化特征与多尺度核机器的自动目标识别方法,主要技术要点包括: 受视觉细胞感受野模型的启发,提出了一种从图像局部点出发,对图像进行多分辨分解的图像处理新方法,并将其应用到MSTAR数据集中的SAR图像目标的特征提取。基于多级特征的整合思想,运用基于多尺度核方法的SVM模型,实现了三类目标的分类,得到了很高的正确率。针对多核机器系数优化学习问题,首先研究了一种基于核目标度量的多尺度小波核分类器及其学习方法。此外,还研究了一种基于分类概率估计的多核机器度量优化方法,提高了合成核机器的特征适应性和分类正确率,并将其应用于大类别图像的检索。. 三、非结构化时序预测与基于合成核机器的快速在线学习算法,关键要点包括:针对混沌时间序列的在线预测问题,提出了一种基于核目标度量的无偏多尺度核模型及其在线学习算法。通过改进最小二乘支持向量回归模型的结构风险形式,提出了一种无偏的回归机器模型,同时,通过引入多尺度核方法,并采用核目标度量对多核系数进行优化,提高了多核机器的预测精度;无偏最小二乘支持向量回归模型的使用,使得回归系数的求取大大简化,在此基础上,研究了一种基于Cholesky分解的快速在线学习算法,提高了在线预测的效率。. 四、理论验证及实验研究。依托指挥自动化实验室软、硬件环境,利用大量数据进行综合实验,验证了所提算法的有效性和先进性。
项目成果
期刊论文数量(14)
专著数量(2)
科研奖励数量(2)
会议论文数量(1)
专利数量(0)
一种新的惯性/视觉组合系统初始对准的时域约束方法
- DOI:--
- 发表时间:2014
- 期刊:仪器仪表学报
- 影响因子:--
- 作者:杨东方;刘志国;张金生;刘华平
- 通讯作者:刘华平
Multivariate Time Series Prediction Based on Multi-Output Support Vector Regression
基于多输出支持向量回归的多元时间序列预测
- DOI:10.1007/978-3-642-37832-4_35
- 发表时间:2014
- 期刊:Advances in Intelligent Systems and Computing
- 影响因子:--
- 作者:Yanning Cai;Hongqiao Wang;Xuemei Ye;Li An
- 通讯作者:Li An
Classification Probability Estimation Based Multi-Class Image Retrieval
基于分类概率估计的多类图像检索
- DOI:10.1007/978-3-642-37832-4_36
- 发表时间:2014
- 期刊:Advances in Intelligent Systems and Computing
- 影响因子:--
- 作者:Yanning Cai;Shicheng Wang;Guangyuan Fu;Linlin Li
- 通讯作者:Linlin Li
一种未知环境下机器人多目标跟踪算法
- DOI:--
- 发表时间:2015
- 期刊:智能系统学报
- 影响因子:--
- 作者:伍明;李琳琳;魏振华;汪洪桥
- 通讯作者:汪洪桥
Robust 2DPCA With Non-greedy l1-Norm Maximization for Image Analysis
用于图像分析的具有非贪婪 l1 范数最大化的鲁棒 2DPCA
- DOI:10.1109/tcyb.2014.2341575
- 发表时间:2015-05-01
- 期刊:IEEE TRANSACTIONS ON CYBERNETICS
- 影响因子:11.8
- 作者:Wang, Rong;Nie, Feiping;Yao, Minli
- 通讯作者:Yao, Minli
数据更新时间:{{ journalArticles.updateTime }}
{{
item.title }}
{{ item.translation_title }}
- DOI:{{ item.doi || "--"}}
- 发表时间:{{ item.publish_year || "--" }}
- 期刊:{{ item.journal_name }}
- 影响因子:{{ item.factor || "--"}}
- 作者:{{ item.authors }}
- 通讯作者:{{ item.author }}
数据更新时间:{{ journalArticles.updateTime }}
{{ item.title }}
- 作者:{{ item.authors }}
数据更新时间:{{ monograph.updateTime }}
{{ item.title }}
- 作者:{{ item.authors }}
数据更新时间:{{ sciAawards.updateTime }}
{{ item.title }}
- 作者:{{ item.authors }}
数据更新时间:{{ conferencePapers.updateTime }}
{{ item.title }}
- 作者:{{ item.authors }}
数据更新时间:{{ patent.updateTime }}
其他文献
Data-driven fault prediction and anomaly measurement for complex systems using support vector probability density estimation
使用支持向量概率密度估计对复杂系统进行数据驱动的故障预测和异常测量
- DOI:10.1016/j.engappai.2017.09.008
- 发表时间:2018
- 期刊:Engineering Application of Artificial Intelligence
- 影响因子:--
- 作者:汪洪桥;蔡艳宁;付光远;伍明
- 通讯作者:伍明
非平坦函数概率密度估计
- DOI:--
- 发表时间:2016
- 期刊:计算机科学与探索
- 影响因子:--
- 作者:汪洪桥;蔡艳宁;付光远;王仕成
- 通讯作者:王仕成
基于图像序列的地面慢动多目标识别与跟踪
- DOI:--
- 发表时间:2016
- 期刊:激光与光电子学进展
- 影响因子:--
- 作者:汪洪桥;蔡艳宁;付光远;伍明;王仕成
- 通讯作者:王仕成
其他文献
{{
item.title }}
{{ item.translation_title }}
- DOI:{{ item.doi || "--" }}
- 发表时间:{{ item.publish_year || "--"}}
- 期刊:{{ item.journal_name }}
- 影响因子:{{ item.factor || "--" }}
- 作者:{{ item.authors }}
- 通讯作者:{{ item.author }}
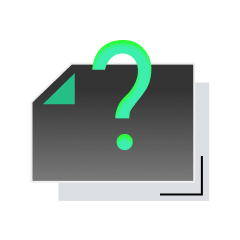
内容获取失败,请点击重试
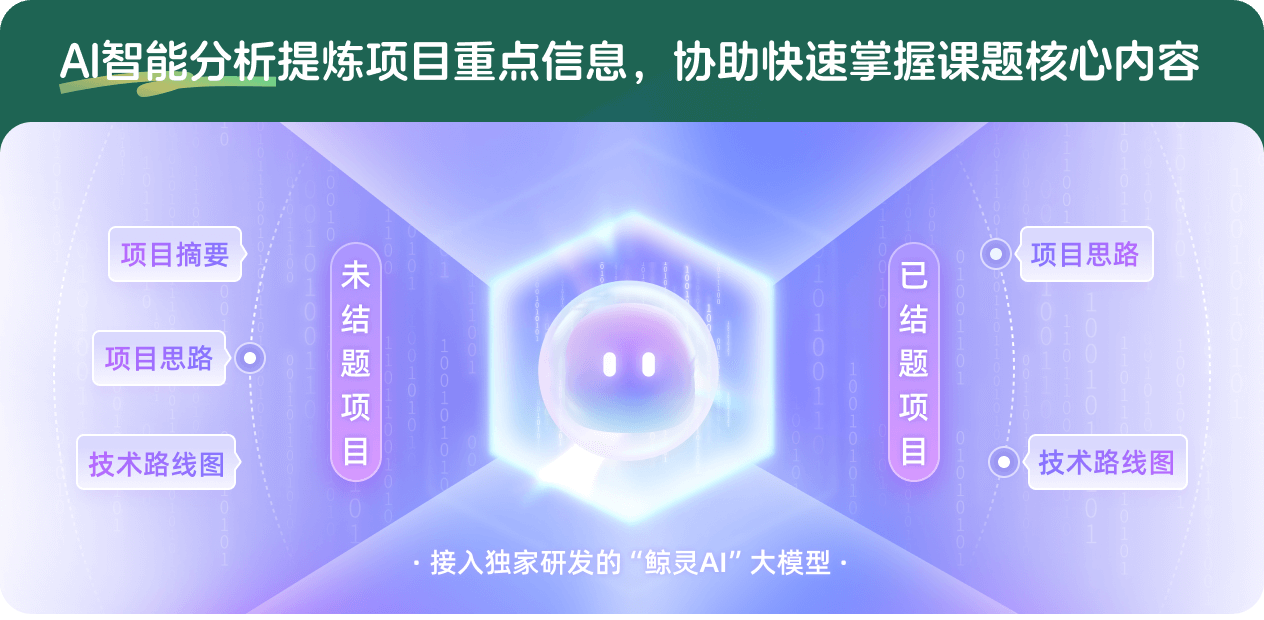
查看分析示例
此项目为已结题,我已根据课题信息分析并撰写以下内容,帮您拓宽课题思路:
AI项目摘要
AI项目思路
AI技术路线图
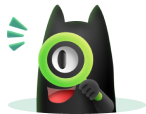
请为本次AI项目解读的内容对您的实用性打分
非常不实用
非常实用
1
2
3
4
5
6
7
8
9
10
您认为此功能如何分析更能满足您的需求,请填写您的反馈:
相似国自然基金
{{ item.name }}
- 批准号:{{ item.ratify_no }}
- 批准年份:{{ item.approval_year }}
- 资助金额:{{ item.support_num }}
- 项目类别:{{ item.project_type }}
相似海外基金
{{
item.name }}
{{ item.translate_name }}
- 批准号:{{ item.ratify_no }}
- 财政年份:{{ item.approval_year }}
- 资助金额:{{ item.support_num }}
- 项目类别:{{ item.project_type }}