面向FAST射电望远镜数据预处理和压缩传输研究
项目介绍
AI项目解读
基本信息
- 批准号:U1831118
- 项目类别:联合基金项目
- 资助金额:42.0万
- 负责人:
- 依托单位:
- 学科分类:A3304.数据、计算和信息提取等应用基础性研究
- 结题年份:2021
- 批准年份:2018
- 项目状态:已结题
- 起止时间:2019-01-01 至2021-12-31
- 项目参与者:潘之辰; 缪晨晨; 夏铭; 吴迪; 丁伟; 李晓川; 朱涵林; 朱海浩; 刘天洋;
- 关键词:
项目摘要
To solve the bottlenecks arising from the movement of the massive radio observation data of the Five hundred meter Aperture Spherical radio Telescope (FAST), a path-finder project of the Square Kilometre Array radio telescope (SKA), we propose to study the noise characteristics of the multi-beam radio survey data and the spatial correlation characteristics of the multi-beam data to form the preprocessing based compression methods for the multi-beam radio survey data of FAST. These proposed methods will be based on the codesign methodology aquired in the process of our previous research regarding SKA prototyping design and the preprocessing and compression schemes in the domain of big data analytics. At the same time, we propose to study the preprocessing based compression methods and customized transmission mechanism for multi-beam radio search data, in order to compose a highly efficient customized transmission protocol that supports the movement of the radio observation data in 19 beams of the FAST telescope from the antenna to the computing cluster. We further propose to optimize the implementation of multi-beam data preprocessing and compression through the co-design of hardware and software. By verifying our implementation at the site of FAST telescope, we expect to make a breakthrough at the bottleneck of FAST massive radio observation data movement, and promote the progress to enhance FAST telescope from single beam debugging mode to multi -beam observation operational mode as an important step to speed up the process of China's major astronomical discoveries.
针对平方公里阵列射电望远镜(SKA)的先导项目- 500米口径球面射电望远镜(FAST)的海量射电观测数据移动的瓶颈,结合我们前期参与国际SKA原型设计研究取得的协同设计方法和在大数据领域积累的预处理与传输研究成果,提出研究FAST多波束射电搜寻数据的噪声特征和多波束数据的空间相关特征,形成面向多波束射电搜寻数据的预处理压缩方法;同时研究面向FAST多波束射电搜寻数据的压缩传输机制,形成支撑FAST望远镜19个波束的射电观测数据从天线到计算集群的高效自定义传输协议;进一步通过软硬件协同设计优化实现多波束数据预处理压缩和自定义传输方法,并通过在FAST台址的现场验证实现,尝试突破FAST海量射电观测数据移动的瓶颈,推进FAST望远镜从单波束调试模式向多波束观测模式转化,加快我国取得重大天文科学发现的进展。
结项摘要
针对平方公里阵列射电望远镜(SKA)的先导项目- 500米口径球面射电望远镜(FAST)的海量射电观测数据移动的瓶颈,结合我们前期参与国际SKA原型设计研究取得的协同设计方法和在大数据领域积累的预处理与传输研究成果,提出研究FAST多波束射电搜寻数据的噪声特征和多波束数据的空间相关特征,形成面向多波束射电搜寻数据的预处理压缩方法;同时研究面向FAST多波束射电搜寻数据的压缩传输机制,形成支撑FAST望远镜19个波束的射电观测数据从天线到计算集群的高效自定义传输协议;进一步通过软硬件协同设计优化实现多波束数据预处理压缩和自定义传输方法,并通过远程接入FAST现场进行验证,尝试突破FAST海量射电观测数据移动的瓶颈,推进FAST望远镜从单波束调试模式向多波束观测模式转化,加快我国取得重大天文科学发现的进展。..在执行过程中,中科院上海高等研究院与国家天文台密切配合,全面完成了论文、人才和技术指标。
项目成果
期刊论文数量(13)
专著数量(0)
科研奖励数量(0)
会议论文数量(4)
专利数量(0)
FPGA based acceleration of game theory algorithm in edge computing for autonomous driving
基于FPGA的自动驾驶边缘计算博弈论算法加速
- DOI:10.1016/j.sysarc.2018.12.009
- 发表时间:2019-02
- 期刊:Journal of Systems Architecture
- 影响因子:4.5
- 作者:Du Sen;Huang Tian;Hou Junjie;Song Shijin;Song Yuefeng
- 通讯作者:Song Yuefeng
An efficient convolutional neural network for small traffic sign detection
用于小型交通标志检测的高效卷积神经网络
- DOI:10.1016/j.sysarc.2019.01.012
- 发表时间:2019-08
- 期刊:Journal of Systems Architecture
- 影响因子:4.5
- 作者:Song Shijin;Que Zhiqiang;Hou Junjie;Du Sen;Song Yuefeng
- 通讯作者:Song Yuefeng
FPGA-Based Scale-Out Prototyping of Degridding Algorithm for Accelerating Square Kilometre Array Telescope Data Processing
基于 FPGA 的横向扩展去网格算法原型,加速平方公里阵列望远镜数据处理
- DOI:10.1109/access.2020.2966666
- 发表时间:2020-01
- 期刊:IEEE Access
- 影响因子:3.9
- 作者:Hou Junjie;Zhu Yongxin;Du Sen;Song Shijin;Song Yuefeng
- 通讯作者:Song Yuefeng
Astronomical Data Preprocessing Implementation Based on FPGA and Data Transformation Strategy for the FAST Telescope as a Giant CPS
FAST巨型CPS天文数据预处理实现及数据转换策略
- DOI:10.1109/access.2020.2981816
- 发表时间:2020
- 期刊:IEEE Access
- 影响因子:3.9
- 作者:Song Yuefeng;Zhu Yongxin;Hou Junjie;Du Sen;Song Shijin
- 通讯作者:Song Shijin
Mapping Large LSTMs to FPGAs with Weight Reuse
通过权重重用将大型 LSTM 映射到 FPGA
- DOI:10.1007/s11265-020-01549-8
- 发表时间:2020-07
- 期刊:Journal of Signal Processing Systems for Signal Image and Video Technology
- 影响因子:1.8
- 作者:Zhiqiang Que;Yongxin Zhu;Hongxiang Fan;Jiuxi Meng;Xinyu Niu;Wayne Luk
- 通讯作者:Wayne Luk
数据更新时间:{{ journalArticles.updateTime }}
{{
item.title }}
{{ item.translation_title }}
- DOI:{{ item.doi || "--"}}
- 发表时间:{{ item.publish_year || "--" }}
- 期刊:{{ item.journal_name }}
- 影响因子:{{ item.factor || "--"}}
- 作者:{{ item.authors }}
- 通讯作者:{{ item.author }}
数据更新时间:{{ journalArticles.updateTime }}
{{ item.title }}
- 作者:{{ item.authors }}
数据更新时间:{{ monograph.updateTime }}
{{ item.title }}
- 作者:{{ item.authors }}
数据更新时间:{{ sciAawards.updateTime }}
{{ item.title }}
- 作者:{{ item.authors }}
数据更新时间:{{ conferencePapers.updateTime }}
{{ item.title }}
- 作者:{{ item.authors }}
数据更新时间:{{ patent.updateTime }}
其他文献
基于卷积目标检测的3D 眼球追踪系统深度估计
- DOI:--
- 发表时间:2018
- 期刊:仪器仪表学报
- 影响因子:--
- 作者:潘新星;汪辉;陈灵;祝永新;杨傲雷
- 通讯作者:杨傲雷
其他文献
{{
item.title }}
{{ item.translation_title }}
- DOI:{{ item.doi || "--" }}
- 发表时间:{{ item.publish_year || "--"}}
- 期刊:{{ item.journal_name }}
- 影响因子:{{ item.factor || "--" }}
- 作者:{{ item.authors }}
- 通讯作者:{{ item.author }}
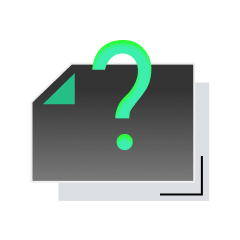
内容获取失败,请点击重试
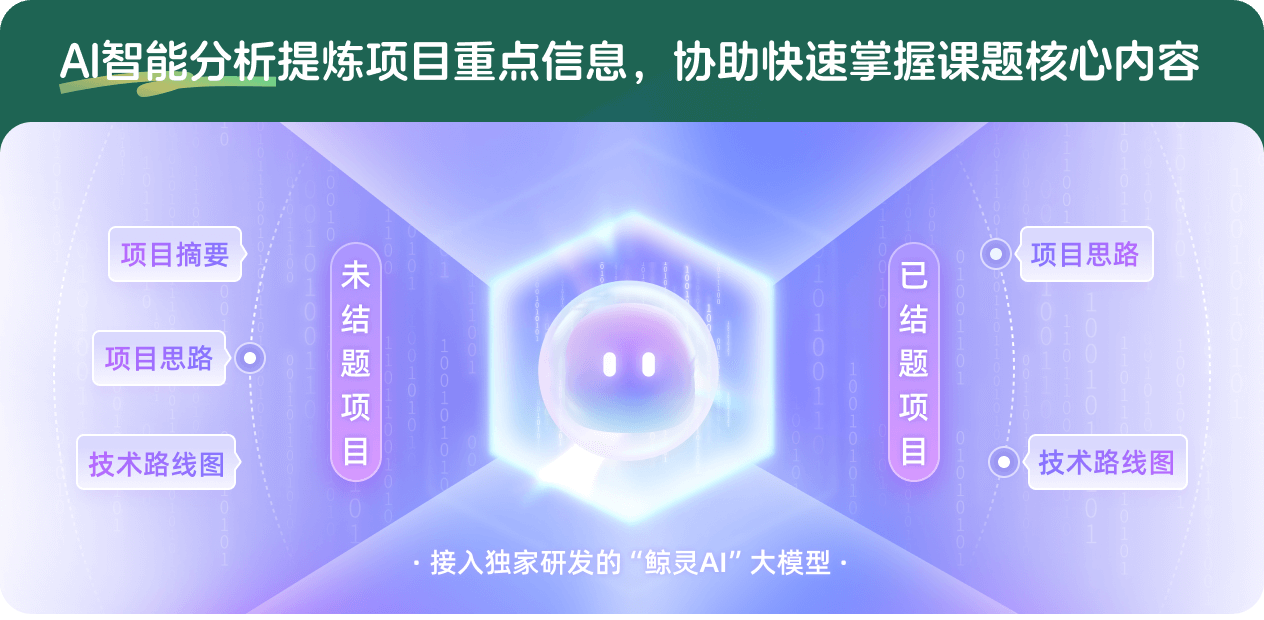
查看分析示例
此项目为已结题,我已根据课题信息分析并撰写以下内容,帮您拓宽课题思路:
AI项目摘要
AI项目思路
AI技术路线图
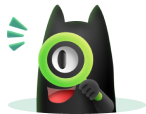
请为本次AI项目解读的内容对您的实用性打分
非常不实用
非常实用
1
2
3
4
5
6
7
8
9
10
您认为此功能如何分析更能满足您的需求,请填写您的反馈:
祝永新的其他基金
面向科学大装置超大规模数据流的定制计算研究
- 批准号:
- 批准年份:2020
- 资助金额:50 万元
- 项目类别:联合基金项目
相似国自然基金
{{ item.name }}
- 批准号:{{ item.ratify_no }}
- 批准年份:{{ item.approval_year }}
- 资助金额:{{ item.support_num }}
- 项目类别:{{ item.project_type }}
相似海外基金
{{
item.name }}
{{ item.translate_name }}
- 批准号:{{ item.ratify_no }}
- 财政年份:{{ item.approval_year }}
- 资助金额:{{ item.support_num }}
- 项目类别:{{ item.project_type }}