面向语音合成的神经网络声码器研究
项目介绍
AI项目解读
基本信息
- 批准号:61871358
- 项目类别:面上项目
- 资助金额:63.0万
- 负责人:
- 依托单位:
- 学科分类:F0117.多媒体信息处理
- 结题年份:2022
- 批准年份:2018
- 项目状态:已结题
- 起止时间:2019-01-01 至2022-12-31
- 项目参与者:戴礼荣; 胡亚军; 阮玉平; 伍宏传; 张雅洁; 艾杨; 张景宣;
- 关键词:
项目摘要
Speech synthesis is an important research topic in the fields of speech information processing and artificial intelligence. Vocoder is a core module of statistical parametric speech synthesis. The traditional source-filter vocoder has the problems of the loss of spectrum details and phase, and the lack of nonlinear processing capability. In recent years, the emergence of neural network vocoder can alleviate the above problems, but there are still some deficiencies in its theoretical model and application effects. Therefore, this project intends to conduct a research on the neural network vocoder for speech synthesis, including the neural network waveform modeling and generation method with source-filter structure, the feature extraction method of the neural network vocoder, the efficiency optimization and parallel computing methods of the neural network vocoder, the adaptive training methods of neural network vocoder with limited data and so on. The purposes of this project include to explore a new model of speech generation with nonlinear filtering, to complete the neural network vocoder framework, to significantly reduce the naturalness gap between synthetic speech and human voice under the premise of run-time efficiency, and to enhance the effects of personalized speech synthesis with limited data of target speakers. The research on the waveform generation neural network and other aspects in this project can also have important reference value for the research on voice conversion, speech enhancement, speech coding and other related fields.
语音合成是语音信息处理与人工智能领域的重要研究方向,声码器是统计参数语音合成的核心模块。传统源-滤波器声码器存在频谱细节与相位丢失、非线性处理能力缺乏等问题。近年来出现的神经网络声码器可改善以上问题,但是在理论模型和应用效果方面仍存在不足。因此,本项目拟开展面向语音合成的神经网络声码器研究,包括源-滤波器结构的神经网络波形建模与生成方法、神经网络声码器的特征提取方法、神经网络声码器的效率优化与并行计算方法、数据受限情况下神经网络声码器的自适应训练方法等。本项目旨在通过以上研究工作探索非线性滤波的语音生成新模型,完善神经网络声码器框架,在保证运行效率的前提下显著缩小统计参数方法合成语音与真人语音的自然度差距,提升目标话者少数据量情况下的个性化语音合成效果。本项目中波形生成神经网络等方面的研究工作对于声音转换、语音增强、语音编码等其他相关领域研究也有重要的参考价值。
结项摘要
声码器是统计参数语音合成的关键模块之一。本项目立项时神经网络声码器研究在理论模型和应用效果方面存在诸多不足,包括波形生成模型黑箱问题、特征提取模型研究欠缺、波形生成精度仍需提升、波形生成过程复杂度高、训练数据规模依赖性强等。本项目针对以上不足主要开展了4个方面的研究工作:(1)在源-滤波器结构的神经网络波形建模与生成方法方面,提出了基于幅度相位谱分级预测的神经网络声码器HiNet,进一步研究了神经网络声码器的混响控制方法、以及混响与噪声消除方法;(2)在神经网络声码器的特征提取方法方面,设计了基于WaveNet的波形自编码器,实现了包含分析端与生成端的完整神经网络声码器;(3)在神经网络声码器的效率优化与并行计算方法方面,提出了面向低比特神经网络声码器的谱增强方法,以及基于幅度相位谱平行直接预测的全帧级神经网络声码器APNet;(4)在数据受限情况下神经网络声码器的自适应训练方法方面,提出了神经网络声码器的在线话者自适应方法,并设计实现了结合声源滤波的话者无关声码器EPWG与SF-GAN。项目研究成果发表学术论文共25篇,包括IEEE/ACM 汇刊论文8篇,语音技术领域顶级国际学术会议ICASSP、Interspeech论文8篇;申请国家发明专利3项。项目培养毕业研究生7人,包括博士5人、硕士2人。本项目通过以上研究工作,探索了非线性滤波的语音生成新模型,完善了神经网络声码器建模方法,在保证运行效率的前提下显著提高了统计参数方法合成语音的自然度与相似度,研究成果对于语音增强、语音编码等相关领域研究也有着重要的参考价值。
项目成果
期刊论文数量(8)
专著数量(1)
科研奖励数量(2)
会议论文数量(17)
专利数量(3)
Non-Parallel Sequence-to-Sequence Voice Conversion with Disentangled Linguistic and Speaker Representations
具有解开语言和说话人表示的非并行序列到序列语音转换
- DOI:10.1109/taslp.2019.2960721
- 发表时间:2020
- 期刊:IEEE/ACM Transactions on Audio, Speech, and Language Processing
- 影响因子:--
- 作者:Jing-Xuan Zhang;Zhen-Hua Ling;Li-Rong Dai
- 通讯作者:Li-Rong Dai
Extracting and Predicting Word-Level Style Variations for Speech Synthesis
提取和预测语音合成的词级风格变化
- DOI:10.1109/taslp.2021.3074757
- 发表时间:2021
- 期刊:IEEE/ACM Transactions on Audio, Speech, and Language Processing
- 影响因子:--
- 作者:Ya-Jie Zhang;Zhen-Hua Ling
- 通讯作者:Zhen-Hua Ling
Sequence-to-Sequence Acoustic Modeling for Voice Conversion
用于语音转换的序列到序列声学建模
- DOI:10.1109/taslp.2019.2892235
- 发表时间:2019-03-01
- 期刊:IEEE-ACM TRANSACTIONS ON AUDIO SPEECH AND LANGUAGE PROCESSING
- 影响因子:5.4
- 作者:Zhang, Jing-Xuan;Ling, Zhen-Hua;Dai, Li-Rong
- 通讯作者:Dai, Li-Rong
UnitNet: A Sequence-to-Sequence Acoustic Model for Concatenative Speech Synthesis
UnitNet:用于串联语音合成的序列到序列声学模型
- DOI:10.1109/taslp.2021.3093823
- 发表时间:2021
- 期刊:IEEE/ACM Transactions on Audio, Speech, and Language Processing
- 影响因子:--
- 作者:Xiao Zhou;Zhen-Hua Ling;Li-Rong Dai
- 通讯作者:Li-Rong Dai
Learning and Modeling Unit Embeddings Using Deep Neural Networks for Unit-Selection-Based Mandarin Speech Synthesis
使用深度神经网络学习和建模单元嵌入,进行基于单元选择的普通话语音合成
- DOI:10.1145/3372244
- 发表时间:2020-01
- 期刊:ACM Transactions on Asian and Low-Resource Language Information Processing
- 影响因子:2
- 作者:Xiao Zhou;Zhen-Hua Ling;Li-Rong Dai
- 通讯作者:Li-Rong Dai
数据更新时间:{{ journalArticles.updateTime }}
{{
item.title }}
{{ item.translation_title }}
- DOI:{{ item.doi || "--"}}
- 发表时间:{{ item.publish_year || "--" }}
- 期刊:{{ item.journal_name }}
- 影响因子:{{ item.factor || "--"}}
- 作者:{{ item.authors }}
- 通讯作者:{{ item.author }}
数据更新时间:{{ journalArticles.updateTime }}
{{ item.title }}
- 作者:{{ item.authors }}
数据更新时间:{{ monograph.updateTime }}
{{ item.title }}
- 作者:{{ item.authors }}
数据更新时间:{{ sciAawards.updateTime }}
{{ item.title }}
- 作者:{{ item.authors }}
数据更新时间:{{ conferencePapers.updateTime }}
{{ item.title }}
- 作者:{{ item.authors }}
数据更新时间:{{ patent.updateTime }}
其他文献
A Synthesis Instance Pruning Approach Based on Virtual Non-uniform Replacements(EI收录)
- DOI:--
- 发表时间:--
- 期刊:Tsinghua Science and Technology
- 影响因子:6.6
- 作者:胡国平;王仁华;张巍;凌震华
- 通讯作者:凌震华
基于目标逼近特征和双向联想贮存器的情感语音基频转换
- DOI:--
- 发表时间:2015
- 期刊:天津大学学报
- 影响因子:--
- 作者:凌震华;高丽;戴礼荣
- 通讯作者:戴礼荣
基于Viterbi 解码的中文合成音库韵律短语边界自动标注
- DOI:--
- 发表时间:--
- 期刊:清华大学学报(自然科学版)
- 影响因子:--
- 作者:杨辰雨;朱立新;凌震华;戴礼荣
- 通讯作者:戴礼荣
其他文献
{{
item.title }}
{{ item.translation_title }}
- DOI:{{ item.doi || "--" }}
- 发表时间:{{ item.publish_year || "--"}}
- 期刊:{{ item.journal_name }}
- 影响因子:{{ item.factor || "--" }}
- 作者:{{ item.authors }}
- 通讯作者:{{ item.author }}
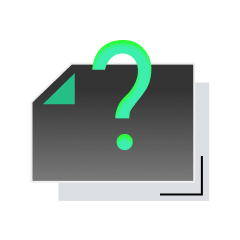
内容获取失败,请点击重试
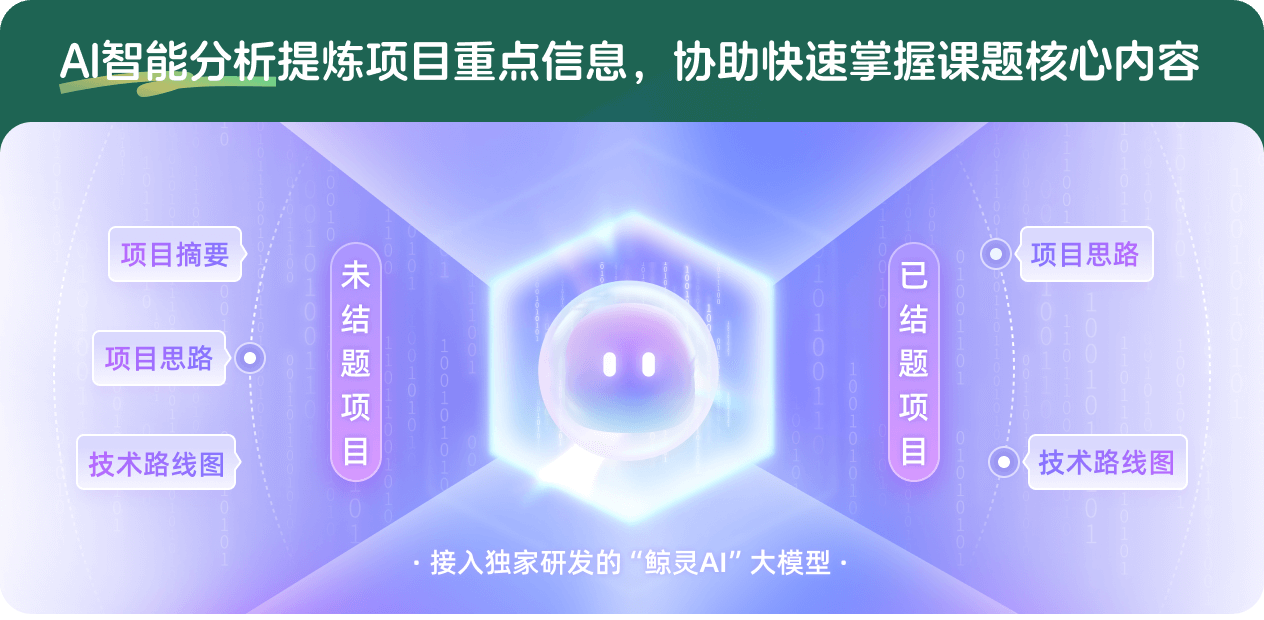
查看分析示例
此项目为已结题,我已根据课题信息分析并撰写以下内容,帮您拓宽课题思路:
AI项目摘要
AI项目思路
AI技术路线图
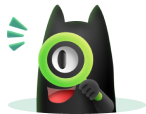
请为本次AI项目解读的内容对您的实用性打分
非常不实用
非常实用
1
2
3
4
5
6
7
8
9
10
您认为此功能如何分析更能满足您的需求,请填写您的反馈:
凌震华的其他基金
融合语音产生机理与统计声学建模的层次化语音合成方法研究
- 批准号:61273032
- 批准年份:2012
- 资助金额:80.0 万元
- 项目类别:面上项目
结合发音动作参数的统计建模语音合成方法研究
- 批准号:60905010
- 批准年份:2009
- 资助金额:19.0 万元
- 项目类别:青年科学基金项目
相似国自然基金
{{ item.name }}
- 批准号:{{ item.ratify_no }}
- 批准年份:{{ item.approval_year }}
- 资助金额:{{ item.support_num }}
- 项目类别:{{ item.project_type }}
相似海外基金
{{
item.name }}
{{ item.translate_name }}
- 批准号:{{ item.ratify_no }}
- 财政年份:{{ item.approval_year }}
- 资助金额:{{ item.support_num }}
- 项目类别:{{ item.project_type }}