染色体突变区间的识别算法研究
项目介绍
AI项目解读
基本信息
- 批准号:61373048
- 项目类别:面上项目
- 资助金额:73.0万
- 负责人:
- 依托单位:
- 学科分类:F0201.计算机科学的基础理论
- 结题年份:2017
- 批准年份:2013
- 项目状态:已结题
- 起止时间:2014-01-01 至2017-12-31
- 项目参与者:杜朴风; 郭菲; 崔文娟; 马文姬; 邓飞; 范莹; 申超;
- 关键词:
项目摘要
Mutation region detection is done via linkage analysis. Today linkage analysis serves as a way of identifying disease causal mutations. Linkage studies have facilitated the identification of several hundred human genes that can harbor mutations that by themselves lead to a disease phenotype. The fundamental problem in linkage analysis is to identify regions whose allele is shared by all or most affected members but by none or few unaffected family members based on the genotype data of a set of individuals. Several famous programs have been developed for linkage analysis when the pedigree within three generations is known and there are enough number of individuals in the pedigree. Those programs can give very accuracy outputs under many but not all cases. Very often, in a pedigree of three generations, there are few number of individuals whose genotype data are available. In this case, the existing methods do not work.To solve the problem, people try to include more individuals in a pedigree of 6 or more generations. The problem becomes mich harder in this case since the genotype data of individuals in the earlier generations cannot be obtained and the relationship between the individuals with genpotype data are far away. To our knowledge, no algorithm can give good outputs when the sampled individuals share common ancesters six generations ago. With the new development of microarray techniques, high-density SNP genotype data can be used for large-scale and cost-effective linkage analysis. Recently, the international HapMap project has produced enormous amount of haplotype data for individuals in some major populations. These new developments make it possible for us to propose new mathematical models for finding genes causing genetic diseases when the sampled individuals share common ancesters six generations ago. This project emphasizes algorithmic issues for all the proposed computational problems. We will also try to implement our designed algorithms to form aoftware packages that work well in practice.
在遗传学上,染色体突变区间的确定通常是通过连锁分析(linkage analysis)进行的。这一方法的应用,已经获知了几百种由自身的基因变异引起的显性疾病。连锁分析的方法是根据一组个体的基因型遗传标记(genotype data)来推断致病基因在染色体中所在的区间。若数量足够多的一组个体是在一个近三代家谱中,已经有有效的实用程序可以解决。在某些情况下,一个近三代的家谱中只能找到少数几个人的基因型标记数据。因此,现有方法无法解决此问题。解决的方法之一是利用更多个体的基因型标记。因此,我们要考虑具有六代或以上的共同祖先的一组个体。然而,在此情况下,问题就变得复杂。在这样大的家谱中,很多先人的基因型标记数据已无法获得,而个体之间的血缘关系变得疏远。目前,尚无任何算法可解决此问题。在此项目中,我们将根据国际人类基因组单体型图计划所得到的数据提出新的数学模型解决此问题。
结项摘要
我们按照项目计划书对染色体区间识别的算法进行了研究。作为该课题的扩展延伸,我们进行了关于单倍型组装问题的研究。另外,我们也对其他相关研究方向,例如,泛基因组比较的研究,蛋白质结构位点预测,阻塞模式匹配问题的研究及谱系比较的研究。, .共在国际学术期刊发表论文11篇。.有几个算法已转换成计算机软件。.我们还成功举办了COCOA2016 及APBC 2017两个国际会议。.此外,我们正在筹划Cocoon2018及CPM2018国际会议。将于2018年7月2日至7月4日于青岛同时举行。.我们参加了几个国际会议并发表了论文。.我们与日本东京电器大学(ZHENZHi-Zhong 教授),加拿大阿尔伯特大学计算机系(LIN Guohui教授, 澳大利亚悉尼科技大(LI Jinyan教授),山东大学(朱大铭教授),天津大学(郭菲副教授),中南大学(王建新教授,李敏教授)合作并联合发表了论文。
项目成果
期刊论文数量(11)
专著数量(0)
科研奖励数量(0)
会议论文数量(0)
专利数量(0)
Approximation algorithms for the scaffolding problem and its generalization
脚手架问题的近似算法及其泛化
- DOI:--
- 发表时间:2017
- 期刊:Theoretical Computer Science
- 影响因子:1.1
- 作者:Zhi-Zhong Chen;Youta Harada;Fei Guo;Lusheng WANG
- 通讯作者:Lusheng WANG
Better ILP-Based Approaches to Haplotype Assembly
更好的基于 ILP 的单倍型组装方法
- DOI:10.1089/cmb.2015.0035
- 发表时间:2016-07-01
- 期刊:JOURNAL OF COMPUTATIONAL BIOLOGY
- 影响因子:1.7
- 作者:Chen, Zhi-Zhong;Deng, Fei;Wang, Lusheng
- 通讯作者:Wang, Lusheng
Randomized Fixed-Parameter Algorithms for the Closest String Problem
最近串问题的随机固定参数算法
- DOI:10.1007/s00453-014-9952-y
- 发表时间:2014
- 期刊:Algorithmica
- 影响因子:1.1
- 作者:Chen Zhi-Zhong;Ma Bin;Wang Lusheng
- 通讯作者:Wang Lusheng
Algorithms for Pedigree Comparison
谱系比较算法
- DOI:10.1109/tcbb.2016.2550434
- 发表时间:2018-03-01
- 期刊:IEEE-ACM TRANSACTIONS ON COMPUTATIONAL BIOLOGY AND BIOINFORMATICS
- 影响因子:4.5
- 作者:Chen,Zhi-Zhong;Feng,Qilong;Wang,Lusheng
- 通讯作者:Wang,Lusheng
An approximation algorithm for maximum internal spanning tree
一种最大内生成树的近似算法
- DOI:10.1007/s10878-017-0245-7
- 发表时间:2016-07
- 期刊:Journal of Combinatorial Optimization
- 影响因子:1
- 作者:Zhi-Zhong Chen;Youta Harada;Fei Guo;Lusheng Wang
- 通讯作者:Lusheng Wang
数据更新时间:{{ journalArticles.updateTime }}
{{
item.title }}
{{ item.translation_title }}
- DOI:{{ item.doi || "--"}}
- 发表时间:{{ item.publish_year || "--" }}
- 期刊:{{ item.journal_name }}
- 影响因子:{{ item.factor || "--"}}
- 作者:{{ item.authors }}
- 通讯作者:{{ item.author }}
数据更新时间:{{ journalArticles.updateTime }}
{{ item.title }}
- 作者:{{ item.authors }}
数据更新时间:{{ monograph.updateTime }}
{{ item.title }}
- 作者:{{ item.authors }}
数据更新时间:{{ sciAawards.updateTime }}
{{ item.title }}
- 作者:{{ item.authors }}
数据更新时间:{{ conferencePapers.updateTime }}
{{ item.title }}
- 作者:{{ item.authors }}
数据更新时间:{{ patent.updateTime }}
其他文献
其他文献
{{
item.title }}
{{ item.translation_title }}
- DOI:{{ item.doi || "--" }}
- 发表时间:{{ item.publish_year || "--"}}
- 期刊:{{ item.journal_name }}
- 影响因子:{{ item.factor || "--" }}
- 作者:{{ item.authors }}
- 通讯作者:{{ item.author }}
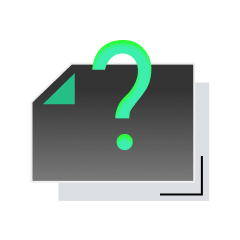
内容获取失败,请点击重试
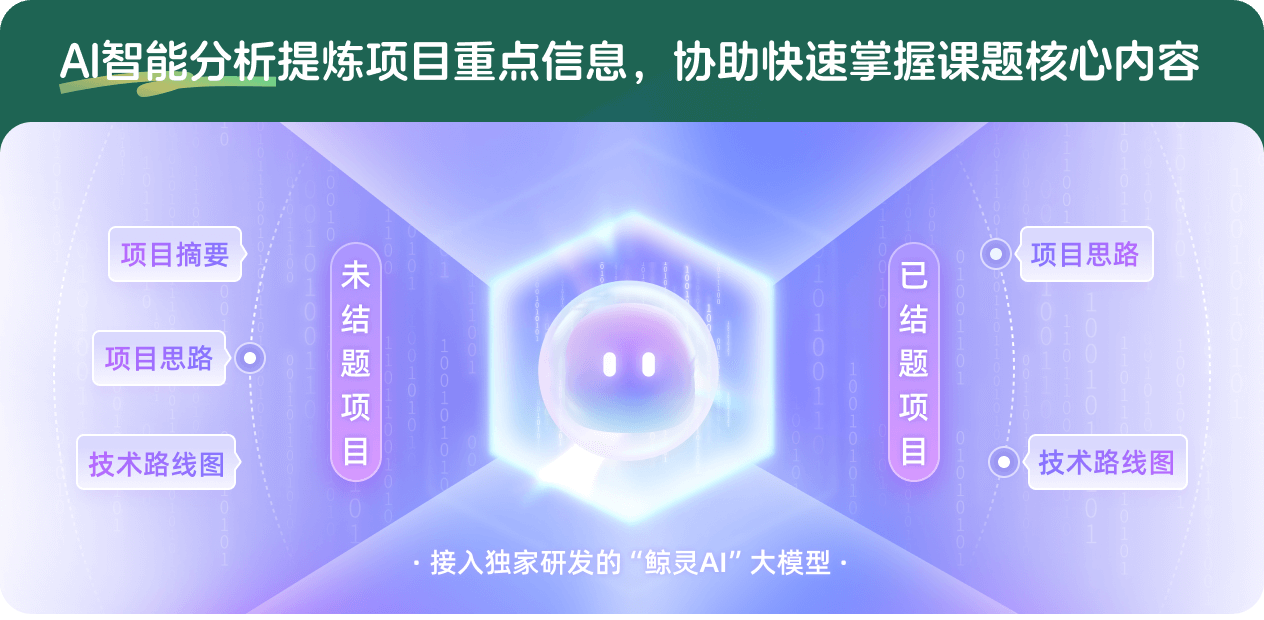
查看分析示例
此项目为已结题,我已根据课题信息分析并撰写以下内容,帮您拓宽课题思路:
AI项目摘要
AI项目思路
AI技术路线图
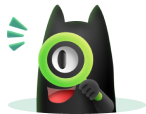
请为本次AI项目解读的内容对您的实用性打分
非常不实用
非常实用
1
2
3
4
5
6
7
8
9
10
您认为此功能如何分析更能满足您的需求,请填写您的反馈:
王鲁生的其他基金
关于某些树的问题,图的平均划分问题,及蛋白质变体质量图的算法设计
- 批准号:61972329
- 批准年份:2019
- 资助金额:60 万元
- 项目类别:面上项目
相似国自然基金
{{ item.name }}
- 批准号:{{ item.ratify_no }}
- 批准年份:{{ item.approval_year }}
- 资助金额:{{ item.support_num }}
- 项目类别:{{ item.project_type }}
相似海外基金
{{
item.name }}
{{ item.translate_name }}
- 批准号:{{ item.ratify_no }}
- 财政年份:{{ item.approval_year }}
- 资助金额:{{ item.support_num }}
- 项目类别:{{ item.project_type }}