面向医学特定疾病的问题分析和相似度计算模型研究
项目介绍
AI项目解读
基本信息
- 批准号:61772146
- 项目类别:面上项目
- 资助金额:67.0万
- 负责人:
- 依托单位:
- 学科分类:F0211.信息检索与社会计算
- 结题年份:2021
- 批准年份:2017
- 项目状态:已结题
- 起止时间:2018-01-01 至2021-12-31
- 项目参与者:陈仕鸿; 瞿瑛瑛; 卢达威; 王敏; 刘罡; 徐凯; 谢文秀; 陈协玲; 陈柏宇;
- 关键词:
项目摘要
As an interdisciplinary field between Natural Language Processing and Information Retrieval, Question Answering (QA) has drawn more and more attention and became an emerging research hotspot for providing exact answer service. However, the disease-specific QA research has difficulties due to the complexity of text analysis and information extraction in medical domain. Therefore, targeting at chronic diseases in medical domain, this project carries out fundamental research topics of automatic question answering. With the focus on Diabetes Mellitus, a growing public health problem throughout the world, this project conducts the research on: medical question target identification and classification, medical quantitative statement representation model and extraction method, and question similarity calculation based on question target feature matching and equivalent semantic pattern linking. In addition, the project grabs heterogeneous Diabetes QA pairs and constructs a large scale multi-lingual Diabetes QA corpus for public use, which will eventually serve disease-specific medical QA systems. We believe that the project will be of a positive significance to the basic and practical research on intelligent QA techniques towards major medical diseases, and meanwhile, embodying its social significance and application value to the diabetic in providing treatment information, improving prevention ability of the high-risk population in early-stage, and enhancing daily self-health management.
问答系统以提供精准答案为目标,已成为自然语言处理与信息检索领域的一个重要分支和新兴研究热点。在医学领域中,由于医学文本分析和信息抽取的复杂性,面向医学特定疾病的问答系统研究还存在诸多困难。本项目将围绕医学领域重大慢性疾病,开展医学自动问答的基础科学研究,并以糖尿病这一世界性公共健康问题为出发点,开展医学问题目标的识别与分类方法研究、医学文本数量表达式的表示模型与抽取方法研究、以及基于问题目标特征匹配和等价语义模板关联的医学问题相似度计算模型研究。此外,项目将采集多源异构糖尿病问答数据构建大规模多语言糖尿病FAQ语料库并实现开放使用,最终服务于面向医学特定疾病的自动问答。研究将对面向医学重大疾病的智能问答技术基础研究和实用化研究具有积极的科学意义,并在提供糖尿病人群的防治信息服务、提高糖尿病前期高危人群的预防能力、提升糖尿病患者的自身健康监控等多个方面具有潜在社会意义和应用价值。
结项摘要
由于医学文本分析和信息抽取的复杂性,面向医学特定疾病的问答系统研究还存在诸多困难,本项目围绕医学领域重大慢性疾病,开展医学自动问答的基础和应用科学研究,包括医学问题目标的识别与分类方法、医学非结构化文本的信息抽取方法、医学文本数量表达式的表示与抽取方法、基于问题目标特征匹配和等价语义模板关联的医学问题相似度计算模型、以及领域知识图谱表示学习模型等研究。通过项目的开展,提出了基于混合神经网络的问题目标分类模型、基于深度学习的疾病命名实体抽取方法、基于模式学习的时间表达式抽取方法、基于多模式融合的问答系统框架、融合邻居语义知识的选择对抗网络等新技术、新模型,通过实验对比验证了有效性,并构建一个超过10万问答对的糖尿病常见问题语料库,用于医疗领域问答任务。本项目共计发表30篇学术论文,均为SCI/EI索引(19篇SCI、11篇EI),其中4篇中科院大类一二区论文,并申请了1项ISO国际标准、4项技术发明专利,通过项目研究,培养了20余名博士、硕士、本科生参与课题研究和实践。项目完成并超出了预期的研究目标,对面向医学领域的智能问答技术基础研究和实用化研究具有积极的科学意义和应用价值。
项目成果
期刊论文数量(19)
专著数量(0)
科研奖励数量(0)
会议论文数量(11)
专利数量(4)
Clinical quantitative information recognition and entity-quantity association from Chinese electronic medical records
中国电子病历的临床定量信息识别及实体量关联
- DOI:10.1007/s13042-020-01160-0
- 发表时间:2020-08
- 期刊:International Journal of Machine Learning and Cybernetics
- 影响因子:5.6
- 作者:Liu Shanshan;Nie Wenjie;Gao Dongfa;Yang Hao;Yan Jun;Hao Tianyong
- 通讯作者:Hao Tianyong
A bibliometric analysis of natural language processing in medical research.
医学研究中自然语言处理的文献计量分析
- DOI:10.1186/s12911-018-0594-x
- 发表时间:2018-03-22
- 期刊:BMC medical informatics and decision making
- 影响因子:3.5
- 作者:Chen X;Xie H;Wang FL;Liu Z;Xu J;Hao T
- 通讯作者:Hao T
Automated classification of clinical trial eligibility criteria text based on ensemble learning and metric learning.
基于集成学习和度量学习的临床试验资格标准文本自动分类
- DOI:10.1186/s12911-021-01492-z
- 发表时间:2021-07-30
- 期刊:BMC medical informatics and decision making
- 影响因子:3.5
- 作者:Zeng K;Xu Y;Lin G;Liang L;Hao T
- 通讯作者:Hao T
A Hybrid Neural Network BERT-Cap Based on Pre-Trained Language Model and Capsule Network for User Intent Classification
基于预训练语言模型和胶囊网络的混合神经网络 BERT-Cap 用于用户意图分类
- DOI:10.1155/2020/8858852
- 发表时间:2020-11
- 期刊:Complexity
- 影响因子:2.3
- 作者:Liu Hai;Liu Yuanxia;Wong Leung-Pun;Lee Lap-Kei;Hao Tianyong
- 通讯作者:Hao Tianyong
Learning knowledge graph embedding with a bi-directional relation encoding network and a convolutional autoencoder decoding network
使用双向关系编码网络和卷积自动编码器解码网络学习知识图嵌入
- DOI:10.1007/s00521-020-05654-4
- 发表时间:2021-01
- 期刊:Neural Computing and Applications
- 影响因子:6
- 作者:Kairong Hu;Hai Liu;Choujun Zhan;Yong Tang;Tianyong Hao
- 通讯作者:Tianyong Hao
数据更新时间:{{ journalArticles.updateTime }}
{{
item.title }}
{{ item.translation_title }}
- DOI:{{ item.doi || "--"}}
- 发表时间:{{ item.publish_year || "--" }}
- 期刊:{{ item.journal_name }}
- 影响因子:{{ item.factor || "--"}}
- 作者:{{ item.authors }}
- 通讯作者:{{ item.author }}
数据更新时间:{{ journalArticles.updateTime }}
{{ item.title }}
- 作者:{{ item.authors }}
数据更新时间:{{ monograph.updateTime }}
{{ item.title }}
- 作者:{{ item.authors }}
数据更新时间:{{ sciAawards.updateTime }}
{{ item.title }}
- 作者:{{ item.authors }}
数据更新时间:{{ conferencePapers.updateTime }}
{{ item.title }}
- 作者:{{ item.authors }}
数据更新时间:{{ patent.updateTime }}
其他文献
面向知识建构的课堂对话规律探析
- DOI:--
- 发表时间:2021
- 期刊:电化教育研究
- 影响因子:--
- 作者:宋宇;邬宝娴;郝天永
- 通讯作者:郝天永
学习分析视角下培养高阶思维的课堂互动研究
- DOI:--
- 发表时间:2020
- 期刊:现代教育技术
- 影响因子:--
- 作者:宋宇;郝天永;刘葵
- 通讯作者:刘葵
其他文献
{{
item.title }}
{{ item.translation_title }}
- DOI:{{ item.doi || "--" }}
- 发表时间:{{ item.publish_year || "--"}}
- 期刊:{{ item.journal_name }}
- 影响因子:{{ item.factor || "--" }}
- 作者:{{ item.authors }}
- 通讯作者:{{ item.author }}
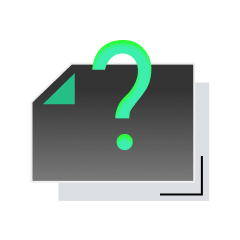
内容获取失败,请点击重试
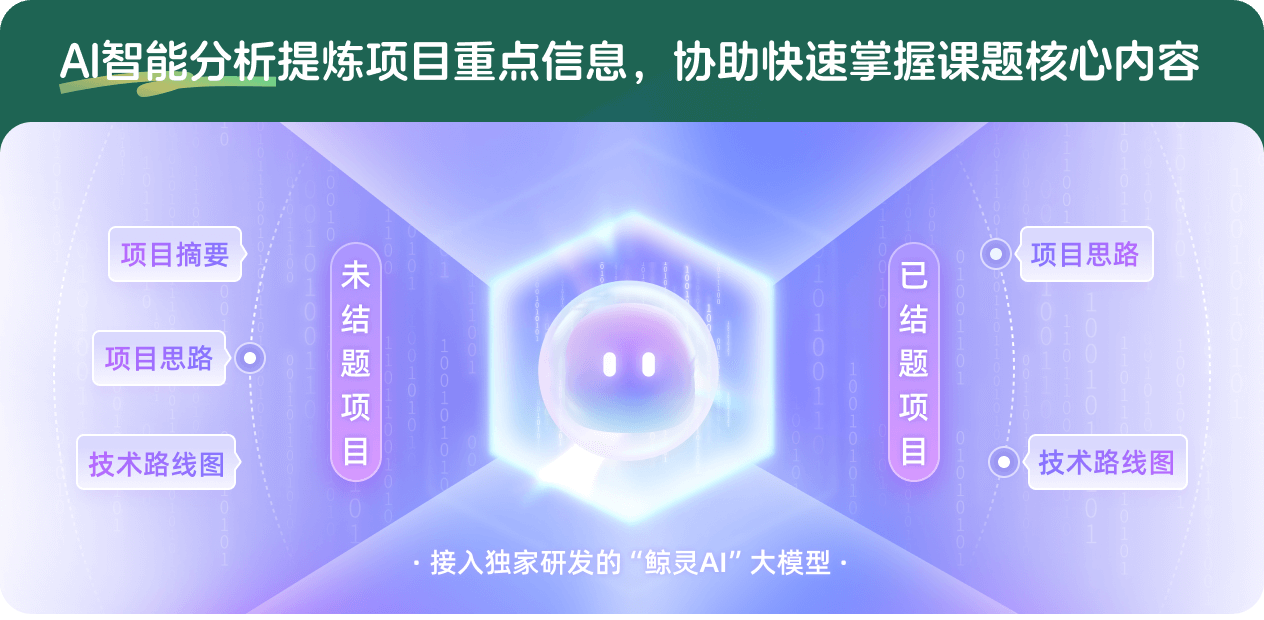
查看分析示例
此项目为已结题,我已根据课题信息分析并撰写以下内容,帮您拓宽课题思路:
AI项目摘要
AI项目思路
AI技术路线图
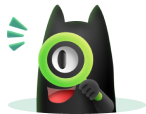
请为本次AI项目解读的内容对您的实用性打分
非常不实用
非常实用
1
2
3
4
5
6
7
8
9
10
您认为此功能如何分析更能满足您的需求,请填写您的反馈:
郝天永的其他基金
医学文本可度量数量信息语义表示与信息抽取关键技术研究
- 批准号:62372189
- 批准年份:2023
- 资助金额:50 万元
- 项目类别:面上项目
相似国自然基金
{{ item.name }}
- 批准号:{{ item.ratify_no }}
- 批准年份:{{ item.approval_year }}
- 资助金额:{{ item.support_num }}
- 项目类别:{{ item.project_type }}
相似海外基金
{{
item.name }}
{{ item.translate_name }}
- 批准号:{{ item.ratify_no }}
- 财政年份:{{ item.approval_year }}
- 资助金额:{{ item.support_num }}
- 项目类别:{{ item.project_type }}