流形循环可微分编程网络的边缘计算及其在肺癌实时预测中的研究
项目介绍
AI项目解读
基本信息
- 批准号:61871186
- 项目类别:面上项目
- 资助金额:63.0万
- 负责人:
- 依托单位:
- 学科分类:F0125.医学信息检测与处理
- 结题年份:2022
- 批准年份:2018
- 项目状态:已结题
- 起止时间:2019-01-01 至2022-12-31
- 项目参与者:何志海; 侯凯; 胡国祥; 郭姝男; 靳远远; 李岩; 黄甜甜; 朱霄雯;
- 关键词:
项目摘要
Lung cancer is the malignant tumor with the highest death rate in the world,and early diagnosis and treatment can prolong the patient's survival. For early pulmonary nodules, especially when the diameter is equal or less than 8 mm, it is difficult to assess them using imaging techniques, and it is also impossible to use biopsy for accurate histopathological diagnosis, and thus the misdiagnosis rate is high. In response to this problem, on one hand, manifold-based differentiable programming networks are studied to improve the accuracy and efficiency of lung cancer detection; at the same time, a feedback network based on conformal mapping is studied, and a mapping model for the confirmed diagnosis of lung cancer and follow-up image nodules of the same case is established, which can achieve an early prediction of nodules; on the other hand, edge computing methods are studied to achieve real-time detection and prediction. The main contents include: research on manifold-based convolution methods in differentiable programming networks; research on the initial features of retrospective lung nodule based on differential homeomorphism mapping methods as an enhancement model of the feedback network; research on migration computing methods for edge computing to realize the real-time performance of edge devices on model predicting; retrospection of series of CT images with different follow-up time of confirmed cases for algorithm validation and optimization to achieve early prediction of lung cancer. This study not only has a great breakthrough in the theory of differentiable programming networks and edge computing, but also provides theoretical basis and algorithm design for lung cancer prediction, which has academic significance and application value.
肺癌是世界上死亡率最高的恶性肿瘤,早期诊断与治疗可以延长患者的生存期。对于直径≤8mm的肺结节很难用影像学技术进行评估,也无法用活检进行精确的组织病理学诊断,往往错失医学干预的最好时机。针对这个问题,一方面研究流形可微分编程网络,提高肺癌检测的准确率和效率;同时构建基于共形几何映射的反馈网络,建立确诊肺癌与同一病例之前随访图像结节的映射模型,实现对结节的早期预测。另一方面研究网络边缘模型,实现检测和预测的实时性。主要内容包括:研究可微分编程网络中基于流形的卷积方法;研究微分同胚共形映射以追溯肺癌结节初始特征及反馈网络增强模型;研究网络边缘模型的迁移计算方法,提高模型推断的实时性;对肺癌结节不同随访时间的系列CT图像进行算法实时验证和优化,实现肺癌的早期预测。本项目不仅能在可微分编程网络和边缘迁移计算理论上有较大突破,对肺癌的早期辅助诊断提供理论依据与技术,具有学术意义和应用价值。
结项摘要
本项目对流形循环可微分编程网络、共形映射及反馈增强网络、边缘计算以及肺结节实时检测和预测进行了深入研究。主要工作包括:.流形可微分编程网络:1)网络设计。提出了通过仿射弧模型化的流形深度学习网络、基于可微分构件的深度神经网络、不确定性形状感知的多任务学习模型等;提出了语义隐空间方向反事实生成方法等,对模型的可解释性进行了研究。2)流形变结构核函数构造。基于群论概念,将传统的卷积核改造成群卷积核,提出了等变注意力机制来动态聚合多组群卷积核、群结构化变分数据填充网络来估计图像的缺失数据;提出了局部流形判别分析卷积核、高约束高分散主成分卷积核,以发现图像流形潜在的局部结构。3)图像特征的哈希表达及分类。提出了自适应加权多通道中心相似深度哈希算法、基于反向自动编码器的深度聚类模型,提取丰富的语义信息。.共形映射及反馈增强网络:1)共形映射方法。提出了基于空间变换进行肺结节生长建模的肺癌预测算法,充分利用前期随访数据提高诊断准确性;提出了基于节点重要性的图分类数据增强方法。2)反馈与强化机制。提出了多策略互学习网络、基于形状感知的多任务学习模型,提高模型精度;提出了基于混合一致交叉表征、对抗判别器的强化学习泛化性能提升方法。.边缘计算模型:1)模型设计。研究了基于FPGA加速的纵向联邦学习算法。2)计算资源管理。提出了异质物联网边缘联邦学习高效训练的调度和资源管理算法。3)迁移计算。提出了基于原型网络的对抗域适应方法,提高对目标域的预测精度。.利用本项目成果并参与开发的肺结节检测系统,已经被全国10多家三甲医院采购使用。在国内外核心学术期刊和重要国际会议上发表学术论文28篇,发表专著2部;授权发明专利1项、申请6项;授权软件著作权8项。培养博士毕业生1名、硕士毕业生14名;在读博士生3名、在读硕士生8名。
项目成果
期刊论文数量(11)
专著数量(2)
科研奖励数量(0)
会议论文数量(17)
专利数量(7)
Adaptive weight multi-channel center similar deep hashing
自适应权重多通道中心相似深度哈希
- DOI:10.1016/j.jvcir.2022.103642
- 发表时间:2022-09
- 期刊:Journal of Visual Communication and Image Representation
- 影响因子:2.6
- 作者:Xinghua Liu;Guitao Cao;Qiubin Lin;Wenming Cao
- 通讯作者:Wenming Cao
Invariants of the Space Point Element Structure and Their Applications
空间点元结构不变量及其应用
- DOI:10.1155/2020/3295492
- 发表时间:2020-10
- 期刊:Mathematical Problems in Engineering
- 影响因子:--
- 作者:Yanping Mui;Youzheng Zhang;Guitao Cao
- 通讯作者:Guitao Cao
Implicit user relationships across sessions enhanced graph for session-based recommendation
跨会话的隐式用户关系增强了基于会话的推荐图表
- DOI:10.1016/j.ins.2022.07.062
- 发表时间:2022-07
- 期刊:Information Sciences
- 影响因子:8.1
- 作者:Wenming Cao;Yishan Liu;Guitao Cao;Zhiquan He
- 通讯作者:Zhiquan He
Client Scheduling and Resource Management for Efficient Training in Heterogeneous IoT-Edge Federated Learning
用于异构物联网边缘联合学习高效培训的客户端调度和资源管理
- DOI:10.1109/tcad.2021.3110743
- 发表时间:2022-08
- 期刊:IEEE Transactions on Computer-Aided Design of Integrated Circuits and Systems
- 影响因子:2.9
- 作者:Yangguang Cui;Kun Cao;Guitao Cao;Meikang Qiu;Tongquan Wei
- 通讯作者:Tongquan Wei
AHU-MultiNet: Adaptive loss balancing based on homoscedastic uncertainty in multi-task medical image segmentation network
AHU-MultiNet:多任务医学图像分割网络中基于同方差不确定性的自适应损失平衡
- DOI:10.1016/j.compbiomed.2022.106157
- 发表时间:2022-10
- 期刊:Computers in Biology and Medicine
- 影响因子:7.7
- 作者:Shasha Liu;Hailing Wang;Yan Li;Xiaohu Li;Guitao Cao;Wenming Cao
- 通讯作者:Wenming Cao
数据更新时间:{{ journalArticles.updateTime }}
{{
item.title }}
{{ item.translation_title }}
- DOI:{{ item.doi || "--"}}
- 发表时间:{{ item.publish_year || "--" }}
- 期刊:{{ item.journal_name }}
- 影响因子:{{ item.factor || "--"}}
- 作者:{{ item.authors }}
- 通讯作者:{{ item.author }}
数据更新时间:{{ journalArticles.updateTime }}
{{ item.title }}
- 作者:{{ item.authors }}
数据更新时间:{{ monograph.updateTime }}
{{ item.title }}
- 作者:{{ item.authors }}
数据更新时间:{{ sciAawards.updateTime }}
{{ item.title }}
- 作者:{{ item.authors }}
数据更新时间:{{ conferencePapers.updateTime }}
{{ item.title }}
- 作者:{{ item.authors }}
数据更新时间:{{ patent.updateTime }}
其他文献
基于形态学的骨髓病理切片图像中幼稚前体细胞的检测方法
- DOI:--
- 发表时间:--
- 期刊:morphology.2010 Seventh international Conference on Fuzzy Systems and Knowledge Discovery (FSKD 10)
- 影响因子:--
- 作者:石军;李玲;曹桂涛
- 通讯作者:曹桂涛
其他文献
{{
item.title }}
{{ item.translation_title }}
- DOI:{{ item.doi || "--" }}
- 发表时间:{{ item.publish_year || "--"}}
- 期刊:{{ item.journal_name }}
- 影响因子:{{ item.factor || "--" }}
- 作者:{{ item.authors }}
- 通讯作者:{{ item.author }}
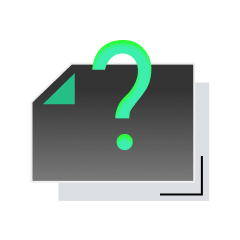
内容获取失败,请点击重试
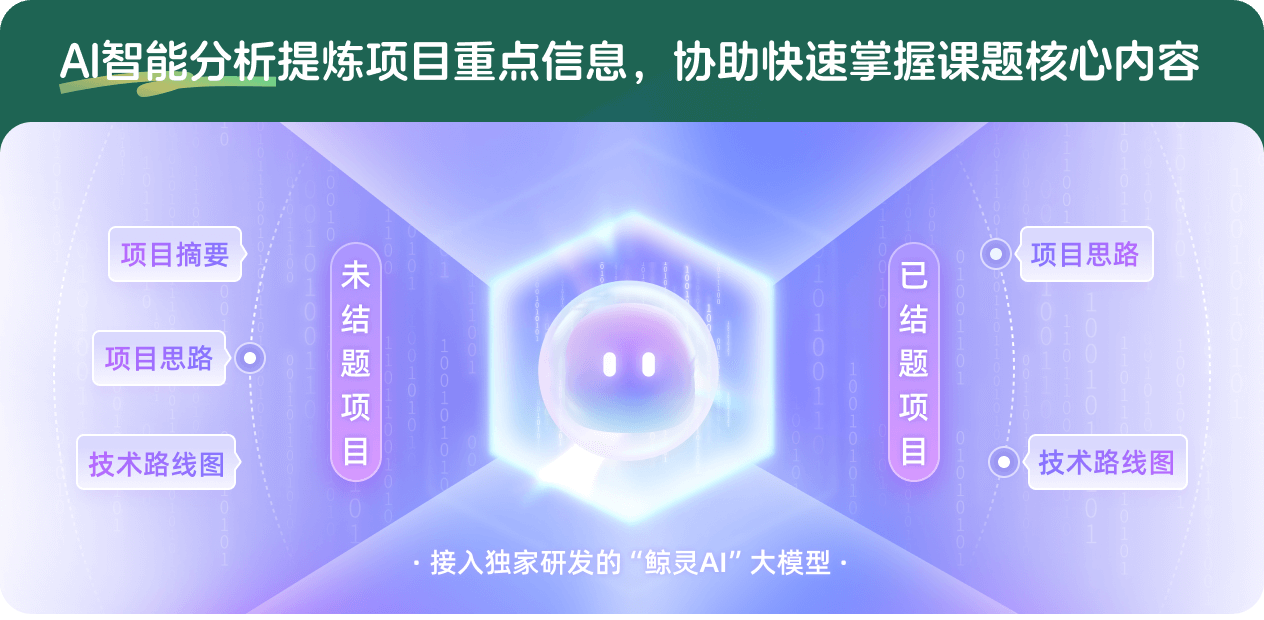
查看分析示例
此项目为已结题,我已根据课题信息分析并撰写以下内容,帮您拓宽课题思路:
AI项目摘要
AI项目思路
AI技术路线图
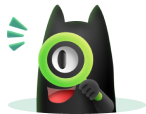
请为本次AI项目解读的内容对您的实用性打分
非常不实用
非常实用
1
2
3
4
5
6
7
8
9
10
您认为此功能如何分析更能满足您的需求,请填写您的反馈:
曹桂涛的其他基金
变化检测驱动的边缘约束条件随机场模型及其迁移学习
- 批准号:61340036
- 批准年份:2013
- 资助金额:17.0 万元
- 项目类别:专项基金项目
相似国自然基金
{{ item.name }}
- 批准号:{{ item.ratify_no }}
- 批准年份:{{ item.approval_year }}
- 资助金额:{{ item.support_num }}
- 项目类别:{{ item.project_type }}
相似海外基金
{{
item.name }}
{{ item.translate_name }}
- 批准号:{{ item.ratify_no }}
- 财政年份:{{ item.approval_year }}
- 资助金额:{{ item.support_num }}
- 项目类别:{{ item.project_type }}