基于深度判别特征学习的SAR图像地物分类
项目介绍
AI项目解读
基本信息
- 批准号:61703332
- 项目类别:青年科学基金项目
- 资助金额:28.0万
- 负责人:
- 依托单位:
- 学科分类:F0604.机器感知与机器视觉
- 结题年份:2020
- 批准年份:2017
- 项目状态:已结题
- 起止时间:2018-01-01 至2020-12-31
- 项目参与者:曹子建; 李红叶; 邹广昆; 王健; 成耐克;
- 关键词:
项目摘要
The synthetic aperture radar (SAR) is an active detection system with long distance, all-weather and timeless imaging capacity, which has been widely used in a variety of civil and military areas. As one typical task of SAR image interpretation, the land-cover classification has attracted great attention of many researchers in the fields of photography, computer vision, earth science and remote sensing. However, the quality of SAR image is degraded serious by a large amount of radar shadow, speckle and blurring. Therefore, it is a difficult task to capture accurate and discriminant features of different SAR image land-covers. To handle such a difficult problem, this project focuses on learning discriminant features of SAR images based on the latest research results in computer vision and deep learning. Specifically, the key research points of this project are listed as follows. Firstly, a novel statistical model of SAR image is provided by casting the deep belief network (DBN) as a generative graphical model with multiple layers of statistical, latent variables. Based on DBN and generalized Gamma distribution, parameters of the proposed model are easy to estimate. Secondly, a new deep metric learning approach is proposed to compute the similarity between SAR images based on the theory of deep learning. And finally, a classification-related feature extraction approach is studied in the third part of this project. Based on the proposed statistical model and similarity metric, the discriminant information of different land-covers could be acquired by combing with prototype theory in cognitive science in this part. The study of this project aims to enrich the theory systems of feature extraction and learning for SAR image. With the learnt discriminant features, difficulties of land-cover classification could be overcome effectively With the implementation of this project, it hopes to provide a solid theoretical basis and feasible method guidance for discriminant feature learning of SAR images in practical.
受成像系统相干特性的影响,SAR图像中存在大量的相干斑、旁瓣、雷达阴影及模糊等现象,这使得在地物分类任务中极难获取不同地物的判别性特征表示。针对该问题,结合计算机视觉及深度学习最新的研究成果,将重点研究SAR图像中判别性特征的学习方法。本项目拟开展以下三个方面的研究工作。一、基于深度信念网,对SAR图像提供一个新的、易于参数估计的统计分布建模方法;二、结合深度学习理论构建一个深层相似性测度学习方法,以对SAR图像之间的相似性进行计算;三、在统计模型及相似性计算的基础上,结合认知学中原型理论,对SAR图像中各类地物的判别性信息进行提取。该项目的研究旨在丰富SAR图像特征提取及学习方法的理论体系和技术手段,实现对SAR图像中不同类型地物进行判别性描述,以克服由成像特性对地物分类所带来的困难。通过本项目的实施,以期为实际应用中对SAR图像进行判别性特征学习问题提供坚实的理论依据和可行的方法指导。
结项摘要
对SAR图像来说,由于受其特殊成像机理、复杂观测场景及解译目标多样性等因素的影响,使得对SAR图像进行自动及半自动地处理及分析是一项极其困难的任务。本项目在对SAR图像特征提取及学习技术发展现状分析的基础上,结合计算机视觉和机器学习领域最新研究成果及技术手段,对SAR图像判别特征学习方法进行系统深入研究,进而获取不同地物的有效表示。具体地,本项目首先基于广义伽马分布,结合深度信念网络模型,提出了一个新的、易于参数估计统计分布模型对SAR图像中不同类型地物进行统计建模;其次,在统计建模的基础上,集合深度学习理论,构建深度相似性测度学习方法,用于对对两幅SAR图像、两个像素点或者这两个局部块之间的相似性测度学习方法;最后,在已有工作的基础上,本项目针对地物分类这一典型应用场景,进而对不同地物目标设计任务相关性的判别特征表示。项目组在已有SAR图像数据基础上对这三个问题展开研究工作,首先在对SAR图像强度值统计分布利用广义伽马混合分布模型进行统计建模之后,提出广义伽马—伯努利受限玻尔兹曼机,并将其与多个伯努利—伯努利受限玻尔兹曼机堆叠之后,构造了一个基于广义伽马分布的深度信念网络对SAR图像中不同地物进行表示;此外,在对高分辨率SAR图像强度值统计分布的基础上计算Fisher向量,基于此,提出了多尺度局部Fisher模式用于货物不同地物对象的判别性信息;最后,项目组通过在Dempster-Shafer证据理论中引入全局及局部性先验信息对经典期望最大化方法进行改进,提出一种对时序SAR图像中变化特性有效的检测方法。通过大量的机载和星载SAR图像进行实验验证,结果表明这些方法可以有效克服在SAR图像中由于图像分析人员背景知识的差异以及对成像区域先验知识的缺乏,无法保证他们对不同类型地物特征的提取的一致性问题。通过本项目的研究,可为SAR图像中不同类型地物及目标的快速、自动特征提取提供一定的理论依据和技术支持。
项目成果
期刊论文数量(3)
专著数量(0)
科研奖励数量(0)
会议论文数量(2)
专利数量(0)
The Generalized Gamma-DBN for High-Resolution SAR Image Classification
用于高分辨率 SAR 图像分类的广义 Gamma-DBN
- DOI:10.3390/rs10060878
- 发表时间:2018-06-01
- 期刊:REMOTE SENSING
- 影响因子:5
- 作者:Zhao, Zhiqiang;Guo, Lei;Wang, Lei
- 通讯作者:Wang, Lei
Incorporating global-local a Priori knowledge into expectation-maximization for SAR image change detection
将全局局部先验知识纳入 SAR 图像变化检测的期望最大化
- DOI:10.1080/01431161.2018.1519276
- 发表时间:2019-01-17
- 期刊:INTERNATIONAL JOURNAL OF REMOTE SENSING
- 影响因子:3.4
- 作者:Jia, Meng;Zhao, ZhiQiang;Qiu, Yuan
- 通讯作者:Qiu, Yuan
数据更新时间:{{ journalArticles.updateTime }}
{{
item.title }}
{{ item.translation_title }}
- DOI:{{ item.doi || "--"}}
- 发表时间:{{ item.publish_year || "--" }}
- 期刊:{{ item.journal_name }}
- 影响因子:{{ item.factor || "--"}}
- 作者:{{ item.authors }}
- 通讯作者:{{ item.author }}
数据更新时间:{{ journalArticles.updateTime }}
{{ item.title }}
- 作者:{{ item.authors }}
数据更新时间:{{ monograph.updateTime }}
{{ item.title }}
- 作者:{{ item.authors }}
数据更新时间:{{ sciAawards.updateTime }}
{{ item.title }}
- 作者:{{ item.authors }}
数据更新时间:{{ conferencePapers.updateTime }}
{{ item.title }}
- 作者:{{ item.authors }}
数据更新时间:{{ patent.updateTime }}
其他文献
二维材料曲面生长:力学与化学的邂逅
- DOI:10.7511/jslx20210226001
- 发表时间:2021
- 期刊:计算力学学报
- 影响因子:--
- 作者:胡知力;薛敏珉;赵志强;张助华
- 通讯作者:张助华
职业因素暴露对心血管系统的影响
- DOI:--
- 发表时间:2015
- 期刊:中国慢性病预防与控制
- 影响因子:--
- 作者:赵志强;何云
- 通讯作者:何云
生态系统服务研究动态及地理学研究范式
- DOI:--
- 发表时间:2011
- 期刊:地理学报
- 影响因子:--
- 作者:李双成;刘金龙;张才玉;赵志强
- 通讯作者:赵志强
钢球展开机构的接触碰撞特性
- DOI:--
- 发表时间:2015
- 期刊:哈尔滨理工大学学报
- 影响因子:--
- 作者:赵彦玲;李积才;赵志强;王弘博
- 通讯作者:王弘博
基于改进暗目标法山区HJ CCD影像气溶胶光学厚度反演
- DOI:--
- 发表时间:2015
- 期刊:光谱学与光谱分析
- 影响因子:--
- 作者:赵志强;李爱农;边金虎;黄成全
- 通讯作者:黄成全
其他文献
{{
item.title }}
{{ item.translation_title }}
- DOI:{{ item.doi || "--" }}
- 发表时间:{{ item.publish_year || "--"}}
- 期刊:{{ item.journal_name }}
- 影响因子:{{ item.factor || "--" }}
- 作者:{{ item.authors }}
- 通讯作者:{{ item.author }}
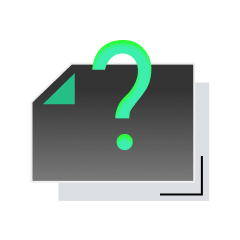
内容获取失败,请点击重试
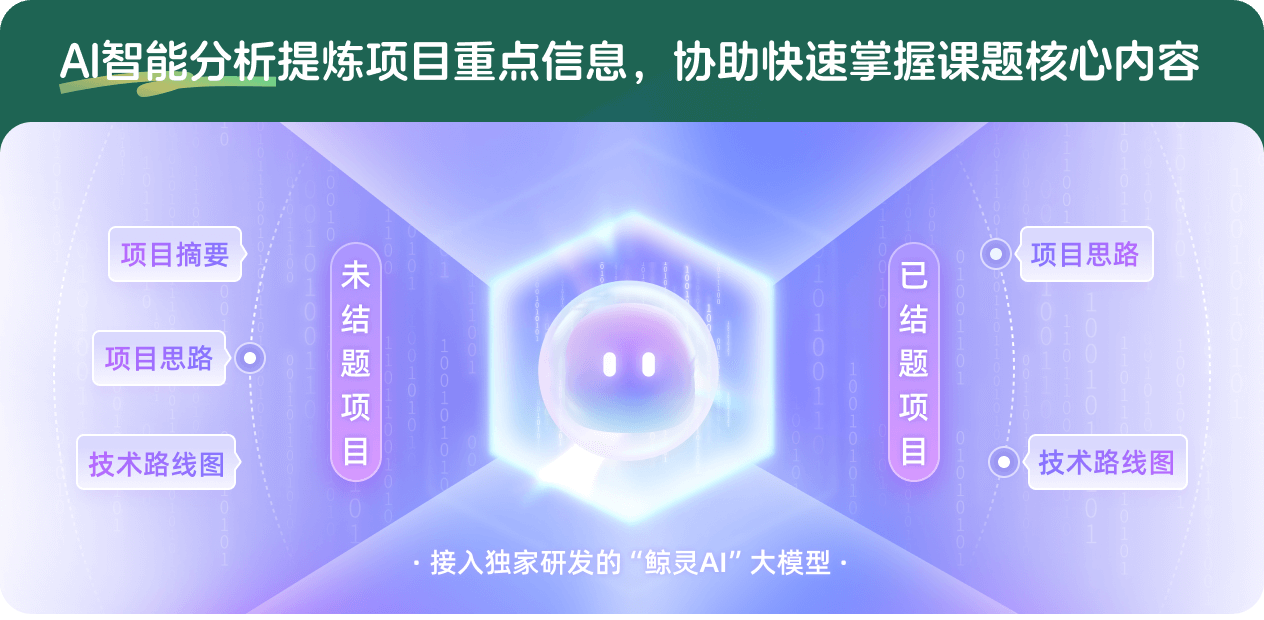
查看分析示例
此项目为已结题,我已根据课题信息分析并撰写以下内容,帮您拓宽课题思路:
AI项目摘要
AI项目思路
AI技术路线图
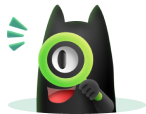
请为本次AI项目解读的内容对您的实用性打分
非常不实用
非常实用
1
2
3
4
5
6
7
8
9
10
您认为此功能如何分析更能满足您的需求,请填写您的反馈:
相似国自然基金
{{ item.name }}
- 批准号:{{ item.ratify_no }}
- 批准年份:{{ item.approval_year }}
- 资助金额:{{ item.support_num }}
- 项目类别:{{ item.project_type }}
相似海外基金
{{
item.name }}
{{ item.translate_name }}
- 批准号:{{ item.ratify_no }}
- 财政年份:{{ item.approval_year }}
- 资助金额:{{ item.support_num }}
- 项目类别:{{ item.project_type }}