大范围不确定随机线性系统自适应滤波估计及其若干应用
项目介绍
AI项目解读
基本信息
- 批准号:61473038
- 项目类别:面上项目
- 资助金额:84.0万
- 负责人:
- 依托单位:
- 学科分类:F0301.控制理论与技术
- 结题年份:2018
- 批准年份:2014
- 项目状态:已结题
- 起止时间:2015-01-01 至2018-12-31
- 项目参与者:张艳霞; 费庆; 刘华; 金英; 冯波; 王新宇; 胡琼; 李江平; 李宁;
- 关键词:
项目摘要
Filtering is a basic and general technology, which is widely used in various areas such as signal de-noising applications and inertial navigation systems, and Kalman filter algorithm is a standard filtering method for linear systems. At present one subtle difficulty for filtering technology is how to design a suitable filtering algorithm which can effectively utilize the measurement signal and guarantee the filtering stability and real-time performance at the presence of large parametric or structural uncertainties. For a stochastic linear system state space model, successful application of standard Kalman filter requires that the system parameters and the noise statistical nature must be obtained a priori precisely. However, such requirements are seldom completely satisfied in practice due to the commonly existence of modeling errors, measurement errors, quantitative errors or unmodeled dynamics. The unknown noise covariance matrix could be estimated in real-time by the adaptive Kalman filter (AKF) or self-tuning Kalman filtering algorithm, however for these algorithms, the parameter estimation is coupled with the state estimating process, which makes the close-loop stability analysis of the filtering algorithm very difficult and the mathematical stability analysis has not yet been fully resolved up to now. Considering such background, motivated by our previous research on the adaptive control and finite-model filtering algorithms, a series of new filtering algorithms will be proposed and investigated so as to deal with the problem of state estimation when there exist large uncertainties of system parameters or system structure. And new mathematical analysis techniques will be used to present the stability analysis results for the new algorithms and the new algorithms will be verified in the inertial navigation systems and mechanical manipulator systems.
滤波估计是一种通用性技术,广泛应用于信号降噪、惯性导航等诸多领域,其典型代表为卡尔曼滤波。滤波估计技术的一个核心困难是系统具有大范围参数不确定性或结构不确定性或先验信息缺乏时如何设计滤波器有效利用可获得的数据保证算法具有稳定性,兼顾精度与实时性。针对随机线性系统,标准卡尔曼滤波算法要求系统模型和噪声统计特性精确已知,但由于建模、测量及量化过程中普遍存在的误差或未建模动态,这一假设在工程实践中常难以满足。已有的自适应卡尔曼滤波、自校正滤波算法可在线估计未知的噪声方差,但由于状态估计和参数辨识相互耦合,其稳定性分析十分困难,理论上尚未完全解决。本项目拟针对这一背景,基于前期对自适应控制以及有限模型滤波算法等方面的研究,对随机线性系统模型中存在大范围参数不确定性或结构不确定性时的滤波估计问题,提出新的滤波估计算法,引入新的数学工具进行理论分析,并将算法在惯导系统、多机械臂系统中加以验证与应用。
结项摘要
本基金项目围绕着大范围不确定随机线性系统自适应滤波估计,在滤波理论、适应学习、应用实例取得了多方面的研究成果。..在滤波理论方面,主要取得以下成果:1)针对随机线性系统过程噪声或观测噪声协方差矩阵完全未知的情形下,对标准卡尔曼滤波算法进行改进,通过从观测序列中构造新随机变量的新思想,实现过程噪声或观测噪声协方差矩阵的在线辨识。2)针对模型与真实系统不匹配时的滤波估计问题,本项目通过有限模型的切换或组合来逼近真实系统的动态,设计了模型间的自适应切换律或融合规则,实现在真实系统未知时对未知状态的估计,并进一步设计了滤波估计与控制设计融为一体的新型自适应控制器。3)针对采样过程中存在的观测及量化误差,通过引入自适应控制思想及两阶段卡尔曼滤波技术,实现了对未知状态和参数的自适应估计,从而对采样后系统的大范围参数不确定性以及噪声的不确定性进行了有效的补偿。4)针对具有不确定耦合作用的多智能体系统,应用单个系统中自适应滤波估计与控制设计的算法及思想,解决了多智能体系统中每个智能体的未知状态与未知耦合参数的在线估计,并基于分散滤波估计算法,在这些不确定性存在的条件下,进一步研究了多智能体系统的分散自适应控制。..在适应学习方面,主要取得以下成果:1)针对具有内部不确定性及耦合不确定性的多智能体系统,进行了一些自适应估计与控制算法的研究,而多智能体系统由于新的耦合不确定性的引入,设计估计与控制算法时既借鉴单智能体情形的基本算法思想,又要针对耦合进行估计补偿。2)提出了具备实时轻量学习能力的新一代人工智能技术,并将其用于同时具有参数不确定性和非参数不确定性的系统,对其中的非线性函数进行估计和补偿,在此基础上基于项目负责人提出的信息浓缩原理,设计出半参数自适应控制器,仿真结果表明其有效性。..在应用实例方面,项目组针对机器人、无人机、无人车等运动体,进行了多传感器信息融合、即时定位与地图构建、协同定位、车机协同、目标检测识别跟踪等研究,取得了一系列针对应用背景的成果。..以上成果多数发表于国际知名学术刊物与会议,项目所发表论文被SCI/EI检索20余篇。项目负责人将在国际顶级学术出版社Springer出版专著《Kalman Filtering and Information Fusion》。
项目成果
期刊论文数量(11)
专著数量(0)
科研奖励数量(5)
会议论文数量(10)
专利数量(8)
Switching control system based on robust model reference adaptive control
基于鲁棒模型参考自适应控制的切换控制系统
- DOI:10.1007/s11424-016-4223-4
- 发表时间:2016-05
- 期刊:Journal of Systems Science and Complexity
- 影响因子:2.1
- 作者:Hu Qiong;Fei Qing;Ma Hongbin;Wu Qinghe;Geng Qingbo;Hu Qiong;Fei Qing;Ma Hongbin;Wu Qinghe;Fei Q;Fei Q
- 通讯作者:Fei Q
Robot manipulator self-identification for surrounding obstacle detection
机器人机械臂自我识别周围障碍物检测
- DOI:10.1007/s11042-016-3275-8
- 发表时间:2017-03-01
- 期刊:MULTIMEDIA TOOLS AND APPLICATIONS
- 影响因子:3.6
- 作者:Wang, Xinyu;Yang, Chenguang;Fu, Mengyin
- 通讯作者:Fu, Mengyin
Decentralized adaptive synchronization with bounded identification errors for discrete-time nonlinear multi-agent systems with unknown parameters and unknown high-frequency gains
具有未知参数和未知高频增益的离散时间非线性多智能体系统的具有有限识别误差的分散自适应同步
- DOI:10.1016/j.jfranklin.2017.11.017
- 发表时间:2018
- 期刊:Journal of the Franklin Institute
- 影响因子:--
- 作者:Zhang Xinghong;Ma Hongbin;Ma Hongbin;Ma HB;Ma HB
- 通讯作者:Ma HB
Novel hybrid adaptive controller for manipulation in complex perturbation environments.
用于复杂扰动环境中操纵的新型混合自适应控制器
- DOI:10.1371/journal.pone.0129281
- 发表时间:2015
- 期刊:PloS one
- 影响因子:3.7
- 作者:Smith AM;Yang C;Ma H;Culverhouse P;Cangelosi A;Burdet E
- 通讯作者:Burdet E
An adaptive Kalman filter estimating process noise covariance
估计过程噪声协方差的自适应卡尔曼滤波器
- DOI:10.1016/j.neucom.2016.10.026
- 发表时间:2017-02-05
- 期刊:NEUROCOMPUTING
- 影响因子:6
- 作者:Wang, Hairong;Deng, Zhihong;Xia, Yuanqing
- 通讯作者:Xia, Yuanqing
数据更新时间:{{ journalArticles.updateTime }}
{{
item.title }}
{{ item.translation_title }}
- DOI:{{ item.doi || "--"}}
- 发表时间:{{ item.publish_year || "--" }}
- 期刊:{{ item.journal_name }}
- 影响因子:{{ item.factor || "--"}}
- 作者:{{ item.authors }}
- 通讯作者:{{ item.author }}
数据更新时间:{{ journalArticles.updateTime }}
{{ item.title }}
- 作者:{{ item.authors }}
数据更新时间:{{ monograph.updateTime }}
{{ item.title }}
- 作者:{{ item.authors }}
数据更新时间:{{ sciAawards.updateTime }}
{{ item.title }}
- 作者:{{ item.authors }}
数据更新时间:{{ conferencePapers.updateTime }}
{{ item.title }}
- 作者:{{ item.authors }}
数据更新时间:{{ patent.updateTime }}
其他文献
基于函数逼近技术的双机械臂自适应阻抗力控制
- DOI:--
- 发表时间:2014
- 期刊:系统科学与数学
- 影响因子:--
- 作者:许泽军;马宏宾;杨辰光;李江平
- 通讯作者:李江平
其他文献
{{
item.title }}
{{ item.translation_title }}
- DOI:{{ item.doi || "--" }}
- 发表时间:{{ item.publish_year || "--"}}
- 期刊:{{ item.journal_name }}
- 影响因子:{{ item.factor || "--" }}
- 作者:{{ item.authors }}
- 通讯作者:{{ item.author }}
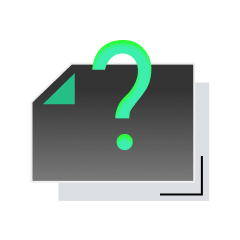
内容获取失败,请点击重试
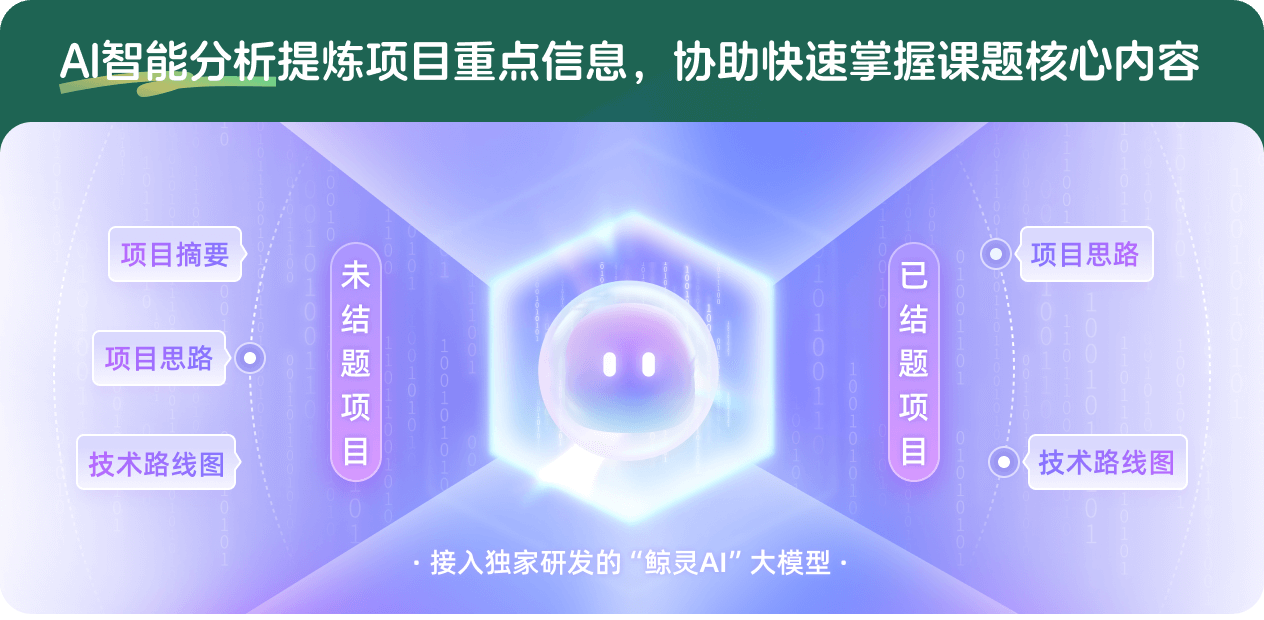
查看分析示例
此项目为已结题,我已根据课题信息分析并撰写以下内容,帮您拓宽课题思路:
AI项目摘要
AI项目思路
AI技术路线图
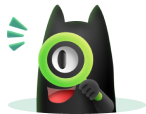
请为本次AI项目解读的内容对您的实用性打分
非常不实用
非常实用
1
2
3
4
5
6
7
8
9
10
您认为此功能如何分析更能满足您的需求,请填写您的反馈:
马宏宾的其他基金
面向智能博弈的非对称动态对策建模仿真与自适应学习
- 批准号:62076028
- 批准年份:2020
- 资助金额:59 万元
- 项目类别:面上项目
基于数据驱动适应学习的人-机-环境多模态感知与自然交互
- 批准号:91648117
- 批准年份:2016
- 资助金额:67.0 万元
- 项目类别:重大研究计划
多智能体分散自适应控制中的反馈学习机制及其极限
- 批准号:61004059
- 批准年份:2010
- 资助金额:20.0 万元
- 项目类别:青年科学基金项目
相似国自然基金
{{ item.name }}
- 批准号:{{ item.ratify_no }}
- 批准年份:{{ item.approval_year }}
- 资助金额:{{ item.support_num }}
- 项目类别:{{ item.project_type }}
相似海外基金
{{
item.name }}
{{ item.translate_name }}
- 批准号:{{ item.ratify_no }}
- 财政年份:{{ item.approval_year }}
- 资助金额:{{ item.support_num }}
- 项目类别:{{ item.project_type }}