基于时序图像分析的冬小麦苗期冠层生长动态监测方法研究
项目介绍
AI项目解读
基本信息
- 批准号:31801264
- 项目类别:青年科学基金项目
- 资助金额:23.0万
- 负责人:
- 依托单位:
- 学科分类:C1301.农业信息学
- 结题年份:2021
- 批准年份:2018
- 项目状态:已结题
- 起止时间:2019-01-01 至2021-12-31
- 项目参与者:杜克明; 郑飞翔; 孙忠富; 王翔宇; 李云霞; 陈运强;
- 关键词:
项目摘要
The continuous monitoring of winter wheat growth at seedling stage is of great significance to acquire the growth condition and to improve the final yield. A continuous monitoring method for canopy dynamics can reveal the time sequence regulation and fitting modal response, which will instruct the establishment of an accurate winter wheat growth monitoring method and improve the management at seedling stage. Recent studies showed that the growth monitoring method at small and medium scale were subjected to the facts that the data were not continuous and the cost was high. The capacity of continually capturing the canopy dynamics of winter wheat at seedling stage is limited, which leads to the ambiguity of the time sequence regulation and fitting modal response. Based on the Internet of Things-based field monitoring, time-series images can be captured continuously and automatically in this research, which lays a solid foundation for dissecting the digital traits of winter wheat canopy images and constructing the monitoring method for canopy growth dynamics. An interaction mechanism of continuous monitoring- growth dynamics- fitting modal response is proposed to reveal the time sequence regulation and fitting modal response, which can support the in-field growth monitor and field management of winter wheat at seedling stage.
冬小麦苗期长势连续监测对于实时掌握冬小麦苗情,及时准确制定生产管理措施和提高产量具有重要实际意义。建立冬小麦冠层生长动态连续监测方法,揭示冠层生长动态的变化规律及其模型响应,有助于建立准确的冬小麦苗期长势连续监测方法,提高冬小麦苗期田间精细管理水平。目前中小尺度的长势监测方法受限于数据采集连续性、使用成本等问题,在田间尺度对冬小麦苗期冠层生长动态的连续捕捉能力有限,难以准确掌握冠层生长动态变化规律及其模型响应,造成监测方法针对性不强。本研究将可见光图像处理与大田物联网监测结合,连续、动态采集冬小麦苗期图像数据;以时序可见光图像数据为基础,研究冬小麦苗期冠层图像分割方法,解析冬小麦苗期冠层图像数字特征,建立冬小麦苗期冠层生长动态连续监测方法,通过连续监测-生长动态-模型响应研究机制,揭示冬小麦苗期冠层生长动态规律与模型响应,为田间尺度冬小麦苗期长势连续监测和生产管理提供决策支撑。
结项摘要
叶面积指数(leaf area index,LAI)和地上生物量(above ground biomass,AGB)是表征冬小麦长势的2个重要参数。农田尺度的LAI和AGB估算对于冬小麦苗期长势监测与田间精细管理具有重要的意义。针对目前基于计算机视觉估算冬小麦苗期长势参数存在易受噪声干扰且对人工特征依赖性较强的问题,本研究综合运用图像处理和深度学习技术,提出一种基于卷积神经网络(Convolutional Neural Network,CNN)的冬小麦苗期长势参数估算方法。以冬小麦苗期冠层可见光图像作为输入,构建适用于冬小麦苗期长势参数估算CNN模型,通过学习的方式建立冬小麦冠层可见光图像与长势参数的关系,实现农田尺度冬小麦苗期冠层LAI和AGB的准确估算。.研究结果表明:提出的冬小麦苗期长势监测方法估算的长势参数和实测长势参数之间存在良好的线性关系,其决定系数均在0.8以上;并且该方法具有优秀的泛化能力;网络结构及超参数对网络准确率影响程度不同,颜色信息缺失会造成准确率下降,输入图像尺寸增大会在一定程度上提升准确率等。如果采用随机梯度法进行模型训练,批处理和学习率对网络的准确率具有一定的影响,呈现为二次曲线的形状;方法准确率同样受到外部因素的影响,植株密度提升会影响估算准确率,虽然准确率出现下降,但依然保持了良好的稳定性;图像饱和降低方法在冬小麦生育期中后期的准确率,在冬小麦拔节和孕穗期的测试结果中发现,受到图像饱和的影响,估算的参数无法反映冬小麦的实际长势情况,该方法仅适用于冬小麦苗期。.该方法通过对时序图像的获取和分析,能够对叶面积指数和地上生物量进行连续监测,进而反映出冬小麦苗期长势的年际变化,是物联网技术和人工智能前沿技术推动智慧农业发展的有效探索。此外,由于可见光图像具有低成本、准确率高的特点,该方法能够有效降低成本,提升适用性。
项目成果
期刊论文数量(8)
专著数量(0)
科研奖励数量(0)
会议论文数量(0)
专利数量(0)
Towards improved accuracy of UAV-based wheat ears counting: A transfer learning method of the ground-based fully convolutional network
提高无人机麦穗计数的准确性:基于地面的全卷积网络的迁移学习方法
- DOI:10.1016/j.eswa.2021.116226
- 发表时间:2021-12-08
- 期刊:EXPERT SYSTEMS WITH APPLICATIONS
- 影响因子:8.5
- 作者:Ma, Juncheng;Li, Yunxia;Zhang, Lingxian
- 通讯作者:Zhang, Lingxian
Improving segmentation accuracy for ears of winter wheat at flowering stage by semantic segmentation
利用语义分割提高花期冬小麦穗的分割精度
- DOI:10.1016/j.compag.2020.105662
- 发表时间:2020-09-01
- 期刊:COMPUTERS AND ELECTRONICS IN AGRICULTURE
- 影响因子:8.3
- 作者:Ma, Juncheng;Li, Yunxia;Zhang, Lingxian
- 通讯作者:Zhang, Lingxian
Estimation of leaf area index for winter wheat at early stages based on convolutional neural networks
基于卷积神经网络的冬小麦早期叶面积指数估算
- DOI:10.1016/j.compag.2021.106480
- 发表时间:2021-09-29
- 期刊:COMPUTERS AND ELECTRONICS IN AGRICULTURE
- 影响因子:8.3
- 作者:Li, Yunxia;Liu, Hongjie;Zhang, Lingxian
- 通讯作者:Zhang, Lingxian
Segmenting ears of winter wheat at flowering stage using digital images and deep learning
利用数字图像和深度学习在开花期分割冬小麦的穗
- DOI:10.1016/j.compag.2019.105159
- 发表时间:2020-01-01
- 期刊:COMPUTERS AND ELECTRONICS IN AGRICULTURE
- 影响因子:8.3
- 作者:Ma, Juncheng;Li, Yunxia;Jiao, Weihua
- 通讯作者:Jiao, Weihua
基于卷积神经网络的冬小麦麦穗检测计数系统
- DOI:--
- 发表时间:2019
- 期刊:农业机械学报
- 影响因子:--
- 作者:张领先;陈运强;李云霞;马浚诚;杜克明
- 通讯作者:杜克明
数据更新时间:{{ journalArticles.updateTime }}
{{
item.title }}
{{ item.translation_title }}
- DOI:{{ item.doi || "--"}}
- 发表时间:{{ item.publish_year || "--" }}
- 期刊:{{ item.journal_name }}
- 影响因子:{{ item.factor || "--"}}
- 作者:{{ item.authors }}
- 通讯作者:{{ item.author }}
数据更新时间:{{ journalArticles.updateTime }}
{{ item.title }}
- 作者:{{ item.authors }}
数据更新时间:{{ monograph.updateTime }}
{{ item.title }}
- 作者:{{ item.authors }}
数据更新时间:{{ sciAawards.updateTime }}
{{ item.title }}
- 作者:{{ item.authors }}
数据更新时间:{{ conferencePapers.updateTime }}
{{ item.title }}
- 作者:{{ item.authors }}
数据更新时间:{{ patent.updateTime }}
其他文献
Sentinel-2宽波段光谱指数预测拔节后受冻冬小麦减产率
- DOI:--
- 发表时间:--
- 期刊:光谱学与光谱分析
- 影响因子:--
- 作者:赵爱萍;马浚诚;武永峰;胡新;任德超;李崇瑞
- 通讯作者:李崇瑞
其他文献
{{
item.title }}
{{ item.translation_title }}
- DOI:{{ item.doi || "--" }}
- 发表时间:{{ item.publish_year || "--"}}
- 期刊:{{ item.journal_name }}
- 影响因子:{{ item.factor || "--" }}
- 作者:{{ item.authors }}
- 通讯作者:{{ item.author }}
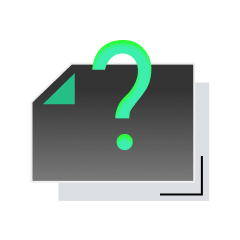
内容获取失败,请点击重试
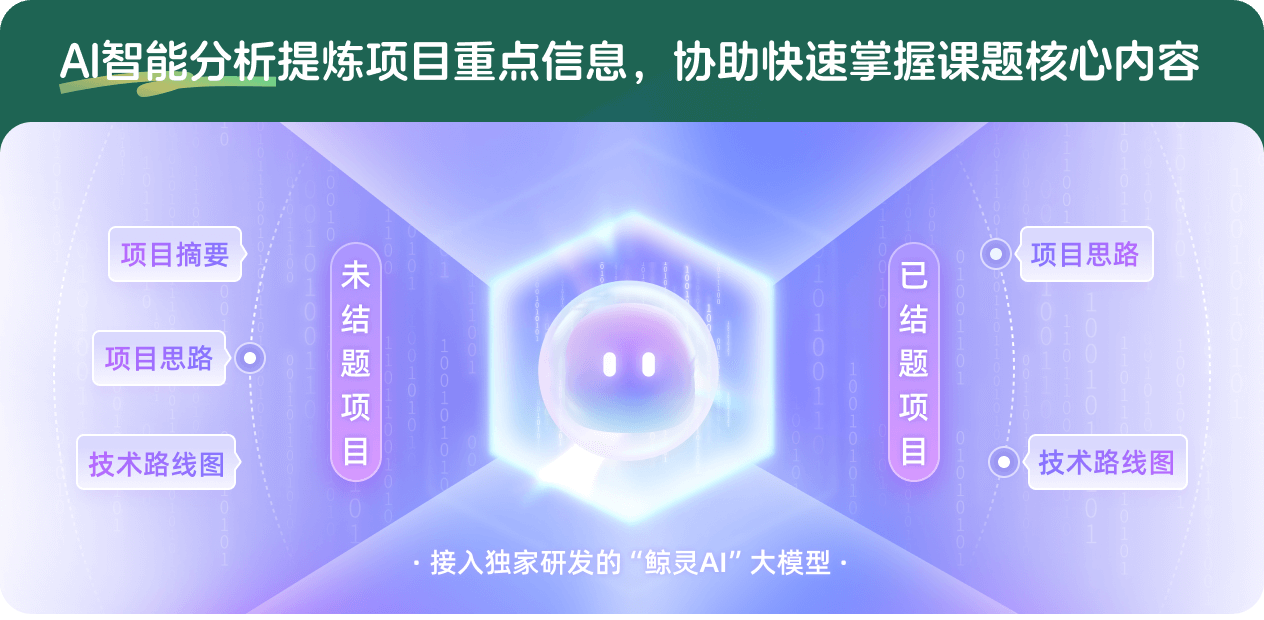
查看分析示例
此项目为已结题,我已根据课题信息分析并撰写以下内容,帮您拓宽课题思路:
AI项目摘要
AI项目思路
AI技术路线图
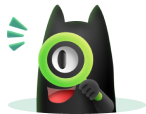
请为本次AI项目解读的内容对您的实用性打分
非常不实用
非常实用
1
2
3
4
5
6
7
8
9
10
您认为此功能如何分析更能满足您的需求,请填写您的反馈:
马浚诚的其他基金
农田尺度具有场景自适应能力的冬小麦产量预测方法研究
- 批准号:32371998
- 批准年份:2023
- 资助金额:50.00 万元
- 项目类别:面上项目
相似国自然基金
{{ item.name }}
- 批准号:{{ item.ratify_no }}
- 批准年份:{{ item.approval_year }}
- 资助金额:{{ item.support_num }}
- 项目类别:{{ item.project_type }}
相似海外基金
{{
item.name }}
{{ item.translate_name }}
- 批准号:{{ item.ratify_no }}
- 财政年份:{{ item.approval_year }}
- 资助金额:{{ item.support_num }}
- 项目类别:{{ item.project_type }}