基于深度卷积神经网络和超像素CRF模型的高空间分辨率遥感图像多尺度征提取与分类识别研究
项目介绍
AI项目解读
基本信息
- 批准号:41601365
- 项目类别:青年科学基金项目
- 资助金额:19.0万
- 负责人:
- 依托单位:
- 学科分类:D0113.遥感科学
- 结题年份:2019
- 批准年份:2016
- 项目状态:已结题
- 起止时间:2017-01-01 至2019-12-31
- 项目参与者:孙岩标; 黄景金; 张荣庭; 黄帅;
- 关键词:
项目摘要
It is popular and important to apply spectral, spatial features and contextual information synthetically for high spatial resolution remotely sensed image classification. However, there are some drawbacks for the methods developed in this research area. For the feature extraction methods, they are separated from classification procedure and need professional prior knowledge to design. For the contextual information application, it is complicated in computing and difficult to capture long distance spatial context. According to these problems, in this study, we investigated to develop a framework based on convolutional neural networks and superpixel Conditional Random Fields (CRF) model to overcome those drawbacks. This framework includes three parts: hierarchical feature extraction based on deep convolutional neural networks. This model is based on the deep learning theory, fuses feature extraction and classification into one procedure without prior knowledge and can extract low level features, such as edge and corner, and high level features, like texture and shape, at the same time. The second part is superpixel based CRF model construction. This model can capture the long spatial context on superpixel scale. Except that, this model improves the computing efficiency of CRF significantly which makes it suitable for high spatial resolution remotely sensed image with huge data amount. The last part is superpixel based CRF model inference by applying Graph cuts algorithm. This project will provide a new way and framework for spectral-spatial based classification and improve the level of processing and the application for high spatial resolution remotely sensed images.
综合应用光谱、纹理、形状特征和空间上下文信息是目前高空间分辨率遥感图像分类识别研究的热点。针对目前研究中特征提取与分类识别过程相分离且依赖于领域先验知识来设计提取模型,以及难以捕获长距离空间上下文信息等问题,本研究提出了一种基于深度卷积神经网络模型和超像素条件随机场模型(CRF)的特征提取和分类识别框架,并进行实验验证。研究内容包括:基于深度卷积神经网络的多尺度特征提取研究。该模型依据新型的深度学习理论,融合特征提取和分类识别于统一过程,无需领域先验知识,并能够完成从低级特征到高级特征的一体化多尺度提取;基于超像素的CRF模型构建研究。该模型能够捕获长距离的空间上下文信息,并大大减少CRF模型的计算复杂度;基于Graph cuts算法的CRF模型最优化推断(inference)。本研究将为融合光谱、空间特征和空间上下文信息高空间分辨率遥感图像分析提供一种新的思路,并提高其处理和应用水平。
结项摘要
综合应用光谱、纹理、形状特征和空间上下文信息是目前高空间分辨率遥感图像分类识别研究的热点。针对目前研究中特征提取与分类识别过程相分离且依赖于领域先验知识来设计提取模型,以及难以捕获长距离空间上下文信息等问题,本研究提出了一种基于深度卷积神经网络模型和超像素条件随机场模型(CRF)的特征提取和分类识别框架,并进行实验验证。研究内容包括:基于深度卷积神经网络的多尺度特征提取研究。该模型依据新型的深度学习理论,融合特征提取和分类识别于统一过程,无需领域先验知识,并能够完成从低级特征到高级特征的一体化多尺度提取。融合多层卷积神经网络空间信息和光谱信息的遥感图像分类研究,光谱信息作为一个独立的特征输入显著的改善了分类的效果。开展了基于FPGA的遥感图像实时化预处理和空间特征提取的相关工作,基于FPGA的编程模式是遥感图像分析的全新方式,该研究为后续的遥感图像软硬件相结合分类识别研究打下坚实基础。本研究将为融合光谱、空间特征和空间上下文信息高空间分辨率遥感图像分析提供一种新的思路,并提高其实时化处理和应用水平。
项目成果
期刊论文数量(6)
专著数量(0)
科研奖励数量(0)
会议论文数量(0)
专利数量(0)
基于FPGA的星上影像正射纠正
- DOI:--
- 发表时间:2019
- 期刊:航天返回与遥感
- 影响因子:--
- 作者:张荣庭;周国清;周祥;刘德全;黄景金
- 通讯作者:黄景金
On-Board Georeferencing Using FPGA-Based Optimized Second-Order Polynomial Equation
使用基于 FPGA 的优化二阶多项式方程进行板载地理配准
- DOI:10.3390/rs11020124
- 发表时间:2019
- 期刊:Remote Sensing
- 影响因子:5
- 作者:Liu Dequan;Zhou Guoqing;Huang Jingjin;Zhang Rongting;Shu Lei;Zhou Xiang;Xin Chun Sheng
- 通讯作者:Xin Chun Sheng
A New FPGA Architecture of FAST and BRIEF Algorithm for On-Board Corner Detection and Matching.
一种用于板载角点检测和匹配的快速简洁算法的新型 FPGA 架构
- DOI:10.3390/s18041014
- 发表时间:2018-03-28
- 期刊:Sensors (Basel, Switzerland)
- 影响因子:--
- 作者:Huang J;Zhou G;Zhou X;Zhang R
- 通讯作者:Zhang R
Hierarchical spatial features learning with deep CNNs for very high-resolution remote sensing image classification
使用深度 CNN 进行分层空间特征学习,实现极高分辨率遥感图像分类
- DOI:10.1080/01431161.2018.1506593
- 发表时间:2018
- 期刊:International Journal of Remote Sensing
- 影响因子:3.4
- 作者:Zhang Guangyun;Zhang Rongting;Zhou Guoqing;Jia Xiuping
- 通讯作者:Jia Xiuping
RPC-Based Orthorectification for Satellite Images Using FPGA.
使用 FPGA 对卫星图像进行基于 RPC 的正射校正
- DOI:10.3390/s18082511
- 发表时间:2018-08-01
- 期刊:Sensors (Basel, Switzerland)
- 影响因子:--
- 作者:Zhang R;Zhou G;Zhang G;Zhou X;Huang J
- 通讯作者:Huang J
数据更新时间:{{ journalArticles.updateTime }}
{{
item.title }}
{{ item.translation_title }}
- DOI:{{ item.doi || "--"}}
- 发表时间:{{ item.publish_year || "--" }}
- 期刊:{{ item.journal_name }}
- 影响因子:{{ item.factor || "--"}}
- 作者:{{ item.authors }}
- 通讯作者:{{ item.author }}
数据更新时间:{{ journalArticles.updateTime }}
{{ item.title }}
- 作者:{{ item.authors }}
数据更新时间:{{ monograph.updateTime }}
{{ item.title }}
- 作者:{{ item.authors }}
数据更新时间:{{ sciAawards.updateTime }}
{{ item.title }}
- 作者:{{ item.authors }}
数据更新时间:{{ conferencePapers.updateTime }}
{{ item.title }}
- 作者:{{ item.authors }}
数据更新时间:{{ patent.updateTime }}
其他文献
华东地区气候舒适度精细化估算及其分布特征研究
- DOI:--
- 发表时间:2015
- 期刊:云南大学学报(自然科学版)
- 影响因子:--
- 作者:张狄;史岚;丁浒;张广运;王锋
- 通讯作者:王锋
其他文献
{{
item.title }}
{{ item.translation_title }}
- DOI:{{ item.doi || "--" }}
- 发表时间:{{ item.publish_year || "--"}}
- 期刊:{{ item.journal_name }}
- 影响因子:{{ item.factor || "--" }}
- 作者:{{ item.authors }}
- 通讯作者:{{ item.author }}
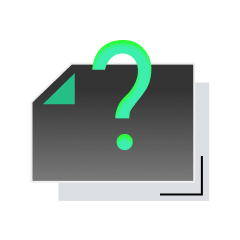
内容获取失败,请点击重试
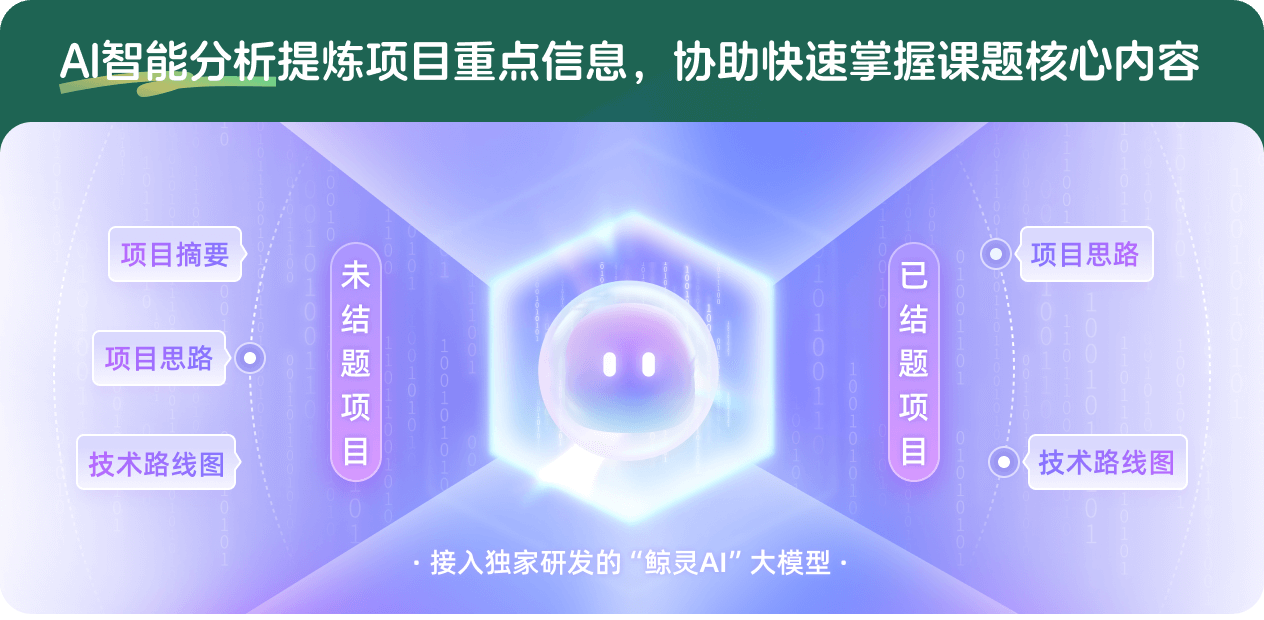
查看分析示例
此项目为已结题,我已根据课题信息分析并撰写以下内容,帮您拓宽课题思路:
AI项目摘要
AI项目思路
AI技术路线图
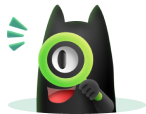
请为本次AI项目解读的内容对您的实用性打分
非常不实用
非常实用
1
2
3
4
5
6
7
8
9
10
您认为此功能如何分析更能满足您的需求,请填写您的反馈:
相似国自然基金
{{ item.name }}
- 批准号:{{ item.ratify_no }}
- 批准年份:{{ item.approval_year }}
- 资助金额:{{ item.support_num }}
- 项目类别:{{ item.project_type }}
相似海外基金
{{
item.name }}
{{ item.translate_name }}
- 批准号:{{ item.ratify_no }}
- 财政年份:{{ item.approval_year }}
- 资助金额:{{ item.support_num }}
- 项目类别:{{ item.project_type }}