基于整句层面的中文语义角色标注关键技术研究
项目介绍
AI项目解读
基本信息
- 批准号:61702209
- 项目类别:青年科学基金项目
- 资助金额:25.0万
- 负责人:
- 依托单位:
- 学科分类:F0211.信息检索与社会计算
- 结题年份:2020
- 批准年份:2017
- 项目状态:已结题
- 起止时间:2018-01-01 至2020-12-31
- 项目参与者:王炜; 周寅; 袁策书; 杜秋实;
- 关键词:
项目摘要
All predicates of a sentence convey the whole semantics of the sentence together. But, the current approaches to Semantic Role Labeling (SRL) usually perform role classifications for each predicate separately but ignore the semantic interaction among all the predicates, which cause much useful structure and semantic information on sentence level missing and finally make the performance of SRL system dramatically decline. Therefore, in order to solve the problem, we propose a series of corresponding methods and the main contents of this proposal include: 1) study on a novel framework on sentence-level to do SRL, taking into account the semantic interaction among multiple predicates in a sentence; 2) study on a global inference method under the above framework, ensuring the global optimal result with consistent outputs; 3) study on the sparse predicates and domain migration problem with the help of deep learning to improve the robustness and generalization. The expected research results will enrich current SRL theory from predicate-level to sentence-level and promote the development of related technologies, which has important theoretical significance and practical value.
一个句子中的全部谓词联合起来表达了句子的完整语义。然而,目前主流的语义角色标注方法仍然停留在单个“谓词”层面,忽视了句子中谓词与谓词之间的语义关联,这就导致很多只能在句子层面获取到的结构和语义信息严重缺失,从而使得语义角色标注的准确率急剧下降。因此,本项目紧紧围绕整句层面的语义角色标注展开如下创新性研究:1)研究基于“整句”层面的语义角色标注框架,兼顾考虑句中多个谓词语义角色标注之间的语义关联;2)研究统一框架下的全局推断模型,力保联合标注模型能够输出全局最优、高度一致性的语义标注结果;3)借助深度学习强大的表征和抽象能力,研究稀疏谓词的标注方法和领域迁移方法,提升标注模型的鲁棒性和泛化性。本项目的预期研究成果将使现有的语义角色标注理论从“谓词”层面提升到“整句”层面,该成果将丰富和发展现有的语义角色标注理论方法,并能推动相关技术的发展,具有重要的理论研究意义和实用价值。
结项摘要
目前主流的语义角色标注方法仍然停留在单个“谓词”层面,而忽视了句子中谓词与谓词之间的语义关联。本项目围绕句子层面的语义角色标注进行了深入的研究和探索:(1)提出一种基于图模型的中文多谓词语义角色标注方法,可以利用句中多个谓词之间的全局特征,提升语义角色的区分度,并采用随机爬山算法优化图模型;(2)对于中文中的稀疏谓词,提出基于层次化聚类的稀疏谓词语义角色标注方法,稀疏谓词可以泛化为与之相近的常用谓词,大大缓和了语义角色标注中的稀疏谓词问题;(3)提出了一种基于深度学习的整句语义角色标注方法,通过局部模型生成初始的结果候选,然后通过深度模型框架挖掘整句的语义特征,进而提升语义角色标注的效果,同时语义分析的效率较高;(4)针对语义角色标注中的领域适应问题,提出了基于深度对抗学习的领域适应方法,可以从多个领域的无标注数据中挖掘出学习到统一的特征表示,并将此特征表示输入到深度学习框架中用于分类,可以显著地改善语义角色标注中的领域适应问题。本项目的研究表明整句层次包含着大量有益的信息,合理利用这些信息构建整句级别的语义角色标注方法可以显著地提升语义角色标注的效果。
项目成果
期刊论文数量(4)
专著数量(0)
科研奖励数量(0)
会议论文数量(0)
专利数量(0)
基于图模型的中文多谓词语义角色标注方法
- DOI:10.19678/j.issn.1000-3428.0048758
- 发表时间:2019
- 期刊:计算机工程
- 影响因子:--
- 作者:杨海彤
- 通讯作者:杨海彤
A Global Deep Reranking Model for Semantic Role Classification
用于语义角色分类的全局深度重排序模型
- DOI:10.1587/transinf.2020edl8164
- 发表时间:2021-07
- 期刊:IEICE Transaction on Information and System
- 影响因子:--
- 作者:Yang Haitong
- 通讯作者:Yang Haitong
基于层次化聚类的稀疏谓词语义角色标注方法
- DOI:10.16208/j.issn1000-7024.2018.11.016
- 发表时间:2018
- 期刊:计算机工程与设计
- 影响因子:--
- 作者:杨海彤
- 通讯作者:杨海彤
数据更新时间:{{ journalArticles.updateTime }}
{{
item.title }}
{{ item.translation_title }}
- DOI:{{ item.doi || "--"}}
- 发表时间:{{ item.publish_year || "--" }}
- 期刊:{{ item.journal_name }}
- 影响因子:{{ item.factor || "--"}}
- 作者:{{ item.authors }}
- 通讯作者:{{ item.author }}
数据更新时间:{{ journalArticles.updateTime }}
{{ item.title }}
- 作者:{{ item.authors }}
数据更新时间:{{ monograph.updateTime }}
{{ item.title }}
- 作者:{{ item.authors }}
数据更新时间:{{ sciAawards.updateTime }}
{{ item.title }}
- 作者:{{ item.authors }}
数据更新时间:{{ conferencePapers.updateTime }}
{{ item.title }}
- 作者:{{ item.authors }}
数据更新时间:{{ patent.updateTime }}
其他文献
其他文献
{{
item.title }}
{{ item.translation_title }}
- DOI:{{ item.doi || "--" }}
- 发表时间:{{ item.publish_year || "--"}}
- 期刊:{{ item.journal_name }}
- 影响因子:{{ item.factor || "--" }}
- 作者:{{ item.authors }}
- 通讯作者:{{ item.author }}
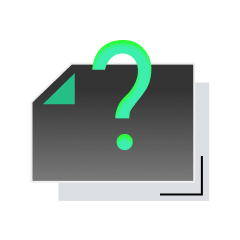
内容获取失败,请点击重试
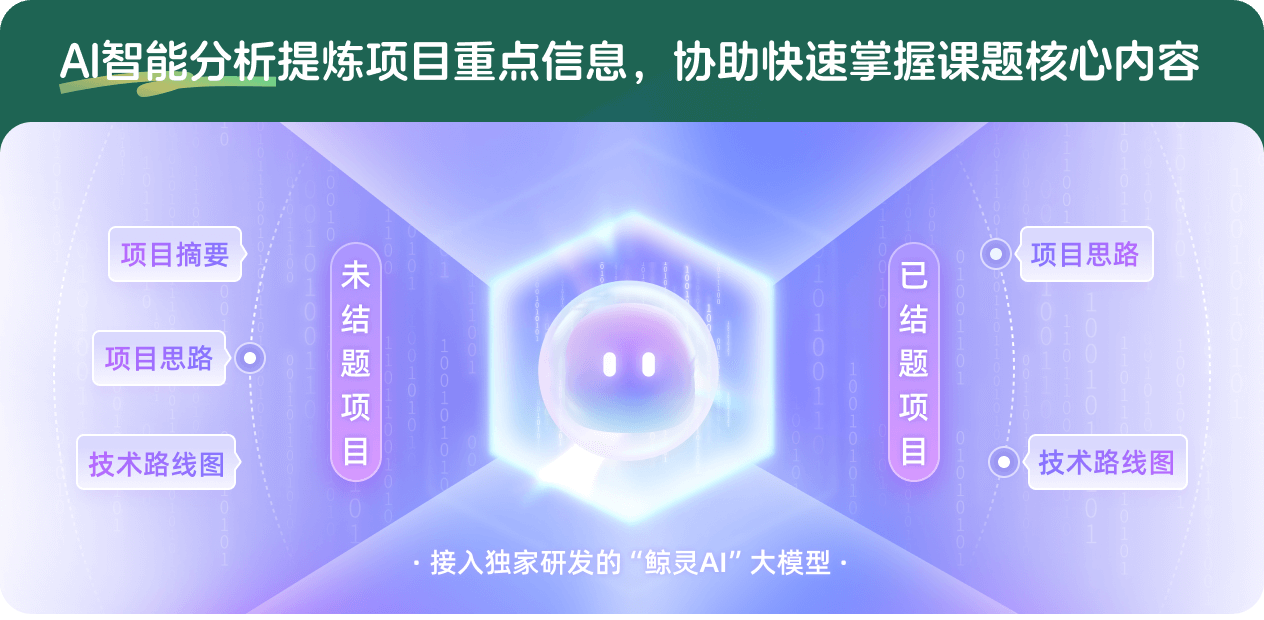
查看分析示例
此项目为已结题,我已根据课题信息分析并撰写以下内容,帮您拓宽课题思路:
AI项目摘要
AI项目思路
AI技术路线图
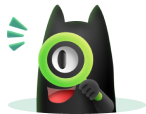
请为本次AI项目解读的内容对您的实用性打分
非常不实用
非常实用
1
2
3
4
5
6
7
8
9
10
您认为此功能如何分析更能满足您的需求,请填写您的反馈:
相似国自然基金
{{ item.name }}
- 批准号:{{ item.ratify_no }}
- 批准年份:{{ item.approval_year }}
- 资助金额:{{ item.support_num }}
- 项目类别:{{ item.project_type }}
相似海外基金
{{
item.name }}
{{ item.translate_name }}
- 批准号:{{ item.ratify_no }}
- 财政年份:{{ item.approval_year }}
- 资助金额:{{ item.support_num }}
- 项目类别:{{ item.project_type }}