面向通用GPU虚拟化多任务的三维堆叠存储架构研究
项目介绍
AI项目解读
基本信息
- 批准号:61402285
- 项目类别:青年科学基金项目
- 资助金额:27.0万
- 负责人:
- 依托单位:
- 学科分类:F0204.计算机系统结构与硬件技术
- 结题年份:2017
- 批准年份:2014
- 项目状态:已结题
- 起止时间:2015-01-01 至2017-12-31
- 项目参与者:毛志刚; 金威; 赵仲元; 王剑峰; 熊力孚;
- 关键词:
项目摘要
Recently, thanks to the lower cost and higher energy efficiency of GPUs (Graphics Processing Units), the General Purpose GPU (GPGPU) computing has provided new opportunities for high performance computing in the future. However, the limitations in both GPU architecture and runtime framework have degraded it as a mere accelerator, and thus cannot directly participate in general computing that essentially featured by multitasking. These limitations greatly prohibit the wide use of GPUs in high performance computing. In face of the absent hardware support on multitasking, we propose to extend the GPU architecture and optimize the runtime framework to virtualize the computing, memory and interconnect resources in GPGPU. To this goal, we focus on the GPU memory architecture and first adapt it to the state-of-art 3D stacked memory integration for larger storage capacity, higher communication bandwidth and lower latency, which are all inadequate by the 2D memory design in contemporary GPUs. Based on the new memory implementation, we then study the 3D stacked memory architecture and dynamic resource management to facilitate the GPGPU multitasking and virtualization. Furthermore, with the aid of runtime framework for multitasking, we can virtualize the memory resource in a more fine-grained and economic way, and thus better support the high performance computing and GPU virtualization in the future.
近年来,基于GPU处理器的通用GPU计算平台以其成本和能效优势为未来的高性能计算提供了新的方法和手段。但GPU本身架构和运行框架的限制使其只能以加速器的角色出现,而无法在以并行多任务处理为基本特征的通用计算环境中直接地发挥作用,从而制约了通用GPU计算的广泛应用。因此,本课题提出对现有GPU架构的拓展和运行框架的优化来实现计算、存储和互连资源的虚拟共享,进而支持GPU处理器片上虚拟化多任务并行处理的目标。针对这一目标,本课题以存储架构为切入点,利用最新的三维堆叠存储器集成工艺所提供的优势,应对现有GPU二维存储设计所面临的存储容量、通信带宽以及延时的诸多挑战。在此基础上,进一步探索和研究符合通用GPU计算虚拟化多任务需求的存储架构设计方法和资源动态管理机制,并借助运行框架多任务处理的拓展,实现更细粒度、更为经济的存储虚拟化方式,为未来的高性能计算及GPU虚拟化提供硬件架构和运行框架的支持。
结项摘要
近年来,GPU处理器以其卓越的性能和能效优势为高性能计算提供了新的方法和手段。随着通用CPU+GPU异构计算平台的不断发展和进步,GPU处理器需要在以并行化为基本特征的通用计算场景中更直接地发挥作用,借助虚拟化技术为诸如人工智能等大规模应用提供更为良好的支持。然而,通用GPU虚拟化技术的实现需要依赖于GPU处理器硬件对并行化多任务处理的支持,以便能够采用更细粒度、更为经济的资源共享方式,充分发挥GPU处理器的性能和能效优势,从而使得GPU处理器能够更高效地融入到未来的高性能计算平台中。. 本项目以通用GPU虚拟化所依赖的并行化多任务硬件支撑技术为研究目标。按照预期的研究计划,本项目从存储架构的设计和优化为切入点,从架构支撑、性能提升和应用优化等多个角度开展对并行化多任务硬件支持的研究。本项目首先立足于新型存储系统的架构设计,以应对现有存储架构所面临的存储容量、通信带宽以及延时等挑战;进而探索通用GPU处理器中存储系统管理机制和运行框架的优化方法,以实现多任务并行化处理时对计算、存储资源的高效共享;同时结合针对典型应用的分析,优化通用GPU处理器进行多任务处理时的性能和功耗。本项目的研究成果有助于在体系结构层面上建立存储架构对并行化多任务处理的硬件支持,突破通用GPU处理器计算虚拟化的关键技术,为未来更高能效的存储系统设计提供参考。.
项目成果
期刊论文数量(6)
专著数量(0)
科研奖励数量(0)
会议论文数量(8)
专利数量(4)
IBOM: An Integrated and Balanced On-Chip Memory for High Performance GPGPUs
IBOM:用于高性能 GPGPU 的集成且平衡的片上存储器
- DOI:10.1109/tpds.2017.2773516
- 发表时间:2018
- 期刊:IEEE Transactions on Parallel and Distributed Systems (TPDS)
- 影响因子:--
- 作者:Wang Jianfei;Wang Qin;Jiang Li;Li Chao;Liang Xiaoyao;Jing Naifeng
- 通讯作者:Jing Naifeng
Buddy SM: Sharing Pipeline Front-End for Improved Energy Efficiency in GPGPUs
Buddy SM:共享管道前端以提高 GPGPU 的能源效率
- DOI:10.1145/2744202
- 发表时间:2015
- 期刊:Acm Transactions ON Architecture and Code Optimization
- 影响因子:1.6
- 作者:Zhang Tao;Jing Naifeng;Jiang Kaiming;Shu Wei;Wu Min-You;Liang Xiaoyao
- 通讯作者:Liang Xiaoyao
Energy-Efficient eDRAM-Based On-Chip Storage Architecture for GPGPUs
适用于 GPGPU 的基于 eDRAM 的节能片上存储架构
- DOI:10.1109/tc.2015.2417545
- 发表时间:2016
- 期刊:IEEE Transactions on Computers (TC)
- 影响因子:--
- 作者:Jing Naifeng;Jiang Li;Zhang Tao;Li Chao;Fan Fengfeng;Liang Xiaoyao
- 通讯作者:Liang Xiaoyao
Incorporating selective victim cache into GPGPU for high-performance computing
将选择性受害者缓存合并到 GPGPU 中以实现高性能计算
- DOI:--
- 发表时间:2017
- 期刊:Concurrency and Computation: Practice and Experience (CCPE)
- 影响因子:--
- 作者:Jianfen Wang;Fengfeng Fan;Li Jiang;Xiaoyao Liang;Naifeng Jing
- 通讯作者:Naifeng Jing
Bank Stealing for a Compact and Efficient Register File Architecture in GPGPU
GPGPU 中紧凑高效的寄存器文件架构的银行窃取
- DOI:10.1109/tvlsi.2016.2584623
- 发表时间:2017
- 期刊:IEEE Transactions on Very Large Scale Integration (VLSI) Systems (TVLSI)
- 影响因子:--
- 作者:Jing Naifeng;Jiang Shunning;Chen Shuang;Zhang Jingjie;Jiang Li;Li Chao;Liang Xiaoyao
- 通讯作者:Liang Xiaoyao
数据更新时间:{{ journalArticles.updateTime }}
{{
item.title }}
{{ item.translation_title }}
- DOI:{{ item.doi || "--"}}
- 发表时间:{{ item.publish_year || "--" }}
- 期刊:{{ item.journal_name }}
- 影响因子:{{ item.factor || "--"}}
- 作者:{{ item.authors }}
- 通讯作者:{{ item.author }}
数据更新时间:{{ journalArticles.updateTime }}
{{ item.title }}
- 作者:{{ item.authors }}
数据更新时间:{{ monograph.updateTime }}
{{ item.title }}
- 作者:{{ item.authors }}
数据更新时间:{{ sciAawards.updateTime }}
{{ item.title }}
- 作者:{{ item.authors }}
数据更新时间:{{ conferencePapers.updateTime }}
{{ item.title }}
- 作者:{{ item.authors }}
数据更新时间:{{ patent.updateTime }}
其他文献
其他文献
{{
item.title }}
{{ item.translation_title }}
- DOI:{{ item.doi || "--" }}
- 发表时间:{{ item.publish_year || "--"}}
- 期刊:{{ item.journal_name }}
- 影响因子:{{ item.factor || "--" }}
- 作者:{{ item.authors }}
- 通讯作者:{{ item.author }}
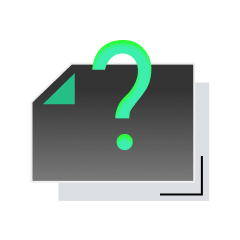
内容获取失败,请点击重试
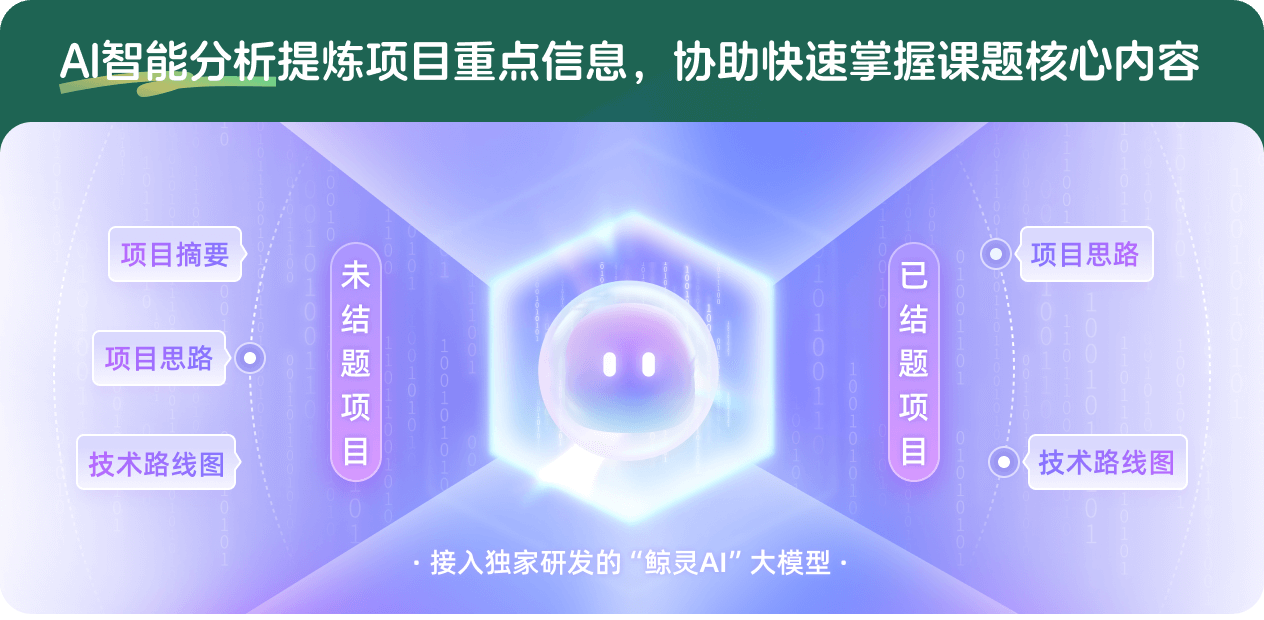
查看分析示例
此项目为已结题,我已根据课题信息分析并撰写以下内容,帮您拓宽课题思路:
AI项目摘要
AI项目思路
AI技术路线图
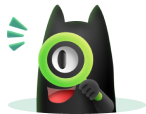
请为本次AI项目解读的内容对您的实用性打分
非常不实用
非常实用
1
2
3
4
5
6
7
8
9
10
您认为此功能如何分析更能满足您的需求,请填写您的反馈:
景乃锋的其他基金
基于动态预测的超并行存内计算新架构设计关键技术研究
- 批准号:92264108
- 批准年份:2022
- 资助金额:74.00 万元
- 项目类别:重大研究计划
基于阻变器件的存储计算深度融合多尺度可重构架构设计研究
- 批准号:61772331
- 批准年份:2017
- 资助金额:62.0 万元
- 项目类别:面上项目
相似国自然基金
{{ item.name }}
- 批准号:{{ item.ratify_no }}
- 批准年份:{{ item.approval_year }}
- 资助金额:{{ item.support_num }}
- 项目类别:{{ item.project_type }}
相似海外基金
{{
item.name }}
{{ item.translate_name }}
- 批准号:{{ item.ratify_no }}
- 财政年份:{{ item.approval_year }}
- 资助金额:{{ item.support_num }}
- 项目类别:{{ item.project_type }}