基于神经网络模型和遗传算法构建院内心跳骤停风险预测模型
项目介绍
AI项目解读
基本信息
- 批准号:81901929
- 项目类别:青年科学基金项目
- 资助金额:21.0万
- 负责人:
- 依托单位:
- 学科分类:H1603.心肺复苏
- 结题年份:2022
- 批准年份:2019
- 项目状态:已结题
- 起止时间:2020-01-01 至2022-12-31
- 项目参与者:--
- 关键词:
项目摘要
In-hospital cardiac arrest is a major cause of hospital death, which can lead to medical dispute. Early prediction of cardiac arrest can help to prevent or prepare to rescue the patients. There is variety prediction models being developed for the prediction of in-hospital cardiac arrest, which however is developed with conventional generalized linear model, with its inherent limitations such as local optimal, requirement of linear assumption. These downsides limited their prediction accuracy, which is at best to be 70-80%. A retrospective cohort will be extracted.from the electronic healthcare records. Enrolled patients are those at high risk of in-hospital cardiac arrest (age>60 years old, renal insufficiency, myocardial infarction or ischemia, organ dysfunctions). In-hospital information are extracted from laboratory, medical imaging, electronic healthcare records, and bedside monitoring systems within 24 hours after hospital admission. Neural network model will be developed with genetic algorithm for the prediction of cardiac arrest. The model will be validated in a prospective cohort. The prediction accuracy in the prospective cohort is expected to be over 90%.
院内心跳骤停(in-hospital cardiac arrest)是住院患者意外死亡的一大重要因素,如能早期预测患者发生心跳骤停,则能及时采取预防和干预措施,提高救治成功率。目前国内外研究学者开发出了不少预测院内心跳骤停的模型,但这些算法大都基于广义线性模型,不能充分考虑危险因素间的交互,容易陷入局部最优,因此这类模型精度在70~80%左右。我们选取存在心跳骤停的高危住院患者,入选标准包括:年龄>60岁;心梗/心肌缺血表现;合并有脏器功能不全的;脑血管意外。从电子病历中提取这些患者数据信息(信息来自检验系统、医学影像系统、His系统、血压监测仪、血氧监测仪等),这些数据信息采集时间窗限定于入院24小时内。用遗传算法对结局事件有影响的变量进行全局筛查,同时在遗传算法中嵌入神经网络模型,拟合获得的模型在前瞻性队列中加以验证,期望外部验证准确率90%以上。
结项摘要
院内心跳骤停(in-hospital cardiac arrest)是住院患者意外死亡的一大重要因素,如能早期预测患者发生心跳骤停,则能及时采取预防和干预措施,提高救治成功率。我们选取存在心跳骤停的高危住院患者,入选标准包括:年龄>60岁;心梗/心肌缺血表现;合并有脏器功能不全的;脑血管意外。用遗传算法对结局事件有影响的变量进行全局筛查,同时在遗传算法中嵌入神经网络模型,拟合获得的模型在前瞻性队列中加以验证,期望外部验证准确率90%以上。在研究期间我们获取了2500例的院内心脏骤停高危患者,在院期间发生心脏骤停次数为121次,我们从电子病历中提取这些患者数据信息(信息来自检验系统、医学影像系统、His系统、血压监测仪、血氧监测仪等),这些数据信息采集时间窗限定于入院24小时内。并训练了预测模型,内部验证该神经网络模型的准确性AUC=83%;其中重要的可用来预测院内心跳骤停的变量包括了年龄、基础疾病累计评分(Charlson评分)、是否合并冠心病基础、是否入住ICU、初始SOFA评分等。另外,我们也对该队列进行了亚组分析,把人群限制在有ARDS的患者,并用遗传算法对结局事件有影响的变量进行全局筛查,同时在遗传算法中嵌入神经网络模型,结果获得了一个较好的预测死亡结局的神经网络模型。该模型的内部准确性达到了0.743,而传统的APACHE III评分其准确性仅为0.665.
项目成果
期刊论文数量(2)
专著数量(0)
科研奖励数量(0)
会议论文数量(0)
专利数量(0)
Analytics with artificial intelligence to advance the treatment of acute respiratory distress syndrome
人工智能分析促进急性呼吸窘迫综合征的治疗
- DOI:10.1111/jebm.12418
- 发表时间:2020-11-01
- 期刊:JOURNAL OF EVIDENCE BASED MEDICINE
- 影响因子:7.3
- 作者:Zhang, Zhongheng;Navarese, Eliano Pio;Ma, Xuelei
- 通讯作者:Ma, Xuelei
Defining persistent critical illness based on growth trajectories in patients with sepsis
根据脓毒症患者的生长轨迹定义持续性危重疾病
- DOI:10.1186/s13054-020-2768-z
- 发表时间:2020-02-18
- 期刊:CRITICAL CARE
- 影响因子:15.1
- 作者:Zhang, Zhongheng;Ho, Kwok M.;Yu, Yunsong
- 通讯作者:Yu, Yunsong
数据更新时间:{{ journalArticles.updateTime }}
{{
item.title }}
{{ item.translation_title }}
- DOI:{{ item.doi || "--"}}
- 发表时间:{{ item.publish_year || "--" }}
- 期刊:{{ item.journal_name }}
- 影响因子:{{ item.factor || "--"}}
- 作者:{{ item.authors }}
- 通讯作者:{{ item.author }}
数据更新时间:{{ journalArticles.updateTime }}
{{ item.title }}
- 作者:{{ item.authors }}
数据更新时间:{{ monograph.updateTime }}
{{ item.title }}
- 作者:{{ item.authors }}
数据更新时间:{{ sciAawards.updateTime }}
{{ item.title }}
- 作者:{{ item.authors }}
数据更新时间:{{ conferencePapers.updateTime }}
{{ item.title }}
- 作者:{{ item.authors }}
数据更新时间:{{ patent.updateTime }}
其他文献
Meta流行病学的产生与发展
- DOI:10.7507/1672-2531.202112135
- 发表时间:2022
- 期刊:中国循证医学杂志
- 影响因子:--
- 作者:龙囿霖;林春滢;郭嘉琪;王心怡;杨柳;程祎凡;黄进;杨克虎;章仲恒;徐畅;商洪才;杜亮
- 通讯作者:杜亮
Meta流行病学研究的报告规范解读
- DOI:--
- 发表时间:2022
- 期刊:中国循证医学杂志
- 影响因子:--
- 作者:郭嘉琪;龙囿霖;林春滢;王心怡;郭琼;林逸飞;黄进;杨克虎;章仲恒;徐畅;商洪才;杜亮
- 通讯作者:杜亮
其他文献
{{
item.title }}
{{ item.translation_title }}
- DOI:{{ item.doi || "--" }}
- 发表时间:{{ item.publish_year || "--"}}
- 期刊:{{ item.journal_name }}
- 影响因子:{{ item.factor || "--" }}
- 作者:{{ item.authors }}
- 通讯作者:{{ item.author }}
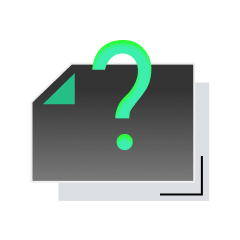
内容获取失败,请点击重试
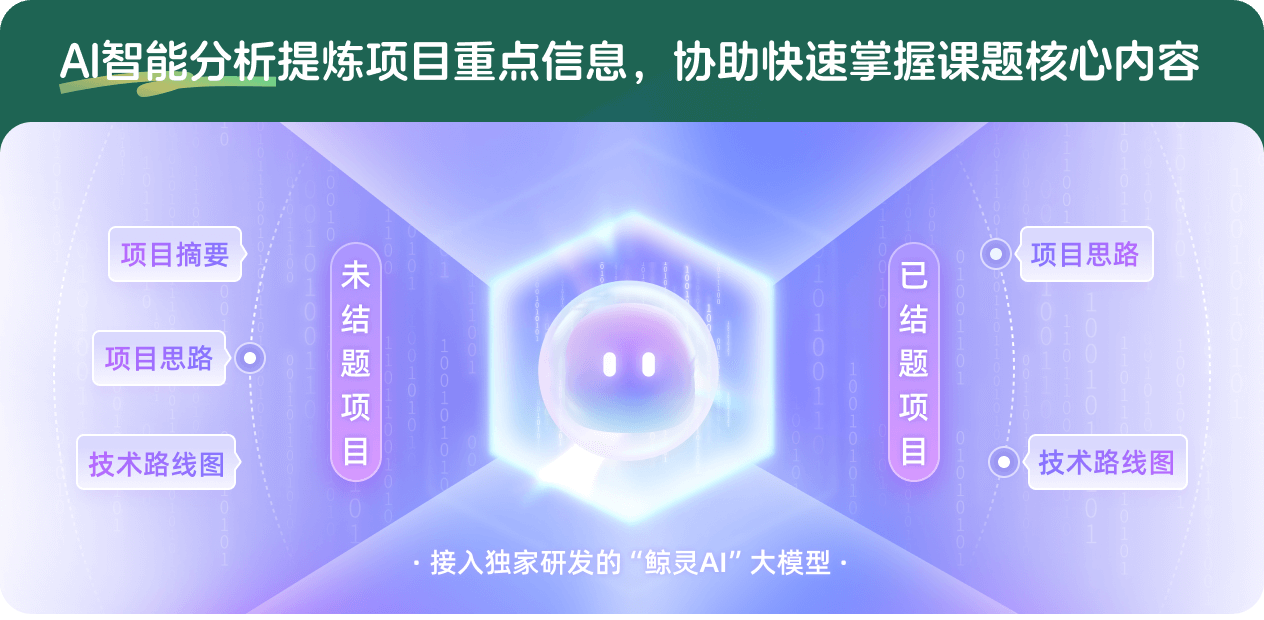
查看分析示例
此项目为已结题,我已根据课题信息分析并撰写以下内容,帮您拓宽课题思路:
AI项目摘要
AI项目思路
AI技术路线图
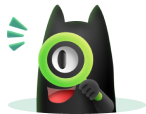
请为本次AI项目解读的内容对您的实用性打分
非常不实用
非常实用
1
2
3
4
5
6
7
8
9
10
您认为此功能如何分析更能满足您的需求,请填写您的反馈:
章仲恒的其他基金
基于转录组学和人工智能的脓毒症激素精准化治疗的研究
- 批准号:
- 批准年份:2022
- 资助金额:52 万元
- 项目类别:面上项目
基于转录组学和人工智能的脓毒症激素精准化治疗的研究
- 批准号:82272180
- 批准年份:2022
- 资助金额:52.00 万元
- 项目类别:面上项目
相似国自然基金
{{ item.name }}
- 批准号:{{ item.ratify_no }}
- 批准年份:{{ item.approval_year }}
- 资助金额:{{ item.support_num }}
- 项目类别:{{ item.project_type }}
相似海外基金
{{
item.name }}
{{ item.translate_name }}
- 批准号:{{ item.ratify_no }}
- 财政年份:{{ item.approval_year }}
- 资助金额:{{ item.support_num }}
- 项目类别:{{ item.project_type }}