基于温度场时空特性的选区激光熔化过程监测方法研究
项目介绍
AI项目解读
基本信息
- 批准号:61803023
- 项目类别:青年科学基金项目
- 资助金额:25.0万
- 负责人:
- 依托单位:
- 学科分类:F0308.智能制造自动化系统理论与技术
- 结题年份:2021
- 批准年份:2018
- 项目状态:已结题
- 起止时间:2019-01-01 至2021-12-31
- 项目参与者:黄明吉; 牛玉玲; 姚姝悦; 张万松; 张钧; 赵雪;
- 关键词:
项目摘要
Selective laser melting process monitoring is aimed to, during the metal part building process, determine and predict the actual state of the powder bed and molten pool by measuring and analyzing the temperature field; and it is one of the key technologies which provide supports for closed-loop control and quality assurance of the building process. The temperature field can quantitatively reflect the state of the powder bed and molten pool from time and space aspects; however, the existing research mostly focus on the static analysis of the images of the temperature field in the building process, in order to explore and verify the correlations between the powder bed, the molten pool and the temperature field; it is still lack of support means for dynamic analysis and online monitoring of the states of the powder bed and molten pool. In this project, the time-varying and spatial distribution characteristics of the temperature field will be analyzed from the perspective of big data, and the data mining methods based on the key features of the temperature field will be proposed. On the basis of frequent pattern mining algorithm and Bayesian classifier, this project research and build a semi supervised learning algorithm model, which based on the accumulated measurement data of the key features of the temperature field, learns and optimizes the classification rules of building process states, and then implements the dynamic determination and abnormal prediction of the states of the powder bed and molten pool according to those rules. Finally, on the foundation of the actual production process, experiments will be carried out from four aspects which include single manufacturing process, batch manufacturing process, across batches and across part types, to verify the feasibility of the proposed methods.
选区激光熔化过程监测是指,在金属零件成型过程中对粉末铺层与熔池的实际状态进行测量、分析与异常预测,是支持成型过程闭环控制与质量保证的关键技术之一。温度场能够在时间和空间上量化表征成型过程中粉末铺层与熔池的实时状态;然而,现有研究多为对成型过程中的温度场图像进行静态分析,以探索和验证温度场与粉末铺层、熔池状态间关联关系为主,尚缺乏面向粉末铺层与熔池状态动态分析与在线监测的支撑手段。本项目拟从大数据角度分析温度场的时变性和空间分布特性,研究面向温度场关键特征的数据挖掘方法;基于频繁模式挖掘和贝叶斯分类器,研究并构建半监督学习算法模型,该模型基于不断累积的温度场关键特征的测量数据,自主学习和优化成型过程状态分类规则,并依据该规则对粉末铺层与熔池的当前状态进行动态判定和异常预测;最后,以实际生产过程为依托,从单一制造过程、批次制造过程、跨批次和跨零件类型四个角度,对所提出的监测方法进行试验验证。
结项摘要
在金属增材制造过程中对粉末铺层与熔池的实际状态进行测量、分析与异常预测,是支持成型过程闭环控制与质量保证的关键技术之一。本项目针对温度场分析与增材制造过程质量监测方法开展研究:定义了温度场关键特征,构建了多层多通道增材制造过程数值仿真模型,基于大量数值仿真试验和实际试验采集不同工艺参数下增材制造过程的温度场数据与零件质量检测数据,通过多维度数据分析,揭示了温度场时空变化规律及其与工艺参数、零件质量间的关联关系,提出了基于温度场关键特征的增材制造过程工艺参数评估算法、基于响应曲面的工艺参数优化方法、基于温度场的零件硬度分布状态预测算法等,试验结果表明,算法预测结果与实际数据较为一致,能较为准确的判断增材制造过程质量状态,解决了通过破坏性测试手段检测零件质量的低效率、低精度的问题,也为工艺参数控制提供参考;提出了基于特征融合的粉末床单一缺陷检测算法和基于深度学习的多缺陷实时检测算法,研究了面向增材制造设备的模块化智能检测单元的实现方案,针对低性能、低功耗的视觉终端,对算法模型进行了轻量化,实现智能算法的终端部署与运行,进而构建了面向增材制造过程实时监测的模块化视觉检测装置样机,通过试验分析了不同照明策略对数据采集和检测准确性的影响,所开发的算法能够在15.67毫秒内精确检测出粉末床缺陷,检测准确率能够达到99.4%,算法基于小样本进行训练,能够极大地减少人工标注的时间和成本。项目研究的相关算法能够应用于增材制造过程监测领域,并且有望形成一套可以产品化的智能实时监测视觉终端,以选配模块的形式与不同增材制造设备集成,具有较为广阔的应用前景。另外,本项目研究成果形成了7篇学术论文,取得了2项授权发明专利,培养了博士研究生1人,硕士研究生4人。
项目成果
期刊论文数量(5)
专著数量(0)
科研奖励数量(0)
会议论文数量(0)
专利数量(2)
基于图像特征融合的粉末床缺陷检测方法
- DOI:--
- 发表时间:2020
- 期刊:航空学报
- 影响因子:--
- 作者:师彬彬;陈哲涵
- 通讯作者:陈哲涵
A layer-wise multi-defect detection system for powder bed monitoring: Lighting strategy for imaging, adaptive segmentation and classification
用于粉床监测的分层多缺陷检测系统:成像照明策略、自适应分割和分类
- DOI:10.1016/j.matdes.2021.110035
- 发表时间:2021-08-11
- 期刊:MATERIALS & DESIGN
- 影响因子:8.4
- 作者:Shi, Binbin;Chen, Zhehan
- 通讯作者:Chen, Zhehan
Hardness Prediction and Verification Based on Key Temperature Features During the Directed Energy Deposition Process
基于定向能量沉积过程中关键温度特征的硬度预测和验证
- DOI:10.1007/s40684-020-00208-4
- 发表时间:2020-03
- 期刊:International Journal of Precision Engineering and Manufacturing-Green Technology
- 影响因子:4.2
- 作者:Chen Zhehan;Guo Xinxin;Shi Jing
- 通讯作者:Shi Jing
Online Monitoring Based on Temperature Field Features and Prediction Model for Selective Laser Sintering Process
基于温度场特征和预测模型的选择性激光烧结过程在线监测
- DOI:10.3390/app8122383
- 发表时间:2018-12-01
- 期刊:APPLIED SCIENCES-BASEL
- 影响因子:2.7
- 作者:Chen, Zhehan;Zong, Xianhui;Zhang, Xiaohua
- 通讯作者:Zhang, Xiaohua
激光增材制造过程数值仿真技术综述
- DOI:--
- 发表时间:2020
- 期刊:航空学报
- 影响因子:--
- 作者:郭鑫鑫;陈哲涵
- 通讯作者:陈哲涵
数据更新时间:{{ journalArticles.updateTime }}
{{
item.title }}
{{ item.translation_title }}
- DOI:{{ item.doi || "--"}}
- 发表时间:{{ item.publish_year || "--" }}
- 期刊:{{ item.journal_name }}
- 影响因子:{{ item.factor || "--"}}
- 作者:{{ item.authors }}
- 通讯作者:{{ item.author }}
数据更新时间:{{ journalArticles.updateTime }}
{{ item.title }}
- 作者:{{ item.authors }}
数据更新时间:{{ monograph.updateTime }}
{{ item.title }}
- 作者:{{ item.authors }}
数据更新时间:{{ sciAawards.updateTime }}
{{ item.title }}
- 作者:{{ item.authors }}
数据更新时间:{{ conferencePapers.updateTime }}
{{ item.title }}
- 作者:{{ item.authors }}
数据更新时间:{{ patent.updateTime }}
其他文献
基于关键测量特性的飞机装配检测数据建模研究
- DOI:--
- 发表时间:--
- 期刊:航空学报
- 影响因子:--
- 作者:陈哲涵;杜福洲;唐晓青
- 通讯作者:唐晓青
选区激光烧结收缩率预测及工艺参数优化
- DOI:10.16865/j.cnki.1000-7555.2018.06.020
- 发表时间:2018
- 期刊:高分子材料科学与工程
- 影响因子:--
- 作者:贺可太;刘硕;陈哲涵;杨智
- 通讯作者:杨智
测量驱动的飞机部件数字化对接系统实现技术研究
- DOI:--
- 发表时间:--
- 期刊:航空制造技术
- 影响因子:--
- 作者:杜福洲;陈哲涵
- 通讯作者:陈哲涵
iGPS测量场精度分析及其应用研究
- DOI:--
- 发表时间:--
- 期刊:航空学报
- 影响因子:--
- 作者:杜福洲;陈哲涵;唐晓青
- 通讯作者:唐晓青
其他文献
{{
item.title }}
{{ item.translation_title }}
- DOI:{{ item.doi || "--" }}
- 发表时间:{{ item.publish_year || "--"}}
- 期刊:{{ item.journal_name }}
- 影响因子:{{ item.factor || "--" }}
- 作者:{{ item.authors }}
- 通讯作者:{{ item.author }}
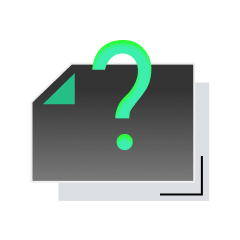
内容获取失败,请点击重试
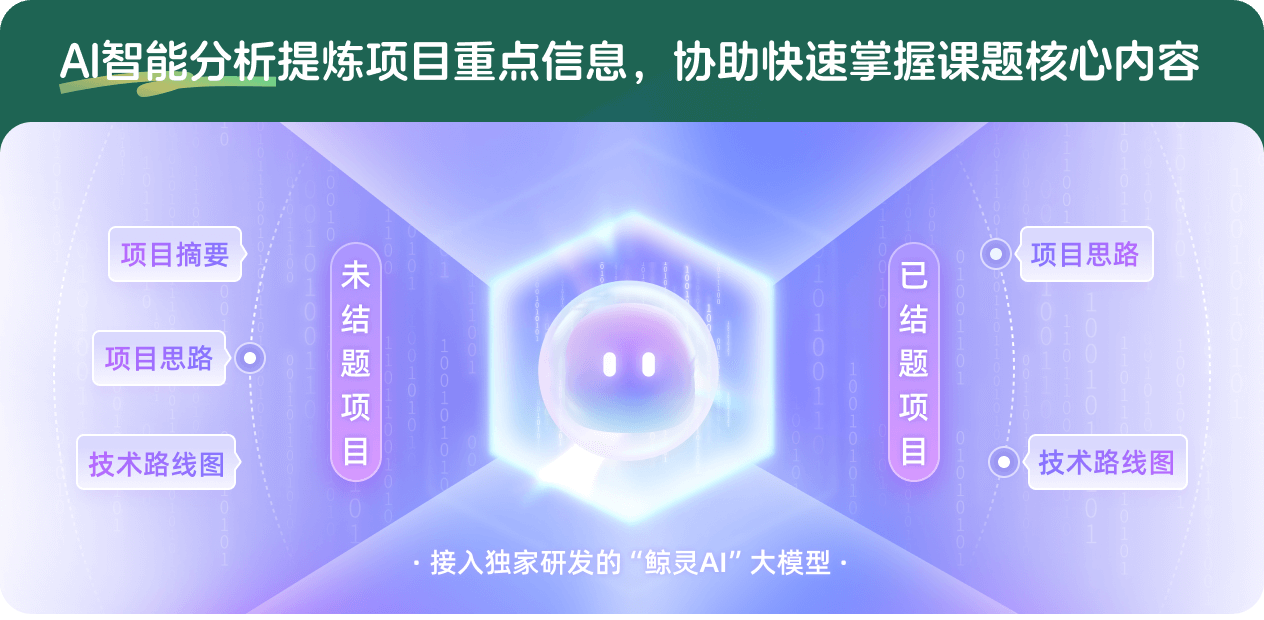
查看分析示例
此项目为已结题,我已根据课题信息分析并撰写以下内容,帮您拓宽课题思路:
AI项目摘要
AI项目思路
AI技术路线图
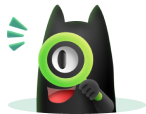
请为本次AI项目解读的内容对您的实用性打分
非常不实用
非常实用
1
2
3
4
5
6
7
8
9
10
您认为此功能如何分析更能满足您的需求,请填写您的反馈:
相似国自然基金
{{ item.name }}
- 批准号:{{ item.ratify_no }}
- 批准年份:{{ item.approval_year }}
- 资助金额:{{ item.support_num }}
- 项目类别:{{ item.project_type }}
相似海外基金
{{
item.name }}
{{ item.translate_name }}
- 批准号:{{ item.ratify_no }}
- 财政年份:{{ item.approval_year }}
- 资助金额:{{ item.support_num }}
- 项目类别:{{ item.project_type }}