最熟悉的陌生人:一种基于相遇模式挖掘与非确定性规划的车联网数据传递方法
项目介绍
AI项目解读
基本信息
- 批准号:61300178
- 项目类别:青年科学基金项目
- 资助金额:23.0万
- 负责人:
- 依托单位:
- 学科分类:F0208.物联网及其他新型网络
- 结题年份:2016
- 批准年份:2013
- 项目状态:已结题
- 起止时间:2014-01-01 至2016-12-31
- 项目参与者:廖乐健; 陆慧梅; 王明钟; 李杨; 王超; 张鸿燕; 付茂才; 王成远;
- 关键词:
项目摘要
Vehicular networking is considered to be a very promising and prosperous market, which contains many business opportunities and is of great research value. For most vehicular applications, the data delivery mechanism is the key technology. In literature, the vehicular mobility prediction has been adopted for the data dissemination by considering the road layout and the human behavior simultaneously. However, the conventional mobility prediction focused on the individual tracks and is lack of the analysis of the human behavior patterns and the similarities of the social attributes which lead to the "occasionally" encounters. After conducting a thorough investigation of vehicular data dissemination algorithms, we propose a time dependent encountering analysis-based mobility prediction method, where the graph model is used to exploit the mobility over the real historical data. Then we propose a novel data dissemination method based on sequential decision making algorithom which optimize the problem to obtain the best action in terms of the data delivery rate and the average delay by considering the exploited mobility (the proper node for the next hop) for the data delivery. We will also develop the simulation system to verify the proposed methods.
车联网市场应用前景广阔,蕴含着巨大的商机和研究价值,其中车联网中的数据传递机制是众多应用的核心技术。由于城市道路的限制和人类行为模式的影响,基于车辆行驶轨迹预测的车联网数据传递方法被普遍认为具有良好的前景。但传统的轨迹预测方法倾向于对个体轨迹的独立分析和判断,忽略了相遇模式背后潜在的人类行为共性和社会属性的相似性对行驶轨迹的影响。本项目充分分析了车联网数据传递算法的研究现状,依据车联网真实数据提出了一种面向相遇模式挖掘的、与时间相关的轨迹预测模型;以预测的轨迹、数据投递率、时间延迟为参数和优化条件,提出一种基于非确定性序贯决策模型的车联网数据传递新方法;并通过搭建仿真验证平台,对提出的模型和算法进行分析和评价。
结项摘要
近年来由于城镇居民收入水平的提高,私家车辆大量普及,推动了车联网这一新兴网络系统的发展。车联网市场应用前景广阔,蕴含着巨大的商机和研究价值,其中车联网中的数据传递机制是众多应用的核心技术。由于城市道路和人类行为模式的限制,基于行驶轨迹预测的数据传递是车联网数据传输方法中很有价值的一类。但是传统轨迹预测方法更倾向于对个体轨迹的独立分析和判断,忽略了相遇模式背后潜在的人类行为共性和社会属性的相似性对轨迹预测的指导作用,本项目充分分析了车联网数据传递算法的研究现状,依据大规模真实数据(1)提出一系列兴趣地点预测模型包括:基于群体和个体倾向的地点预测模型、时间敏感的地点预测模型、基于隐模式的地点预测模型。从理论分析和实验验证我们所提出来的基于群体数据预测个体行为轨迹特征的思想的有效性和可行性;(2)以预测的轨迹、投递率、时间延迟作为参数和优化条件,提出了一种基于多目标优化的非确定性序贯决策模型的数据传递新方法,该方法针对车联网中数据传递的多目标优化问题,并从理论上分析证明了传输延时与中继节点个数的反相关性,首次指出同时优化传输时延与跳数的不合理性,并为其提供了一种用户定制的折中解决方案;(3)提出了一种基于车辆轨迹密度的路侧单元布置算法,该方法可以通过车流量和轨迹信息动态的调整路侧单元的工作模式,进行工作调度,以节约能源及成本;(4)提出一种基于城市道路网信息和历史轨迹的车辆轨迹预测方法,并从安全性的角度对提出的模型和算法进行分析和评价,为车联网中存在的位置隐私问题提供了保护机制。 ..本项目在国际期刊和国际会议发表学术论文17篇:SCI收录3篇,EI收录14篇,其中包括领域CCF A类国际会议AAAI、IJCAI、CCF推荐C类著名国际会议IPCCC、APWeb、WASA、KSEM、APNOMs国际期刊Neurocomputing、Mobile Information Science、国内核心期刊《电子学报》等。
项目成果
期刊论文数量(4)
专著数量(0)
科研奖励数量(1)
会议论文数量(13)
专利数量(0)
Effective successive POI recommendation inferred with individual behavior and group preference
根据个人行为和群体偏好推断出有效的连续 POI 推荐
- DOI:10.1016/j.neucom.2015.10.146
- 发表时间:2016-10-19
- 期刊:NEUROCOMPUTING
- 影响因子:6
- 作者:Chen, Jialiang;Li, Xin;Li, Kan
- 通讯作者:Li, Kan
基于双语词典的微博多类情感分析方法
- DOI:--
- 发表时间:--
- 期刊:电子学报
- 影响因子:--
- 作者:礼欣;韩旭;宋丹丹;廖乐健
- 通讯作者:廖乐健
数据更新时间:{{ journalArticles.updateTime }}
{{
item.title }}
{{ item.translation_title }}
- DOI:{{ item.doi || "--"}}
- 发表时间:{{ item.publish_year || "--" }}
- 期刊:{{ item.journal_name }}
- 影响因子:{{ item.factor || "--"}}
- 作者:{{ item.authors }}
- 通讯作者:{{ item.author }}
数据更新时间:{{ journalArticles.updateTime }}
{{ item.title }}
- 作者:{{ item.authors }}
数据更新时间:{{ monograph.updateTime }}
{{ item.title }}
- 作者:{{ item.authors }}
数据更新时间:{{ sciAawards.updateTime }}
{{ item.title }}
- 作者:{{ item.authors }}
数据更新时间:{{ conferencePapers.updateTime }}
{{ item.title }}
- 作者:{{ item.authors }}
数据更新时间:{{ patent.updateTime }}
其他文献
其他文献
{{
item.title }}
{{ item.translation_title }}
- DOI:{{ item.doi || "--" }}
- 发表时间:{{ item.publish_year || "--"}}
- 期刊:{{ item.journal_name }}
- 影响因子:{{ item.factor || "--" }}
- 作者:{{ item.authors }}
- 通讯作者:{{ item.author }}
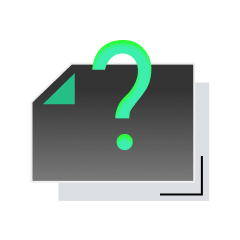
内容获取失败,请点击重试
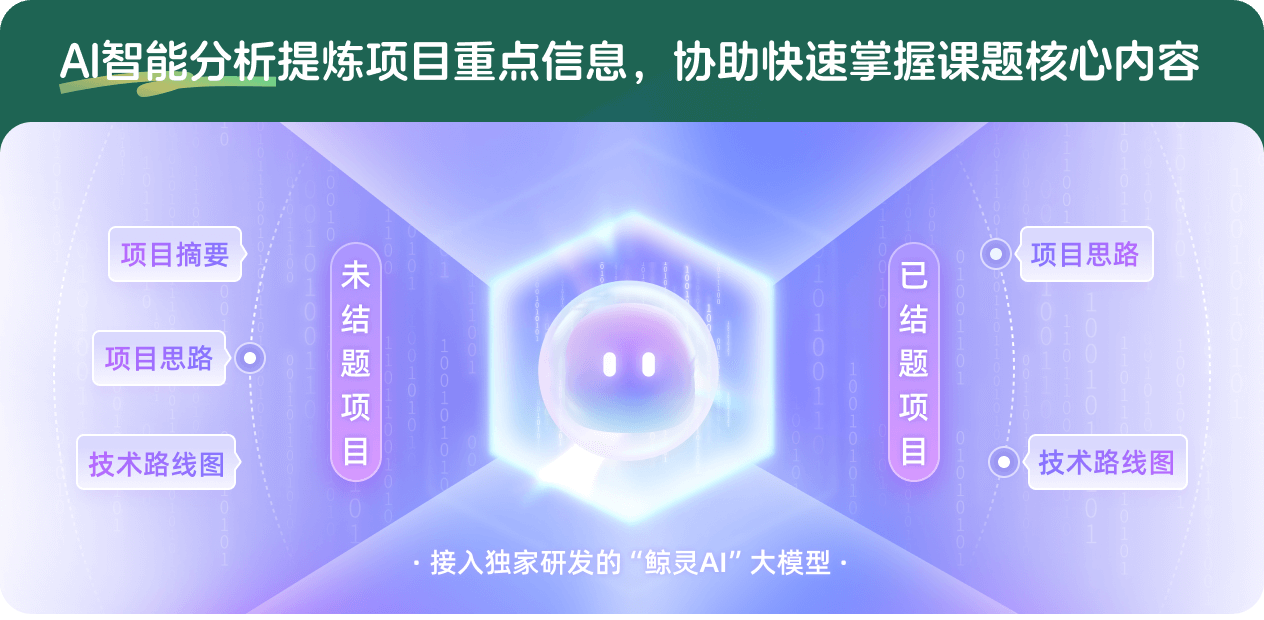
查看分析示例
此项目为已结题,我已根据课题信息分析并撰写以下内容,帮您拓宽课题思路:
AI项目摘要
AI项目思路
AI技术路线图
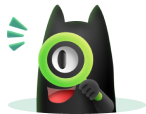
请为本次AI项目解读的内容对您的实用性打分
非常不实用
非常实用
1
2
3
4
5
6
7
8
9
10
您认为此功能如何分析更能满足您的需求,请填写您的反馈:
礼欣的其他基金
面向可泛化深度强化学习的关键技术研究
- 批准号:62276024
- 批准年份:2022
- 资助金额:54.00 万元
- 项目类别:面上项目
知识数据双驱动的深度强化学习技术研究
- 批准号:
- 批准年份:2022
- 资助金额:80 万元
- 项目类别:
面向可泛化深度强化学习的关键技术研究
- 批准号:92270125
- 批准年份:2022
- 资助金额:54 万元
- 项目类别:面上项目
面向多标签网络的嵌入式表示、对齐及其应用研究
- 批准号:61772074
- 批准年份:2017
- 资助金额:62.0 万元
- 项目类别:面上项目
相似国自然基金
{{ item.name }}
- 批准号:{{ item.ratify_no }}
- 批准年份:{{ item.approval_year }}
- 资助金额:{{ item.support_num }}
- 项目类别:{{ item.project_type }}
相似海外基金
{{
item.name }}
{{ item.translate_name }}
- 批准号:{{ item.ratify_no }}
- 财政年份:{{ item.approval_year }}
- 资助金额:{{ item.support_num }}
- 项目类别:{{ item.project_type }}