三维道路场景下多特征的车联网中继节点选择方法研究
项目介绍
AI项目解读
基本信息
- 批准号:61902041
- 项目类别:青年科学基金项目
- 资助金额:28.0万
- 负责人:
- 依托单位:
- 学科分类:F0214.新型计算及其应用基础
- 结题年份:2022
- 批准年份:2019
- 项目状态:已结题
- 起止时间:2020-01-01 至2022-12-31
- 项目参与者:--
- 关键词:
项目摘要
The relay-node selection in vehicular networks is one of the key basic technologies of Intelligent Transportation. And the introduction of road information can significantly improve the performance of this selection. In view of the current lack of the relay-node selection method with 3D road information introduced effectively, this project will face the complexity of both 3D road structure and wireless communication environment, aim at the relay-node selection with ultra-low latency and high reliability, and do research as follows. 1) To break through the limitations of the relay-node selection based on the single road feature, establishing a three-dimensional road-feature system with quantitative description. And constructing a performance-maximizing multi-objective optimization model constrained by multiple factors in the actual scene; 2) For the characteristics that the multiple levels of the three-dimensional road interlace, road structures compound and the communication ranges of different levels are varied, a new method of relay-node selection based on three-dimensional road-feature system is proposed to realize ultra-low delay and high reliability relay node selection in specific scenarios; 3) To face the problem of unstable performance of the relay node selection affected by different factors, the mathematical relationship between message dissemination speed, package delivery rate and environment factors, process factors for the relay-node selection is studied, further a new relay-node selection adapting to environment factors in the 3D road scenarios is developed. The expected results can provide the theoretical basis and technical support for fully autonomous driving in the whole road network and promote the large-scale commercial use of the Internet of Vehicles in Intelligent Transportation.
车联网中继节点选择是智能交通的关键基础技术之一,道路结构信息的引入能显著提升节点选择性能。鉴于当前缺乏有效引入三维道路信息的中继节点选择方法,本项目面对三维道路结构和无线通信环境的复杂性,以超低时延且高可靠的中继节点选择为目标,开展研究:1)突破现有以单一道路特征为中继节点选择依据的局限,建立定量描述的三维道路特征体系,并构建切合实际场景的多因素约束的性能最大化多目标优化模型;2)针对三维道路多层交错且结构复合及不同层通信范围差异较大的特点,提出基于三维道路特征体系的中继节点选择新方法,实现特定场景下超低时延、高可靠的中继节点选择;3)应对不同因素影响下中继节点选择性能不稳定的问题,研究环境因素、过程因素与消息传播速度及包投递率之间的关系,提出自适应环境因素的三维道路场景下中继节点选择的新方法。预期成果可为实现全路网自动驾驶提供理论依据和技术支撑,推进智能交通领域高性能车联网的大规模商用。
结项摘要
三维交通网络的提速建设对车联网超低时延性提出了越来越迫切的要求。研究并提出三维道路场景下超低时延且高可靠的中继节点鲁棒选择方法是车联网领域内极具挑战性、且迫切需要解决的问题之一。本项目针对实际三维复杂道路场景下车联网中继节点的选择展开研究,具体研究内容和工作包括:1)建立了车联网中约束车辆行驶的三维路网模型及刻画不同三维道路结构的特征值,基于此提出了一种道路结构识别方法;提出了基于BERT(Bidirectional Encoder Representation from Transformers)的深度时空网络模型,并扩展为多任务时序预测模型范式。此部分工作建立了三维道路体系,能实现对城市中车辆需求量的高精度、细粒度预测,从而有利于车辆轨迹的预测,提供中继节点选择的先验信息。2)研究了不同道路场景特点及三维道路上坡度、道路形状、发送功率等因素对三维道路场景下中继节点选择方案的影响,分别提出了适用三维双层直道场景、立交桥场景、弯曲山道场景的中继节点选择方法,能提高消息的传播速度和可靠性。3)提出了一种复杂场景下的自适应中继节点选择方法,通过不同场景下最优位置和通信障碍物造成的空白区的定义,统一了不同场景下中继节点选择的准则,提高了复杂三维道路场景下中继节点的鲁棒性。4)在以上工作基础上,提出了一种在V2X(Vehicle to Everything)下多协同节点串行卸载、并行计算的分布式卸载策略,该策略利用车辆可预测的行驶轨迹,对任务进行不等拆分,分布式计算于本地、MEC(Mobile Edge Computing)及协同车辆,目标系统时延的最小化。综上,本研究的工作成果能为车辆轨迹的预测、车-车超短时延的通信、路网数据实时的处理提供关键技术和新的方法,从而促使5G车联网垂直场景应用和完全自动驾驶的落地。
项目成果
期刊论文数量(7)
专著数量(1)
科研奖励数量(2)
会议论文数量(0)
专利数量(7)
Relay Cooperative Transmission Algorithms for IoV Under Aggregated Interference
聚合干扰下车联网中继协同传输算法
- DOI:10.1109/tits.2021.3106545
- 发表时间:2021-09-13
- 期刊:IEEE TRANSACTIONS ON INTELLIGENT TRANSPORTATION SYSTEMS
- 影响因子:8.5
- 作者:Ji, Baofeng;Han, Ying;Wen, Hong
- 通讯作者:Wen, Hong
V2X多节点协同分布式卸载策略
- DOI:--
- 发表时间:2022
- 期刊:通信学报
- 影响因子:--
- 作者:曹敦;张应宝;邹电;王进;汤强;冀保峰
- 通讯作者:冀保峰
A Beacon-less Relay-node Selection Method for 3-D Scenarios in Internet of Vehicle
车联网3D场景无信标中继节点选择方法
- DOI:10.53106/160792642022092305012
- 发表时间:2022-09
- 期刊:Journal of Internet and Technology
- 影响因子:--
- 作者:Dun Cao;Dongwei Li;Osama Alfarraj;Amr Tolba;Gwang-Jun Kim
- 通讯作者:Gwang-Jun Kim
Fast Visual Tracking with Squeeze and Excitation Region Proposal Network
使用挤压和激励区域提议网络进行快速视觉跟踪
- DOI:--
- 发表时间:2023
- 期刊:Human-centric Computing and Information Sciences
- 影响因子:6.6
- 作者:Dun Cao;Renhua Dai;Jin Wang;Osama Alfaeeraj;Amr Tolba;Pradip Kumar Sharma;Min Zhu
- 通讯作者:Min Zhu
BERT-Based Deep Spatial-Temporal Network for Taxi Demand Prediction
基于 BERT 的深度时空网络用于出租车需求预测
- DOI:10.1109/tits.2021.3122114
- 发表时间:2021-10-29
- 期刊:IEEE TRANSACTIONS ON INTELLIGENT TRANSPORTATION SYSTEMS
- 影响因子:8.5
- 作者:Cao, Dun;Zeng, Kai;Zhou, Siyuan
- 通讯作者:Zhou, Siyuan
数据更新时间:{{ journalArticles.updateTime }}
{{
item.title }}
{{ item.translation_title }}
- DOI:{{ item.doi || "--"}}
- 发表时间:{{ item.publish_year || "--" }}
- 期刊:{{ item.journal_name }}
- 影响因子:{{ item.factor || "--"}}
- 作者:{{ item.authors }}
- 通讯作者:{{ item.author }}
数据更新时间:{{ journalArticles.updateTime }}
{{ item.title }}
- 作者:{{ item.authors }}
数据更新时间:{{ monograph.updateTime }}
{{ item.title }}
- 作者:{{ item.authors }}
数据更新时间:{{ sciAawards.updateTime }}
{{ item.title }}
- 作者:{{ item.authors }}
数据更新时间:{{ conferencePapers.updateTime }}
{{ item.title }}
- 作者:{{ item.authors }}
数据更新时间:{{ patent.updateTime }}
其他文献
一种山区环境下车际网的中继节点选择方法
- DOI:--
- 发表时间:2017
- 期刊:计算机工程与科学
- 影响因子:--
- 作者:曹敦;雷正保
- 通讯作者:雷正保
其他文献
{{
item.title }}
{{ item.translation_title }}
- DOI:{{ item.doi || "--" }}
- 发表时间:{{ item.publish_year || "--"}}
- 期刊:{{ item.journal_name }}
- 影响因子:{{ item.factor || "--" }}
- 作者:{{ item.authors }}
- 通讯作者:{{ item.author }}
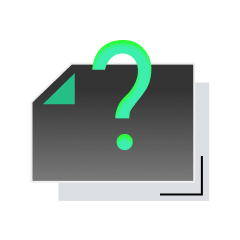
内容获取失败,请点击重试
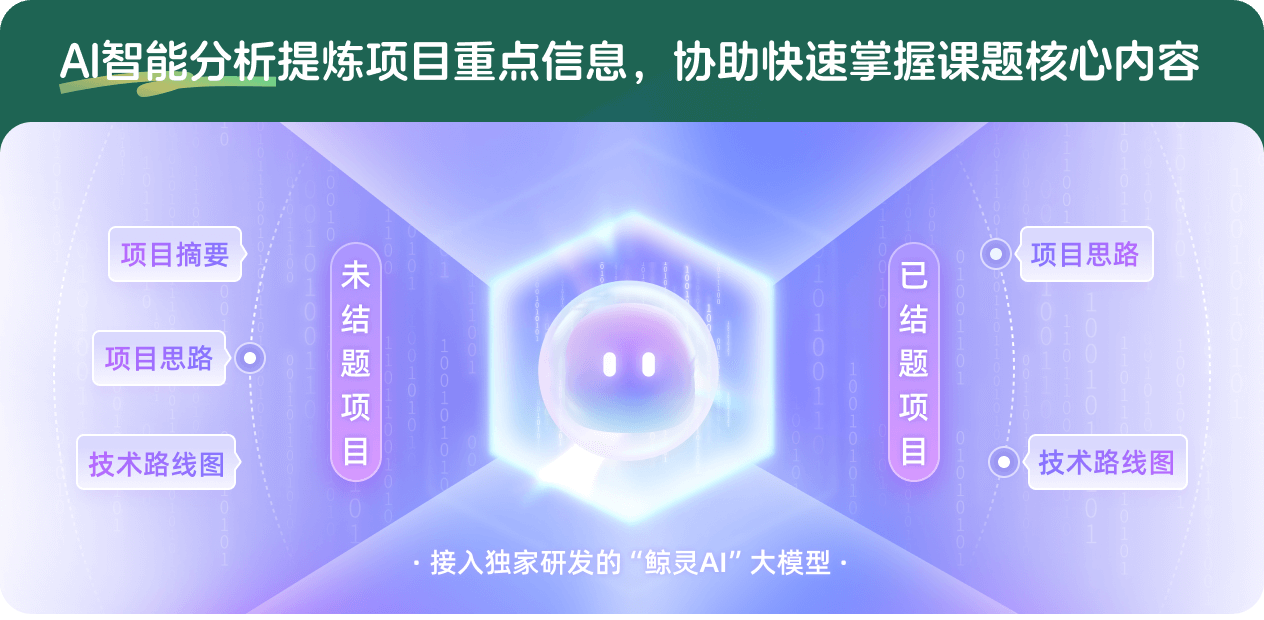
查看分析示例
此项目为已结题,我已根据课题信息分析并撰写以下内容,帮您拓宽课题思路:
AI项目摘要
AI项目思路
AI技术路线图
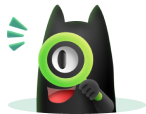
请为本次AI项目解读的内容对您的实用性打分
非常不实用
非常实用
1
2
3
4
5
6
7
8
9
10
您认为此功能如何分析更能满足您的需求,请填写您的反馈:
曹敦的其他基金
面向多源可分解任务的车联网边缘计算资源联邦优化研究
- 批准号:62272063
- 批准年份:2022
- 资助金额:54.00 万元
- 项目类别:面上项目
面向多源可分解任务的车联网边缘计算资源联邦优化研究
- 批准号:
- 批准年份:2022
- 资助金额:54 万元
- 项目类别:面上项目
相似国自然基金
{{ item.name }}
- 批准号:{{ item.ratify_no }}
- 批准年份:{{ item.approval_year }}
- 资助金额:{{ item.support_num }}
- 项目类别:{{ item.project_type }}
相似海外基金
{{
item.name }}
{{ item.translate_name }}
- 批准号:{{ item.ratify_no }}
- 财政年份:{{ item.approval_year }}
- 资助金额:{{ item.support_num }}
- 项目类别:{{ item.project_type }}