机械微弱信号自适应互补随机共振滤波增强检测与故障诊断研究
项目介绍
AI项目解读
基本信息
- 批准号:51605002
- 项目类别:青年科学基金项目
- 资助金额:20.0万
- 负责人:
- 依托单位:
- 学科分类:E0503.机械动力学
- 结题年份:2019
- 批准年份:2016
- 项目状态:已结题
- 起止时间:2017-01-01 至2019-12-31
- 项目参与者:刘方; 杨慧; 李文睿; 苏云升; 周鹏; 代志家;
- 关键词:
项目摘要
Condition monitoring and fault diagnosis are crucial in guarantee of machinery safety operation. The machinery incipient fault signal is rather weak, which brings difficulties in signal detection and fault diagnosis. Stochastic-resonance (SR)-based filter has been adopted in machinery signal enhancement and fault diagnosis due to its distinct advantage characterized by the fact that the weak periodic signal can be enhanced by proper noise. However, the flexibility and accuracy of SR-based filter are insufficient, which reduces effectiveness and efficiency in weak signal detection and fault diagnosis. Focusing on these issues, this project intends to investigate a new adaptive complementary SR-based filter which utilizes the sound and the vibration signals simultaneously to adaptively enhance the weak signal for fault diagnosis. The project includes four parts as: 1) construct an adaptive SR-based filter, and investigate the weighted summation approach of the feedback criterions based on the artificial neural network; 2) construct an objective function based on the synthetic feedback criterion, and investigate the genetic-algorithm–based multi-parameters optimization method; 3) investigate a dual-channel complementary SR-based filter in which the vibration and the sound signals are fused to enhance each other at the data-layer; and 4) study the diagnosis conclusion fusion method based on the Dempster-Shafer evidence theory at the decision-layer. This project expects to provide theoretical method for high-accuracy, high-efficient machinery condition monitoring and incipient fault diagnosis.
状态监测和故障诊断对于保障机器设备运行安全至关重要。机械早期故障信号十分微弱,对微弱信号检测是个难点,也是实现精密诊断的关键点。随机共振滤波器能利用噪声增强微弱周期信号而被应用于机械故障诊断中,但其适应性、实时性和精准度不足导致难以实现高效检测诊断。针对此问题,本项目拟研究一种新的自适应互补随机共振滤波器,通过声音和振动信号的互补融合滤波,实现机械微弱信号的自适应增强和故障诊断。具体内容包括:1)建立自适应随机共振滤波器模型,利用人工神经网络研究多反馈指标的加权组合方法;2)构建基于自适应反馈综合指标的目标函数,研究滤波器多参数遗传算法优化方法;3)建立双通道信号互补随机共振滤波器模型,研究利用振动和声音信号在数据层互补融合信号增强检测方法;4)利用DS证据理论研究双通道信号诊断结论的决策层融合方法。通过项目研究期望为实现高精度高效率的机械状态监测与早期故障诊断提供新的理论支持。
结项摘要
微弱信号检测是实现高效高精度机械故障诊断的关键点和难点。随机共振是一种能够利用噪声增强微弱信号的非线性系统。本项目深入研究了基于随机共振的微弱信号检测方法以及相关机械故障诊断方法,取得了一系列的创新成果并超额完成了预期的研究任务。代表性的创新成果简介如下:1)提出一种多指标融合的自适应随机共振方法,能够更全面地评估随机共振滤波器的性能并完成系统多参数的自适应寻优,从而实现机械微弱信号的自适应增强和诊断。2)提出一种双通道互补随机共振架构,将传统单通道信号输入拓展为双通道互补输入,双输入信号能够利用相关噪声实现互补增强,从而提高了微弱信号检测的效果。3)提出一种微弱声音和振动信号互补融合的随机共振架构,利用同源声音和振动信号的互补信息协同增强提高机械故障诊断的精度。研究成果共发表SCI期刊论文21篇、EI期刊论文1篇,其中项目负责人一作/通信论文19篇,包括振动声音信号处理和机械故障诊断领域权威期刊《Journal of Sound and Vibration》6篇、《Mechanical Systems and Signal Processing》2篇;机电系统和仪器测试领域权威期刊《IEEE Trans. on Power Electronics》、《IEEE Trans. on Instrumentation and Measurement》、《IEEE Sensors Journal》共8篇、《Measurement Science and Technology》4篇;《振动与冲击》1篇;申请/授权发明专利5项。上述文章在谷歌学术中被引用350余次,在WOS中SCI他引230余次,最高单篇引用78次。
项目成果
期刊论文数量(22)
专著数量(0)
科研奖励数量(3)
会议论文数量(0)
专利数量(5)
Automatic bearing fault diagnosis of permanent magnet synchronous generator in wind turbine subjected to noise interference
噪声干扰下风电机组永磁同步发电机轴承故障自动诊断
- DOI:--
- 发表时间:2018
- 期刊:Measurement Science and Technology
- 影响因子:2.4
- 作者:Jun Guo;Siliang Lu;Chao Zhai;Qingbo He
- 通讯作者:Qingbo He
A Two-Step Strategy for Online Fault Detection of High-Resistance Connection in BLDC Motor
BLDC 电机高阻连接在线故障检测的两步策略
- DOI:10.1109/tpel.2019.2929102
- 发表时间:2020-03
- 期刊:IEEE Transactions on Power Electronics
- 影响因子:6.7
- 作者:Wang Hui;Lu Siliang;Qian Gang;Ding Jianming;Liu Yongbin;Wang Qunjing
- 通讯作者:Wang Qunjing
Enhanced feature extraction method for motor fault diagnosis using low-quality vibration data from wireless sensor networks
利用无线传感器网络低质量振动数据进行电机故障诊断的增强特征提取方法
- DOI:10.1088/1361-6501/ab5cca
- 发表时间:2020-01
- 期刊:Measurement Science and Technology
- 影响因子:2.4
- 作者:Qing Shu;Siliang Lu;Min Xia;Jianming Ding;Jiahao Niu;Yongbin Liu
- 通讯作者:Yongbin Liu
Feature fusion using kernel joint approximate diagonalization of eigen-matrices for rolling bearing fault identification
使用特征矩阵的核联合近似对角化的特征融合用于滚动轴承故障识别
- DOI:10.1016/j.jsv.2016.09.018
- 发表时间:2016-12-22
- 期刊:JOURNAL OF SOUND AND VIBRATION
- 影响因子:4.7
- 作者:Liu, Yongbin;He, Bing;Zhao, Yilei
- 通讯作者:Zhao, Yilei
A review of stochastic resonance in rotating machine fault detection
旋转机械故障检测中的随机共振综述
- DOI:10.1016/j.ymssp.2018.06.032
- 发表时间:2019-02-01
- 期刊:MECHANICAL SYSTEMS AND SIGNAL PROCESSING
- 影响因子:8.4
- 作者:Lu, Siliang;He, Qingbo;Wang, Jun
- 通讯作者:Wang, Jun
数据更新时间:{{ journalArticles.updateTime }}
{{
item.title }}
{{ item.translation_title }}
- DOI:{{ item.doi || "--"}}
- 发表时间:{{ item.publish_year || "--" }}
- 期刊:{{ item.journal_name }}
- 影响因子:{{ item.factor || "--"}}
- 作者:{{ item.authors }}
- 通讯作者:{{ item.author }}
数据更新时间:{{ journalArticles.updateTime }}
{{ item.title }}
- 作者:{{ item.authors }}
数据更新时间:{{ monograph.updateTime }}
{{ item.title }}
- 作者:{{ item.authors }}
数据更新时间:{{ sciAawards.updateTime }}
{{ item.title }}
- 作者:{{ item.authors }}
数据更新时间:{{ conferencePapers.updateTime }}
{{ item.title }}
- 作者:{{ item.authors }}
数据更新时间:{{ patent.updateTime }}
其他文献
多普勒畸变声学信号的伪时频分析及其校正
- DOI:--
- 发表时间:2016
- 期刊:振动与冲击
- 影响因子:--
- 作者:张海滨;陆思良;何清波;孔凡让
- 通讯作者:孔凡让
列车轴承轨边声信号冲击成分提取
- DOI:10.16385/j.cnki.issn.1004-4523.2020.04.023
- 发表时间:2020
- 期刊:振动工程学报
- 影响因子:--
- 作者:陈婧;刘方;付洋洋;刘永斌;陆思良;曹正
- 通讯作者:曹正
基于KJADE的列车轴承轨边声学诊断方法研究
- DOI:10.16450/j.cnki.issn.1004-6801.2020.04.022
- 发表时间:2020
- 期刊:振动、测试与诊断
- 影响因子:--
- 作者:龙磊;何兵;刘方;刘永斌;李桂华;陆思良
- 通讯作者:陆思良
其他文献
{{
item.title }}
{{ item.translation_title }}
- DOI:{{ item.doi || "--" }}
- 发表时间:{{ item.publish_year || "--"}}
- 期刊:{{ item.journal_name }}
- 影响因子:{{ item.factor || "--" }}
- 作者:{{ item.authors }}
- 通讯作者:{{ item.author }}
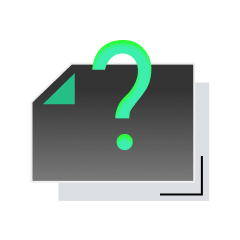
内容获取失败,请点击重试
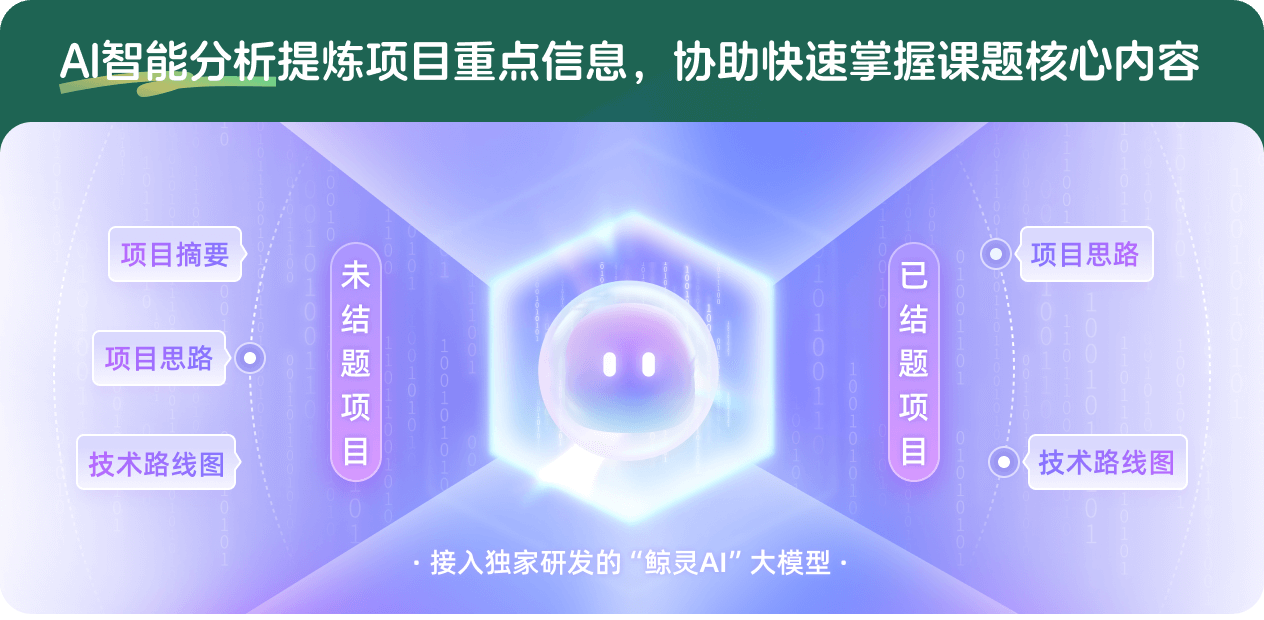
查看分析示例
此项目为已结题,我已根据课题信息分析并撰写以下内容,帮您拓宽课题思路:
AI项目摘要
AI项目思路
AI技术路线图
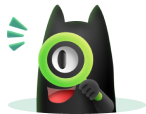
请为本次AI项目解读的内容对您的实用性打分
非常不实用
非常实用
1
2
3
4
5
6
7
8
9
10
您认为此功能如何分析更能满足您的需求,请填写您的反馈:
陆思良的其他基金
面向物联网在线监测的噪声强化全模拟电路物理神经网络研究
- 批准号:52375522
- 批准年份:2023
- 资助金额:50.00 万元
- 项目类别:面上项目
相似国自然基金
{{ item.name }}
- 批准号:{{ item.ratify_no }}
- 批准年份:{{ item.approval_year }}
- 资助金额:{{ item.support_num }}
- 项目类别:{{ item.project_type }}
相似海外基金
{{
item.name }}
{{ item.translate_name }}
- 批准号:{{ item.ratify_no }}
- 财政年份:{{ item.approval_year }}
- 资助金额:{{ item.support_num }}
- 项目类别:{{ item.project_type }}