基于混合模型驱动的电力物联系统数据质量优化方法
项目介绍
AI项目解读
基本信息
- 批准号:61803136
- 项目类别:青年科学基金项目
- 资助金额:24.0万
- 负责人:
- 依托单位:
- 学科分类:F0308.智能制造自动化系统理论与技术
- 结题年份:2021
- 批准年份:2018
- 项目状态:已结题
- 起止时间:2019-01-01 至2021-12-31
- 项目参与者:罗平; 郭金凤; 王淑娟; 何美光; 宁士远; 丁亮;
- 关键词:
项目摘要
The stability and reliability of industrial internet of things data play an important guiding role in the healthy and scientific operation of the new industrial chain. Currently, the potential physical model and the data-driven model used to address the problem are imperfect. Each of them encounters its limits in industrial application. The optimization method of the power industrial internet of things data based on a hybrid model is proposed in this project. Such as LSSVM, Brent’s method, Fuzzy Logic, Adaptive Kalman Filtering, RNN, and Broad Learning are considered to solve the problem. The main content is aiming to complete the quantitative performance of characterization theory and the physical model, improve the discrimination and recovery methods for the timing-exception in communication, and establish a neural network model merged with broad learning reducing the residuals. The effectiveness of this method will be confirmed by the comparison of the data derived from several different IOT systems, in the power industry scenario. Eventually, the results could help establish the data-quality standard of the IOT system, make the platform data displayed continuously and accurately, more importantly, make a contribution to the smart-grid and the reformation of electric power system.
保障物联系统数据质量的稳定可靠性,对新型产业链的健康、科学化运行具有重要指导作用。目前潜在解决该问题的物理模型与数据驱动模型皆有不完善之处,且单独采用信息系统物理模型或神经网络模型的方法都存在局限性。本项目提出并研究了基于混合模型驱动的电力物联系统数据质量优化方法。以最小二乘支持向量机、Brent’s整体优化算法、模糊逻辑、动态自适应Kalman滤波、循环神经网络、宽度学习等为解决问题的技术手段,进一步拓展完善物联系统性能定量表征理论与物理模型、提升通讯数据异常尤其是时序异常的判别及恢复方法,建立基于宽度学习改进的神经网络模型。并在电力工业场景下,通过多个不同来源物联系统展示数据的对比,论证该方法的有效性。最终建立物联系统数据质量评价标准,使平台数据展示连续、准确。研究成果对于推动国家智能电网信息化建设以及中央电力改革深入能源服务市场具有重要意义。
结项摘要
保障物联系统数据质量的稳定可靠性,对新型产业链的健康、科学化运行具有重要指导作用。本项目研究前潜在解决该问题的物理模型与数据驱动模型皆有不完善之处,且单独采用信息系统物理模型或神经网络模型的方法都存在局限性。本项目完成了基于混合模型驱动的电力物联系统数据质量优化方法。以无监督学习、支持向量机、模糊神经网络、自适应Kalman滤波、宽度学习等为解决问题的技术手段,进一步拓展并完善了物联系统性能定量表征理论与物理模型、提升通讯数据异常尤其是时序异常的判别及恢复方法;并在电力工业场景下,通过多个不同来源物联系统展示数据的对比,论证该方法的有效性。最终建立物联系统数据质量评价标准,使平台数据展示连续、准确。研究已经应用于有关电气综合系统平台,取得了发明专利等成果,并得到了政府的科技创业支持。
项目成果
期刊论文数量(11)
专著数量(0)
科研奖励数量(0)
会议论文数量(2)
专利数量(6)
Real-Time Charging Risk Assessment for Electric Vehicles Based on Improved Broad BP-AHP
基于改进广义BP-AHP的电动汽车实时充电风险评估
- DOI:10.1109/tie.2021.3111591
- 发表时间:2021-09
- 期刊:IEEE Transactions on Industrial Electronics
- 影响因子:7.7
- 作者:Quanbo Ge;Hanzhe Qiao;Chunxi Li;Qinmin Yang;Haoyu Jiang
- 通讯作者:Haoyu Jiang
Fault Diagnosis of Power IoT System Based on Improved Q-KPCA-RF Using Message Data
基于改进Q-KPCA-RF的基于消息数据的电力物联网系统故障诊断
- DOI:10.1109/jiot.2021.3058563
- 发表时间:2021-06
- 期刊:IEEE Internet of Things Journal
- 影响因子:10.6
- 作者:Haoyu Jiang;Kai Chen;Quanbo Ge;Yun Wang;Jinqiang Xu;Chunxi Li
- 通讯作者:Chunxi Li
Optimal economic dispatching of multi-microgrids by an improved genetic algorithm
改进遗传算法的多微电网优化经济调度
- DOI:10.1049/csy2.12008
- 发表时间:2021-03-01
- 期刊:IET CYBER-SYSTEMS AND ROBOTICS
- 影响因子:--
- 作者:Jiang, Haoyu;Ning, Shiyuan;Bin, Yu
- 通讯作者:Bin, Yu
Wear Fault Diagnosis of Aeroengines Based on Broad Learning System and Ensemble Learning
基于广义学习系统和集成学习的航空发动机磨损故障诊断
- DOI:10.3390/en12244750
- 发表时间:2019-12
- 期刊:Energies
- 影响因子:3.2
- 作者:Mengmeng Wang;Quanbo Ge;Haoyu Jiang;Gang Yao
- 通讯作者:Gang Yao
Maximum Power Demand Prediction Using Fbprophet With Adaptive Kalman Filtering
使用 Fbprophet 和自适应卡尔曼滤波进行最大功率需求预测
- DOI:10.1109/access.2020.2968101
- 发表时间:2020-01-01
- 期刊:IEEE ACCESS
- 影响因子:3.9
- 作者:Guo, Chen;Ge, Quanbo;Hua, Qiang
- 通讯作者:Hua, Qiang
数据更新时间:{{ journalArticles.updateTime }}
{{
item.title }}
{{ item.translation_title }}
- DOI:{{ item.doi || "--"}}
- 发表时间:{{ item.publish_year || "--" }}
- 期刊:{{ item.journal_name }}
- 影响因子:{{ item.factor || "--"}}
- 作者:{{ item.authors }}
- 通讯作者:{{ item.author }}
数据更新时间:{{ journalArticles.updateTime }}
{{ item.title }}
- 作者:{{ item.authors }}
数据更新时间:{{ monograph.updateTime }}
{{ item.title }}
- 作者:{{ item.authors }}
数据更新时间:{{ sciAawards.updateTime }}
{{ item.title }}
- 作者:{{ item.authors }}
数据更新时间:{{ conferencePapers.updateTime }}
{{ item.title }}
- 作者:{{ item.authors }}
数据更新时间:{{ patent.updateTime }}
其他文献
一维全场彩虹喷雾测量方法试验研究
- DOI:--
- 发表时间:2017
- 期刊:光学技术
- 影响因子:--
- 作者:姜淏予;操凯霖;李灿;吴学成
- 通讯作者:吴学成
彩虹自标定方法对混合喷雾比例的原位测量
- DOI:--
- 发表时间:2017
- 期刊:光学技术
- 影响因子:--
- 作者:操凯霖;姜淏予;李灿;吴学成
- 通讯作者:吴学成
全场彩虹技术测量喷雾浓度及粒径分布
- DOI:--
- 发表时间:2013
- 期刊:Acta Physica Sinica
- 影响因子:1
- 作者:姜淏予;洪巧巧;Gérard Gréhan;岑可法
- 通讯作者:岑可法
其他文献
{{
item.title }}
{{ item.translation_title }}
- DOI:{{ item.doi || "--" }}
- 发表时间:{{ item.publish_year || "--"}}
- 期刊:{{ item.journal_name }}
- 影响因子:{{ item.factor || "--" }}
- 作者:{{ item.authors }}
- 通讯作者:{{ item.author }}
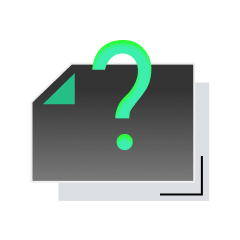
内容获取失败,请点击重试
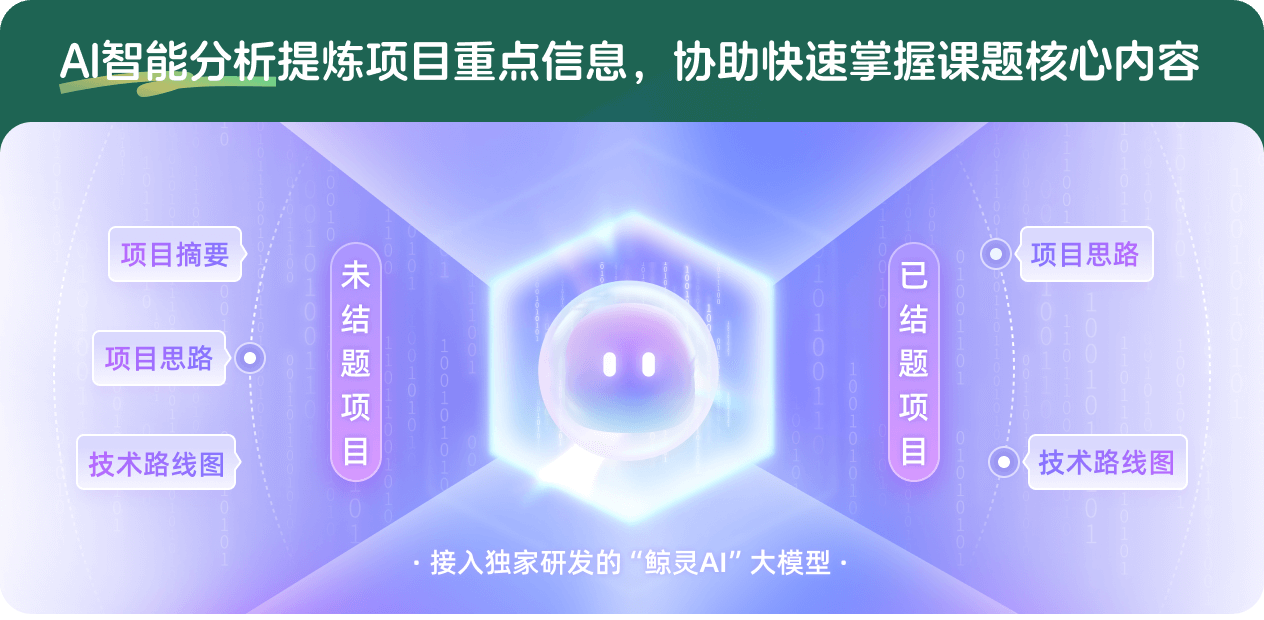
查看分析示例
此项目为已结题,我已根据课题信息分析并撰写以下内容,帮您拓宽课题思路:
AI项目摘要
AI项目思路
AI技术路线图
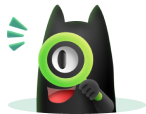
请为本次AI项目解读的内容对您的实用性打分
非常不实用
非常实用
1
2
3
4
5
6
7
8
9
10
您认为此功能如何分析更能满足您的需求,请填写您的反馈:
相似国自然基金
{{ item.name }}
- 批准号:{{ item.ratify_no }}
- 批准年份:{{ item.approval_year }}
- 资助金额:{{ item.support_num }}
- 项目类别:{{ item.project_type }}
相似海外基金
{{
item.name }}
{{ item.translate_name }}
- 批准号:{{ item.ratify_no }}
- 财政年份:{{ item.approval_year }}
- 资助金额:{{ item.support_num }}
- 项目类别:{{ item.project_type }}