大脑中神经元网络结构与动力学的研究
项目介绍
AI项目解读
基本信息
- 批准号:11901388
- 项目类别:青年科学基金项目
- 资助金额:21.0万
- 负责人:
- 依托单位:
- 学科分类:A0604.生物与生命科学中的数学
- 结题年份:2022
- 批准年份:2019
- 项目状态:已结题
- 起止时间:2020-01-01 至2022-12-31
- 项目参与者:--
- 关键词:
项目摘要
The neuronal networks in the brain are endowed with complex and delicate connectivity structure, which supports efficient information processing and rich emotional and cognitive functions. The direct measurement of the connectivity structure in the brain is experimentally challenging at the current stage, impeding the deep understanding of the brain structure. In contrast, with the fast development of calcium imaging and electrophysiological recording techniques, it is now feasible to simultaneously measure the firing activity of thousands of neurons in a network. Based on this, as a first step of this project, we aim to develop information-theoretic based statistical methods to recover the connectivity structure of a neuronal network using the time series data of neuronal dynamical activities. Importantly, our developed method will be validated via a close collaboration with experimental labs. Furthermore, by taking into account the fact that the function of the brain is in general encoded and represented by the dynamics of neuronal activities, by using math tools including PDE and asymptotic analysis, numerical computation, nonlinear network dynamics analysis, and non-equilibrium statistical physics, we next aim to develop the kinetic theory of neuronal network dynamics based on the heterogeneous features of real neuronal connectivity, describing analytically the general relation between network connectivity and the corresponding neuronal population dynamics. Our work will provide a potential mathematical framework to understand the relation between brain structure and its functions.
大脑中神经元网络的复杂结构赋予了我们高效的信息处理能力以及丰富的情感与认知功能。然而神经元网络的结构特点以及结构与功能之间的关系至今仍尚未完全明确。目前,在实验中对神经元之间物理连接的直接测量较为困难,阻碍了人们对大脑结构特性的深入认识。另一方面,我们可以相对容易地同时记录大量神经元群体的电活动信号。因此,在本项研究中,申请人首先计划发展基于信息论的统计推断方法,并同神经科学实验室紧密合作,尝试从神经元动力学时间序列数据中反向推断神经元网络的底层连接结构。进一步,考虑到大脑的功能通常由神经元网络动力学所编码和表征,申请人计划利用偏微分方程和渐近分析、数值计算、非线性网络动力学、以及非平衡态统计物理等工具,建立基于真实神经元网络各向异性连接结构的网络动理学理论,解析刻画网络结构与神经元群体动力学之间的定量关系,为理解大脑的结构与功能关系提供潜在的理论框架。
结项摘要
该项目致力于应用微分方程、渐近分析、数值计算和网络动力学分析等应用数学方法研究大脑结构特点及其与动力学之间的定量关系。在项目执行期间,取得了一系列重要的研究成果,主要包括(1)建立了分析单神经元结构与动力学关系的数学分析框架,并应用于分析神经元树突信号整合的动力学,提出了树突整合的定量法则与机制;(2)提出了刻画神经元网络异质性结构特点的最大熵原理,并通过分析多个物种的大脑网络结构数据,验证了最大熵原理的普适性;(3)发展了研究神经元网络结构与动力学关系的渐近分析方法,并揭示了网络动力学中时间尺度层级化现象背后的机制;(4)建立了格兰杰因果等常用的统计推断方法之间的渐近等价性,并通过数值计算模拟验证它们在重构神经元网络连接结构上的有效性。这些研究成果阐明了一些重要的大脑网络结构与动力学特点背后的机制,并可以为我们之后深入理解大脑的结构与动力学关系提供有效的理论分析框架和统计推断工具。
项目成果
期刊论文数量(6)
专著数量(0)
科研奖励数量(1)
会议论文数量(0)
专利数量(0)
Hierarchical timescales in the neocortex: Mathematical mechanism and biological insights.
新皮质的层次时间尺度:数学机制和生物学见解
- DOI:10.1073/pnas.2110274119
- 发表时间:2022-02-08
- 期刊:Proceedings of the National Academy of Sciences of the United States of America
- 影响因子:11.1
- 作者:Li S;Wang XJ
- 通讯作者:Wang XJ
Mathematical Modeling and Analysis of Spatial Neuron Dynamics: Dendritic Integration and Beyond
空间神经元动力学的数学建模和分析:树突整合及其他
- DOI:10.1002/cpa.22020
- 发表时间:2021-08-24
- 期刊:COMMUNICATIONS ON PURE AND APPLIED MATHEMATICS
- 影响因子:3
- 作者:Li,Songting;McLaughlin,David W.;Zhou,Douglas
- 通讯作者:Zhou,Douglas
Emergence of spatially periodic diffusive waves in small-world neuronal networks
小世界神经元网络中空间周期性扩散波的出现
- DOI:10.1103/physreve.100.042401
- 发表时间:2019
- 期刊:Phys. Rev. E
- 影响因子:--
- 作者:Gu Qinglong;Xiao Yanyang;Li Songting;Zhou Douglas
- 通讯作者:Zhou Douglas
Fast algorithms for simulation of neuronal dynamics based on the bilinear dendritic integration rule
基于双线性树突积分规则的神经元动力学模拟快速算法
- DOI:10.4310/cms.2019.v17.n5.a7
- 发表时间:2019
- 期刊:Communications in Mathematical Sciences
- 影响因子:1
- 作者:Dai Wei P.;Li Songting;Zhou Douglas
- 通讯作者:Zhou Douglas
数据更新时间:{{ journalArticles.updateTime }}
{{
item.title }}
{{ item.translation_title }}
- DOI:{{ item.doi || "--"}}
- 发表时间:{{ item.publish_year || "--" }}
- 期刊:{{ item.journal_name }}
- 影响因子:{{ item.factor || "--"}}
- 作者:{{ item.authors }}
- 通讯作者:{{ item.author }}
数据更新时间:{{ journalArticles.updateTime }}
{{ item.title }}
- 作者:{{ item.authors }}
数据更新时间:{{ monograph.updateTime }}
{{ item.title }}
- 作者:{{ item.authors }}
数据更新时间:{{ sciAawards.updateTime }}
{{ item.title }}
- 作者:{{ item.authors }}
数据更新时间:{{ conferencePapers.updateTime }}
{{ item.title }}
- 作者:{{ item.authors }}
数据更新时间:{{ patent.updateTime }}
其他文献
其他文献
{{
item.title }}
{{ item.translation_title }}
- DOI:{{ item.doi || "--" }}
- 发表时间:{{ item.publish_year || "--"}}
- 期刊:{{ item.journal_name }}
- 影响因子:{{ item.factor || "--" }}
- 作者:{{ item.authors }}
- 通讯作者:{{ item.author }}
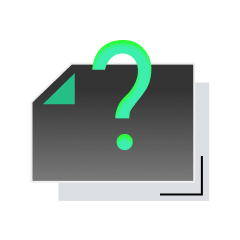
内容获取失败,请点击重试
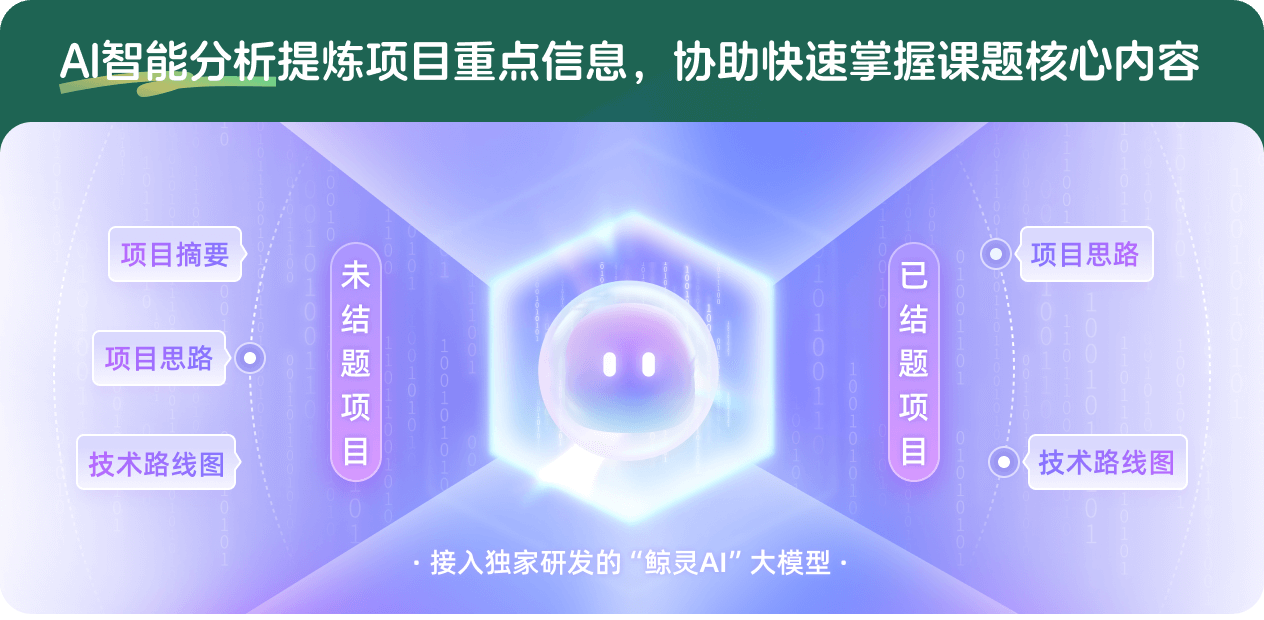
查看分析示例
此项目为已结题,我已根据课题信息分析并撰写以下内容,帮您拓宽课题思路:
AI项目摘要
AI项目思路
AI技术路线图
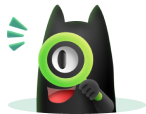
请为本次AI项目解读的内容对您的实用性打分
非常不实用
非常实用
1
2
3
4
5
6
7
8
9
10
您认为此功能如何分析更能满足您的需求,请填写您的反馈:
李松挺的其他基金
大脑复杂网络的结构推断与动力学分析
- 批准号:12271361
- 批准年份:2022
- 资助金额:46 万元
- 项目类别:面上项目
相似国自然基金
{{ item.name }}
- 批准号:{{ item.ratify_no }}
- 批准年份:{{ item.approval_year }}
- 资助金额:{{ item.support_num }}
- 项目类别:{{ item.project_type }}
相似海外基金
{{
item.name }}
{{ item.translate_name }}
- 批准号:{{ item.ratify_no }}
- 财政年份:{{ item.approval_year }}
- 资助金额:{{ item.support_num }}
- 项目类别:{{ item.project_type }}