基于仿生学原理的恶意代码判定与防护模型研究
项目介绍
AI项目解读
基本信息
- 批准号:61472447
- 项目类别:面上项目
- 资助金额:80.0万
- 负责人:
- 依托单位:
- 学科分类:F0205.网络与系统安全
- 结题年份:2018
- 批准年份:2014
- 项目状态:已结题
- 起止时间:2015-01-01 至2018-12-31
- 项目参与者:单征; 韩林; 崔平非; 戴超; 岳峰; 李男; 金久真; 张啸川; 白虹;
- 关键词:
项目摘要
With the continuous deep-going of China's informationalization , information security is increasingly in grim situation. One of the main threats faced by information security is malicious code. And its determination and defense approach has been the focus of various security research teams. However, existing methods have several weakpoints, such as the difficulty to effectively identify emerging malicious code, security professionals require manual intervention , the threat of malicious code is not timely responded etc. This topic learns bionics in cognitive theory, immune theory and autonomic nervous theory to explore the application of principles of bionics in the field of malicious code determination and defense applications. The main research topics consist three aspects: First, the determination of malicious code based on cognitive theory. With excavation code behavior in depth to determine the nature of emerging malicious code accurately. Second, the optimal matching of defense strategy based on the theory of immunity to make the the choice of defense strategy effectively against malicious code without human intervention. Third, malicious code determination and defense model based on the autonomic nervous. With the applications of adaptive strategies the model could respond to malicious code threats timely. By studying this topic , we could improve the intelligence, automation, integration degree of malicious code determination and defense, and guarantee the accuracy of determining and the validity and timeliness of malicious code defense.
随着我国信息化建设的持续深入,信息安全形势日益严峻,恶意代码作为信息安全面临的主要威胁之一,其判定与防御方法一直是各安全团队的研究重点。但是现有方法存在对于新兴恶意代码难以有效识别,需要安全专业人员的人工介入,对恶意代码威胁响应不及时等问题。本课题借鉴仿生学原理中的认知理论、免疫理论以及自主神经理论,探索仿生学原理在恶意代码判定与防御领域的应用,课题主要研究三方面的问题:一是基于认知理论的恶意代码判定,深度挖掘代码行为,准确判定新兴代码恶意性;二是基于免疫理论的恶意代码防御策略最优匹配,在无需人工介入的情况下选择能够有效对抗恶意代码的防御策略;三是基于自主神经系统的恶意代码判定与防御模型,对防御策略加以自适应应用,及时响应恶意代码威胁。通过本课题的研究,能够提高恶意代码判定与防御的智能化、自动化、一体化程度,进而保证恶意代码判定的准确性,防御的有效性、及时性。
结项摘要
本课题基于仿生学原理,重点围绕恶意代码的检测、防御、相似性分析和基准测试集构建展开研究。本课题提出了软件基因理论,并探索其在恶意代码检测判定及漏洞挖掘方面的应用。针对现有公开的数据集往往无法匹配恶意代码的发展速度,且部分数据集的采集覆盖率较低的问题,本课题提出了一种基于生物遗传算法的自动化的恶意代码基准测试集生成框架,实现了从大规模的原始数据集到具有代表性的基准测试集的生成。本课题研发了一套面向恶意代码分析的二进制软件逆向系统,并提出多种恶意代码检测识别算法,用于检测恶意代码心跳行为、识别定位恶意代码的汇编级行为,实现了恶意代码的分类和威胁性评估和沙箱规避行为的检测。本课题首次提出与平台无关的归约指令依赖关系图,并将其运用于程序相似性度量,实现了跨平台通用的程序相似性度量,不仅能应对主流的代码混淆算法,同时能对程序的内部进行相似性度量。本课题研究借鉴生物界的拟态现象,借助软件多样化编译实现系统异构性,降低网络空间中软件漏洞和后门的威胁。
项目成果
期刊论文数量(32)
专著数量(2)
科研奖励数量(0)
会议论文数量(11)
专利数量(3)
针对指令重叠混淆技术的恶意代码反汇编研究
- DOI:--
- 发表时间:2016
- 期刊:小型微型计算机系统
- 影响因子:--
- 作者:戴超;庞建民;梁光辉;白虹;张啸川;陈茜月
- 通讯作者:陈茜月
基于小波变换的木马心跳行为检测方法
- DOI:--
- 发表时间:2016
- 期刊:计算机科学
- 影响因子:--
- 作者:白虹;庞建民;戴超;岳峰
- 通讯作者:岳峰
Automation Benchmark Generation Framework for Malware Detection
恶意软件检测自动化基准生成框架
- DOI:--
- 发表时间:2018
- 期刊:Security and Communication Networks
- 影响因子:--
- 作者:Guanghui Liang;Jianmin Pang;Zheng Shan;Runqing Yang;Yihang Chen
- 通讯作者:Yihang Chen
一种基于主动认知决策的高效能模型
- DOI:--
- 发表时间:2015
- 期刊:计算机科学
- 影响因子:--
- 作者:杨劲;庞建民;王俊超;于锦涛;刘睿
- 通讯作者:刘睿
一种二进制翻译中间表示正确性的测试方法
- DOI:--
- 发表时间:--
- 期刊:信息工程大学学报
- 影响因子:--
- 作者:傅立国;庞建民;岳峰
- 通讯作者:岳峰
数据更新时间:{{ journalArticles.updateTime }}
{{
item.title }}
{{ item.translation_title }}
- DOI:{{ item.doi || "--"}}
- 发表时间:{{ item.publish_year || "--" }}
- 期刊:{{ item.journal_name }}
- 影响因子:{{ item.factor || "--"}}
- 作者:{{ item.authors }}
- 通讯作者:{{ item.author }}
数据更新时间:{{ journalArticles.updateTime }}
{{ item.title }}
- 作者:{{ item.authors }}
数据更新时间:{{ monograph.updateTime }}
{{ item.title }}
- 作者:{{ item.authors }}
数据更新时间:{{ sciAawards.updateTime }}
{{ item.title }}
- 作者:{{ item.authors }}
数据更新时间:{{ conferencePapers.updateTime }}
{{ item.title }}
- 作者:{{ item.authors }}
数据更新时间:{{ patent.updateTime }}
其他文献
二进制翻译中动静结合的寄存器分配优化方法
- DOI:--
- 发表时间:2019
- 期刊:计算机研究与发展
- 影响因子:--
- 作者:王军;庞建民;傅立国;岳峰;单征;张家豪
- 通讯作者:张家豪
多面体模型中分裂分块算法的设计与实现
- DOI:--
- 发表时间:2020
- 期刊:计算机学报
- 影响因子:--
- 作者:李颖颖;赵捷;庞建民
- 通讯作者:庞建民
一种基于混合学习的恶意代码检测方法
- DOI:--
- 发表时间:2021
- 期刊:电子学报
- 影响因子:--
- 作者:梁光辉;摆亮;庞建民;单征;岳峰;张磊
- 通讯作者:张磊
其他文献
{{
item.title }}
{{ item.translation_title }}
- DOI:{{ item.doi || "--" }}
- 发表时间:{{ item.publish_year || "--"}}
- 期刊:{{ item.journal_name }}
- 影响因子:{{ item.factor || "--" }}
- 作者:{{ item.authors }}
- 通讯作者:{{ item.author }}
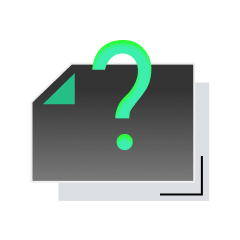
内容获取失败,请点击重试
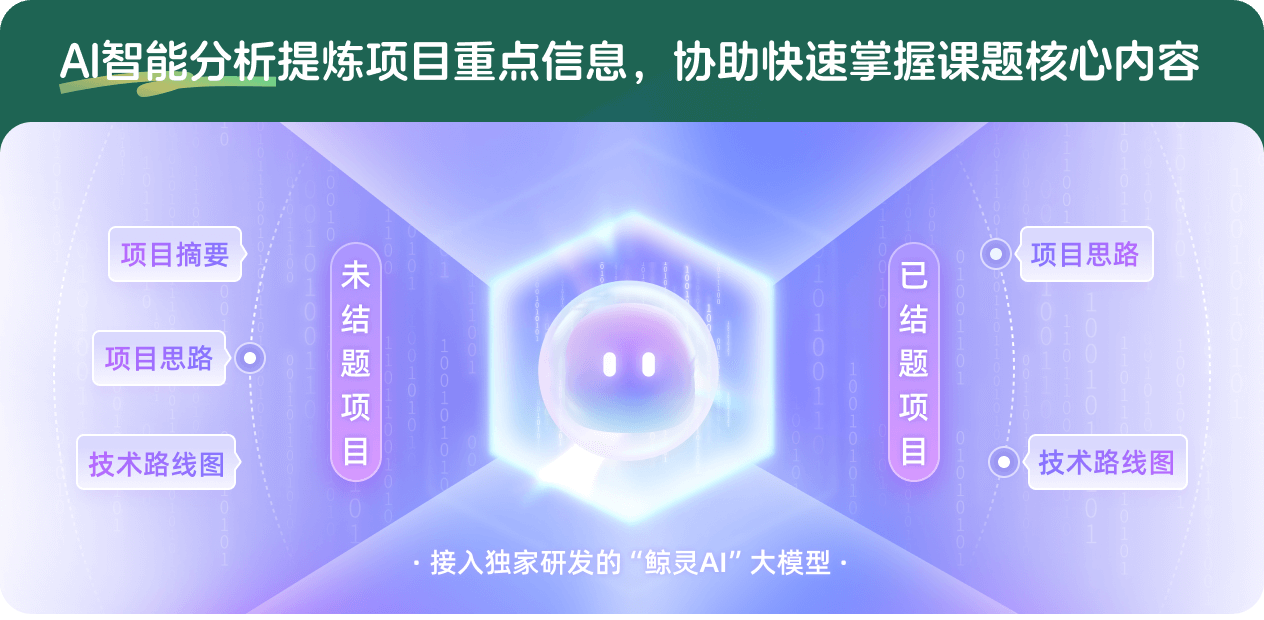
查看分析示例
此项目为已结题,我已根据课题信息分析并撰写以下内容,帮您拓宽课题思路:
AI项目摘要
AI项目思路
AI技术路线图
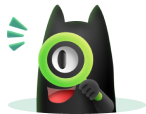
请为本次AI项目解读的内容对您的实用性打分
非常不实用
非常实用
1
2
3
4
5
6
7
8
9
10
您认为此功能如何分析更能满足您的需求,请填写您的反馈:
相似国自然基金
{{ item.name }}
- 批准号:{{ item.ratify_no }}
- 批准年份:{{ item.approval_year }}
- 资助金额:{{ item.support_num }}
- 项目类别:{{ item.project_type }}
相似海外基金
{{
item.name }}
{{ item.translate_name }}
- 批准号:{{ item.ratify_no }}
- 财政年份:{{ item.approval_year }}
- 资助金额:{{ item.support_num }}
- 项目类别:{{ item.project_type }}