高光谱影像混合像元的团簇平均场光谱分解新方法
项目介绍
AI项目解读
基本信息
- 批准号:61801018
- 项目类别:青年科学基金项目
- 资助金额:25.0万
- 负责人:
- 依托单位:
- 学科分类:F0113.信息获取与处理
- 结题年份:2021
- 批准年份:2018
- 项目状态:已结题
- 起止时间:2019-01-01 至2021-12-31
- 项目参与者:邹金霖; 王陆旸; 邵扬; 张胜; 于磊; 牛秉青;
- 关键词:
项目摘要
Hyperspectral image information processing is a hot topic in the field of remote sensing applications and it can be applied widely in civil and military fields. However, the mixed pixel resulting from spatial resolution and ground object features restricts the quantitative application of hyperspectral remote sensing image severely. In order to accurately depict the real properties of mixed pixel, a new spectral unmixing method using cluster dynamical mean field is proposed to solve the problem of large decomposition error of linear mixed models. A mathematical description model of hyperspectral mixture system meeting the self-consistency is proposed, this models can accurately express the nonlinear spectral mixture model of mixed pixel, break through the limitations of spatial resolution of hyperspectral sensor, and achieve micro scale description between different substances spectrum. This project proposes a new method which can map hyperspectral mixture system description model into effective impurity model, designes impurity solvers for the mean field of a spectral cluster, builds a new model for fast and accurate spectral decomposition of mixed pixel, calculates spectral abundance of mixed pixel, and achieves mixed pixel spectral unmixing finally. The validity of the algorithm will be verified by using the satellite image and the data obtained from the ground in this project. This method can satisfy practical and provide the new insights and theoretical basis for nonlinear.spectral mixture.
高光谱影像信息处理是遥感应用领域的一个研究热点,在民用和军用领域都具有广泛的应用前景。但是空间分辨率和地物多样性导致的混合像元严重制约了高光谱卫星遥感影像定量化应用发展。为了在亚像元精度内精确刻画出混合像元的真实属性,本项目针对线性混合模型分解误差大的问题,在构建混合像元成像机理模型的基础上,研究团簇平均场光谱分解新方法。提出一种满足自洽性的高光谱关联混合系统数学描述模型,能够准确表述混合像元非线性光谱混合模式,突破高光谱传感器空间分辨率的限制,实现不同物质光谱间微观尺度描述;提出有效杂质模型映射的新方法,设计面向光谱团簇平均场的精确对角化杂质求解器,建立混合像元快速准确光谱分解新模型,计算混合像元光谱丰度,实现混合像元光谱分解。本项目利用卫星影像和地面获取数据验证算法模型的有效性,能够满足实际应用需求,为非线性光谱分解技术提供新思路和理论依据。
结项摘要
混合像元分解是高光谱遥感数据处理的关键问题之一,在目标探测、识别与分类中具有广泛的应用前景。本项目针对高光谱影像线性光谱分解精度低的问题,基于团簇平均场理论研究了混合像元成像机理,并结合深度学习框架提出了系列非线性光谱解混模型,实现了高光谱混合像元分解结果均方根误差小于0.1,光谱角距离低于0.1的技术指标,能够满足实际应用需求。.本项目主要研究内容包括:(1)在混合成像机理分析基础上,构建了非线性光谱关联系统描述模型,为光谱分解模型设计提供理论依据;(2)提出了量子效应机制的光谱聚类模型,建立团簇平均场分解数学描述,构建自洽约束条件,准确获取光谱丰度信息,实现混合像元的光谱分解;(3)提出了散射变换框架下的高光谱混合像元分解方法,利用多层深度特征提取的结构,提高了噪声干扰条件下的丰度估计精度;(4)提出了散射变换和注意力机制相结合的深度网络模型,增强相关特征,并消除深度学习网络结构深度增加带来的模型梯度消失问题,实现了小样本条件下的高光谱影像混合像元分解;(5)利用高光谱遥感影像,完成对所提算法模型的评估和验证。.本项目的研究成果能够为非线性混合像元分解技术提供新思路,对于高光谱数据定量化应用精度的提升、目标探测能力的提高以及地物目标分类的精细化提供理论依据和技术支持,具有重要的现实意义。
项目成果
期刊论文数量(5)
专著数量(0)
科研奖励数量(0)
会议论文数量(0)
专利数量(2)
Attention-Based Residual Network with Scattering Transform Features for Hyperspectral Unmixing with Limited Training Samples
具有散射变换特征的基于注意力的残差网络,用于有限训练样本的高光谱分解
- DOI:10.3390/rs12030400
- 发表时间:2020-01
- 期刊:Remote. Sens.
- 影响因子:--
- 作者:Yiliang Zeng;Christian Ritz;Jiahong Zhao;Jinhui Lan
- 通讯作者:Jinhui Lan
Scattering Transform Framework for Unmixing of Hyperspectral Data
用于分解高光谱数据的散射变换框架
- DOI:10.3390/rs11232868
- 发表时间:2019-12
- 期刊:Remote. Sens.
- 影响因子:--
- 作者:Yiliang Zeng;Christian Ritz;Jiahong Zhao;Jinhui Lan
- 通讯作者:Jinhui Lan
JRL-YOLO: A Novel Jump-Join Repetitious Learning Structure for Real-Time Dangerous Object Detection.
JRL-YOLO:一种用于实时危险物体检测的新颖的跳跃连接重复学习结构
- DOI:10.1155/2021/5536152
- 发表时间:2021
- 期刊:Computational intelligence and neuroscience
- 影响因子:--
- 作者:Zeng Y;Zhang L;Zhao J;Lan J;Li B
- 通讯作者:Li B
Sub-pixel spectral clustering model of quantum mechanism effect for hyperspectral images
高光谱图像量子机制效应的亚像素光谱聚类模型
- DOI:10.1007/s12517-020-5147-9
- 发表时间:2020-01
- 期刊:Arabian Journal of Geosciences
- 影响因子:--
- 作者:Yiliang Zeng;Jinhui Lan;Kangli Liu
- 通讯作者:Kangli Liu
数据更新时间:{{ journalArticles.updateTime }}
{{
item.title }}
{{ item.translation_title }}
- DOI:{{ item.doi || "--"}}
- 发表时间:{{ item.publish_year || "--" }}
- 期刊:{{ item.journal_name }}
- 影响因子:{{ item.factor || "--"}}
- 作者:{{ item.authors }}
- 通讯作者:{{ item.author }}
数据更新时间:{{ journalArticles.updateTime }}
{{ item.title }}
- 作者:{{ item.authors }}
数据更新时间:{{ monograph.updateTime }}
{{ item.title }}
- 作者:{{ item.authors }}
数据更新时间:{{ sciAawards.updateTime }}
{{ item.title }}
- 作者:{{ item.authors }}
数据更新时间:{{ conferencePapers.updateTime }}
{{ item.title }}
- 作者:{{ item.authors }}
数据更新时间:{{ patent.updateTime }}
其他文献
Distance estimation using a panoramic sensor based on a novel spherical optical flow algorithm
使用基于新型球形光流算法的全景传感器进行距离估计
- DOI:10.1016/j.optlastec.2012.07.009
- 发表时间:2013-02
- 期刊:Optics and Laser Technology
- 影响因子:5
- 作者:蓝金辉;李建;胡广大;曾溢良
- 通讯作者:曾溢良
Imitate geometric manifold coverage method for one-class classification of remote sensing data
遥感数据一类分类的仿几何流形覆盖法
- DOI:10.1007/s12517-014-1275-4
- 发表时间:2015-02
- 期刊:Arabian Journal of Geosciences
- 影响因子:--
- 作者:曾溢良;蓝金辉
- 通讯作者:蓝金辉
其他文献
{{
item.title }}
{{ item.translation_title }}
- DOI:{{ item.doi || "--" }}
- 发表时间:{{ item.publish_year || "--"}}
- 期刊:{{ item.journal_name }}
- 影响因子:{{ item.factor || "--" }}
- 作者:{{ item.authors }}
- 通讯作者:{{ item.author }}
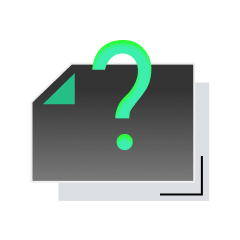
内容获取失败,请点击重试
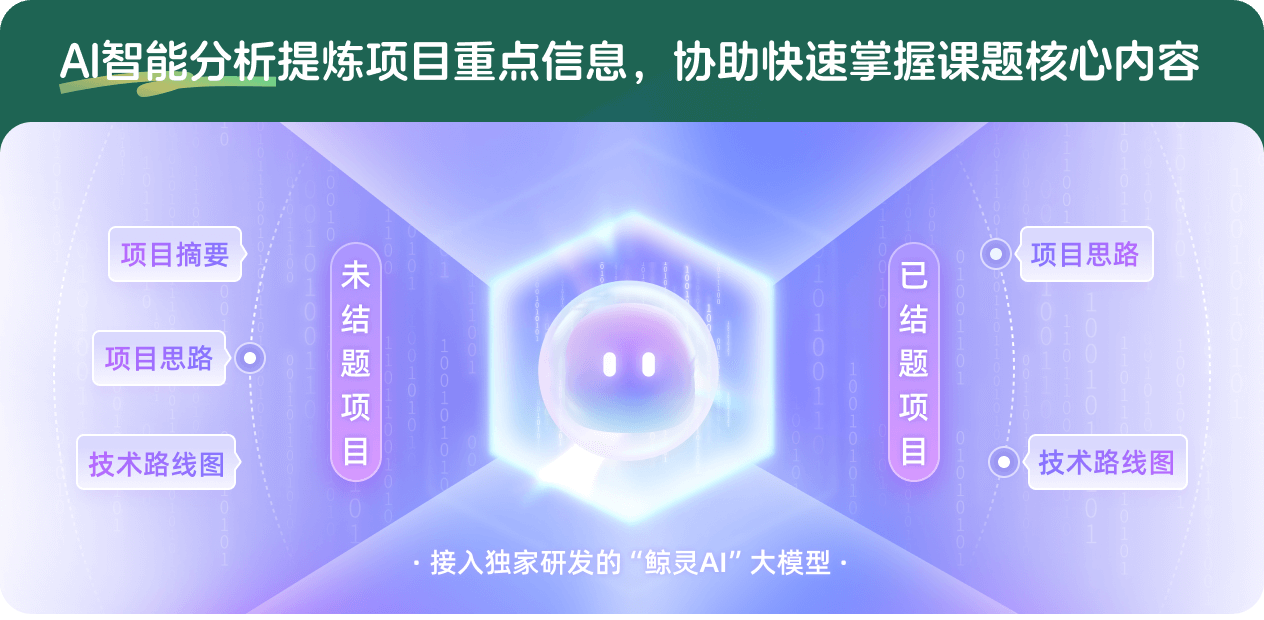
查看分析示例
此项目为已结题,我已根据课题信息分析并撰写以下内容,帮您拓宽课题思路:
AI项目摘要
AI项目思路
AI技术路线图
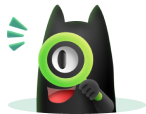
请为本次AI项目解读的内容对您的实用性打分
非常不实用
非常实用
1
2
3
4
5
6
7
8
9
10
您认为此功能如何分析更能满足您的需求,请填写您的反馈:
相似国自然基金
{{ item.name }}
- 批准号:{{ item.ratify_no }}
- 批准年份:{{ item.approval_year }}
- 资助金额:{{ item.support_num }}
- 项目类别:{{ item.project_type }}
相似海外基金
{{
item.name }}
{{ item.translate_name }}
- 批准号:{{ item.ratify_no }}
- 财政年份:{{ item.approval_year }}
- 资助金额:{{ item.support_num }}
- 项目类别:{{ item.project_type }}