基于生物信息学和自然语言处理的水稻抗病基因挖掘
项目介绍
AI项目解读
基本信息
- 批准号:61202305
- 项目类别:青年科学基金项目
- 资助金额:22.0万
- 负责人:
- 依托单位:
- 学科分类:F0213.生物信息计算与数字健康
- 结题年份:2015
- 批准年份:2012
- 项目状态:已结题
- 起止时间:2013-01-01 至2015-12-31
- 项目参与者:牛晓辉; 袁道军; 张四兰; 熊慧娟; 常继伟; 邢锋;
- 关键词:
项目摘要
Along with the completion of rice genome sequencing and beginning of gene annotation era, the research on rice resistance gene/ protein has accumulated a large number of bioinformatics data and biological literature data. Unfortunately, the current exploration of the functional genes of rice disease resistance still lags behind. Based on the research basis of our previous work in functional gene discovery and bioinformatics algorithm study, and by using both bioinformatics methods based on sequence, structure, gene expression analysis and natural language process methods based on semantics, the gene discovery system for Xanthomonas oryzae pv.oryzae resistant gene and Magnaporthe grisea resistant gene in rice is constructed. First, we use the microarray method and text mining method to build a filtered candidate gene dataset, then we use sequence structure information, gene ontology information, documentation semantics of entry information and biomedical event extraction information and build through the support vector machine four categories of predictors, and finally we use artificial neural network to establish a comprehensive evaluation of the multiple classifiers system and construct credible rice disease resistance gene discovery system through cross-validation. Methods used in this research combine text mining-based natural language processing with the traditional ab-initio method based on sequence information, which may shorten the screening process of the rice disease resistance gene, and the credibility of the data and results are high if compared with the mere traditional bioinformatics method.
伴随着水稻基因测序的完成和基因注释时代的来临,水稻抗病基因/蛋白质积累了大量的生物信息学数据和生物文献数据,而水稻抗病功能基因的发掘当前仍显滞后。课题组在功能基因挖掘和生物信息学算法的已有研究基础上,将基于序列、结构和表达差异分析的生物信息学方法和基于语义学的生物自然语言处理方法相结合,对水稻白叶枯病、抗稻瘟病等抗病基因进行发掘。首先利用基因表达差异的微阵列方法和病原物基因共显的语义学方法构建初筛候选抗病基因数据集,再提取序列结构信息、基因本体论信息、文献语义词条信息和生物事件提取信息,通过支撑向量机构建四个分类预测器,最后利用神经网络建立多分类器的综合评判系统,经过系统自检和完善得到可信的水稻抗病基因发掘系统。在传统基于序列结构的从头预测方法基础上,本课题有效结合基于文本挖掘的自然语言处理方法,能缩短水稻抗病基因的筛选过程,其数据和结果的可信度将较传统生物信息学方法有所提高。
结项摘要
该课题的主要研究思路是将生物自然语言处理(BioNLP)作为系统生物学的一环,看做一种有利的补充手段,取得足够可信的科学证据,协助传统的基于序列结构的传统生物信息学方法,去挖掘水稻基因数据,并可对其他有意义的生物医药类知识挖掘提供支持。..研究项目已经按照计划顺利进行。在实施该项目过程中,作者访问了香港城市大学语言学系与Alex Chengyu Fang教授展开BioNLP合作,访问德州大学阿灵顿分校与Heng Huang教授展开数据挖掘的合作,拜访科罗拉多大学丹佛分校与Kevin B Cohen展开BioNLP合作。在项目支持下发表论文9篇,其中6篇SCI,另外3篇也均为EI收录,项目取得了预计目标。..我们与香港城市大学的Alex Chengyu Fang教授合作,对芬兰Turku大学做出的TEES生物文本事件提取系统做出了优化,优化后的系统相较之前的24个BioNLP ST参赛队伍的结果为最好;我们将新的BioNLP方法结合到我们比较擅长的基于序列方法的预测方法,得到了水稻白叶枯病抗病基因的重排序;我们同样对BioNLP的事件提取做了语言学分析;在对水稻的基因进行分析时,我们同时将生物信息学的表型分析方法用到代谢物对象中,发现了可能的重要SNP;我们还在智能算法和可视化结果呈现发面做了一些尝试,也继续在传统的基于序列的功能基因预测领域做了一些工作。
项目成果
期刊论文数量(11)
专著数量(0)
科研奖励数量(0)
会议论文数量(4)
专利数量(0)
Gene prioritization of resistant rice gene against Xanthomas oryzae pv. oryzae by using text mining technologies.
水稻抗黄斑病基因的基因优先排序
- DOI:10.1155/2013/853043
- 发表时间:2013
- 期刊:BioMed research international
- 影响因子:--
- 作者:Xia J;Zhang X;Yuan D;Chen L;Webster J;Fang AC
- 通讯作者:Fang AC
Identification of discriminative features for biological event extraction through linguistically informed feature selection
通过语言信息特征选择识别生物事件提取的判别特征
- DOI:--
- 发表时间:2013
- 期刊:JOURNAL OF FOOD AGRICULTURE & ENVIRONMENT
- 影响因子:--
- 作者:Zhang, Xing;Xia, Jingbo;Webster, Jonathan;Fang, Alex Chengyu
- 通讯作者:Fang, Alex Chengyu
A novel feature selection strategy for enhanced biomedical event extraction using the Turku system.
使用图尔库系统增强生物医学事件提取的新特征选择策略
- DOI:10.1155/2014/205239
- 发表时间:2014
- 期刊:BioMed research international
- 影响因子:--
- 作者:Xia J;Fang AC;Zhang X
- 通讯作者:Zhang X
水稻代谢物通路中表型关联分析的SNP和代谢物的关联系统遗传学验证
- DOI:--
- 发表时间:2015
- 期刊:Frontiers in Plant Science
- 影响因子:5.6
- 作者:夏静波
- 通讯作者:夏静波
An efficient intrusion detection system based on support vector machines and gradually feature removal method
基于支持向量机和逐步特征去除方法的高效入侵检测系统
- DOI:10.1016/j.eswa.2011.07.032
- 发表时间:2012-01-01
- 期刊:EXPERT SYSTEMS WITH APPLICATIONS
- 影响因子:8.5
- 作者:Li, Yinhui;Xia, Jingbo;Dai, Kuobin
- 通讯作者:Dai, Kuobin
数据更新时间:{{ journalArticles.updateTime }}
{{
item.title }}
{{ item.translation_title }}
- DOI:{{ item.doi || "--"}}
- 发表时间:{{ item.publish_year || "--" }}
- 期刊:{{ item.journal_name }}
- 影响因子:{{ item.factor || "--"}}
- 作者:{{ item.authors }}
- 通讯作者:{{ item.author }}
数据更新时间:{{ journalArticles.updateTime }}
{{ item.title }}
- 作者:{{ item.authors }}
数据更新时间:{{ monograph.updateTime }}
{{ item.title }}
- 作者:{{ item.authors }}
数据更新时间:{{ sciAawards.updateTime }}
{{ item.title }}
- 作者:{{ item.authors }}
数据更新时间:{{ conferencePapers.updateTime }}
{{ item.title }}
- 作者:{{ item.authors }}
数据更新时间:{{ patent.updateTime }}
其他文献
其他文献
{{
item.title }}
{{ item.translation_title }}
- DOI:{{ item.doi || "--" }}
- 发表时间:{{ item.publish_year || "--"}}
- 期刊:{{ item.journal_name }}
- 影响因子:{{ item.factor || "--" }}
- 作者:{{ item.authors }}
- 通讯作者:{{ item.author }}
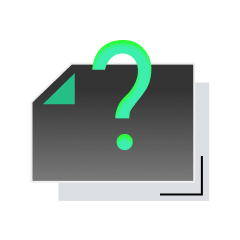
内容获取失败,请点击重试
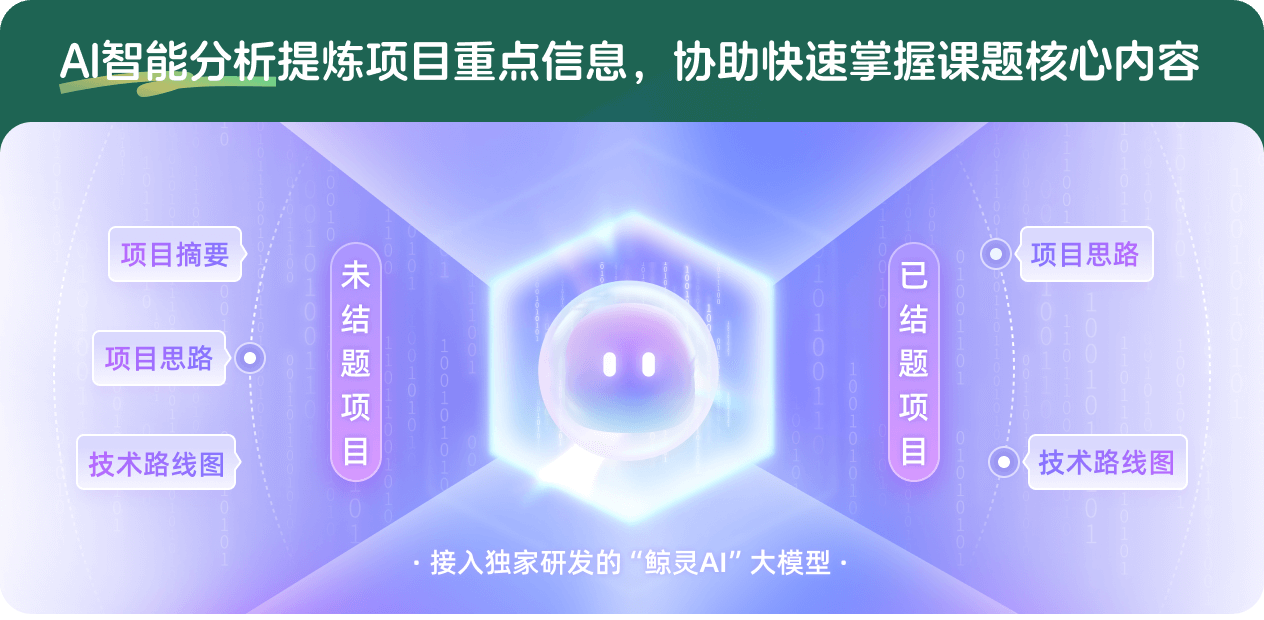
查看分析示例
此项目为已结题,我已根据课题信息分析并撰写以下内容,帮您拓宽课题思路:
AI项目摘要
AI项目思路
AI技术路线图
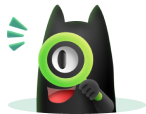
请为本次AI项目解读的内容对您的实用性打分
非常不实用
非常实用
1
2
3
4
5
6
7
8
9
10
您认为此功能如何分析更能满足您的需求,请填写您的反馈:
相似国自然基金
{{ item.name }}
- 批准号:{{ item.ratify_no }}
- 批准年份:{{ item.approval_year }}
- 资助金额:{{ item.support_num }}
- 项目类别:{{ item.project_type }}
相似海外基金
{{
item.name }}
{{ item.translate_name }}
- 批准号:{{ item.ratify_no }}
- 财政年份:{{ item.approval_year }}
- 资助金额:{{ item.support_num }}
- 项目类别:{{ item.project_type }}