多任务贝叶斯网络学习及其应用
项目介绍
AI项目解读
基本信息
- 批准号:61703416
- 项目类别:青年科学基金项目
- 资助金额:21.0万
- 负责人:
- 依托单位:
- 学科分类:F0601.人工智能基础
- 结题年份:2020
- 批准年份:2017
- 项目状态:已结题
- 起止时间:2018-01-01 至2020-12-31
- 项目参与者:黄金才; 冯旸赫; 王江; 游翰霖; 张雪婷; 曹晓丰; 王艺林; 岳瑞琪;
- 关键词:
项目摘要
Bayesian network is a well-known artificial intelligence model, it is easy to interpret and can be used to model the uncertainty. Hence, it is widely used in classification, predication and diagnosis. Given the training data, a Bayesian network can be automatically built with learning algorithms. However, the candidate structures exponentially increase with the number of nodes. Thus learning an accurate Bayesian network is very challenging. Recently, a new machine learning mechanism named Multi-task learning that utilizes the related information among multiple tasks to cover the lack of insufficient prior knowledge and training data, and improves the overall learning accuracy. Thus it has been attracting a great deal of attention. To improve the Bayesian network learning accuracy, we introduce the multi-task learning setting in this project, and explore the way of calculating task relatedness, the method of co-optimizing multiple Bayesian network structures, and the active transfer algorithm for parameter learning. Moreover, we develop the approximate inference algorithm, the method of key evidence analysis, and the performance evaluation system under the multi-task learning setting. To evaluate the proposed methods, we carry out experiments based on the BNs from well-known repository as well as two real world case studies: multiple user behaviors analysis in cyberspace and traumatic medical decision support in multiple hospitals. We expect this work to form a basis for analyzing and designing multi-task decision support system under uncertainty.
贝叶斯网络作为人工智能领域的重要模型,能够对问题的不确定性进行建模,且具有很强的可解释性,被广泛的应用在分类、预测、诊断等方面。在给定训练数据的情况下,贝叶斯网络可以通过学习算法自动的构建,然而由于可供选择的模型结构随节点数呈指数型增加,贝叶斯网络学习问题一直以来都是一个难点问题。近几年来,多任务学习由于能够利用不同任务间存在的相关性,进而弥补先验知识和数据的不足,提高学习精度,引起了学术界的广泛关注。本项目通过引入多任务条件,研究任务间相似度计算、多贝叶斯网络结构协同优化,以及网络参数的主动迁移学习的方法,从而期望获得更准确的模型。同时,通过研究多任务条件下贝叶斯网络的近似推理算法、关键证据集的分析方法,以及综合的模型评价方法,在公开数据和网络空间多用户行为分析、多医院创伤医疗决策两个具体应用中,对所提算法进行检验,为不确定环境下多任务辅助决策系统的分析和设计提供理论与方法支撑。
结项摘要
贝叶斯网络作为人工智能领域的重要模型,能够对问题的不确定性进行建模,且具有很强的可解释性,近年来在网络安全防护领域得到了高度关注。在给定训练数据的情况下,贝叶斯网络可以通过学习算法自动的构建,然而由于可供选择的模型结构随节点数呈指数型增加,贝叶斯网络学习问题一直以来都是一个难点问题。近几年来,多任务学习由于能够利用不同任务间存在的相关性,进而弥补先验知识和数据的不足,提高学习精度,引起了学术界的广泛关注。本项目通过引入多任务条件,研究贝叶斯网络数值优化算法,多个贝叶斯网络结构集成学习方法,从而获得了更准确的模型。. 由于在网络安全领域,网络流量数据分布在不同计算机终端上,因此可以自然的将多任务贝叶斯网络学习方法应用在XSS攻击流量检测上,通过任务之间的共享信息来提高算法的性能。此外,还构建了反映攻击特征的领域知识本体,将其作为知识库来提取有关攻击的特征,做为构建模型的基础,并利用威胁情报改进了攻击检测模型的学习效果,同时提出了一种节点重要性排序的方法对检测结果进行合理的解释,并在实际XSS攻击数据集上应用本项目模型和方法,取得了良好的效果。项目证明了多任务贝叶斯网络学习的可行性,同时开展了利用贝叶斯网络进行XSS攻击检测的系列研究,为网络安全与人工智能的交叉提供了一个可行的方向。
项目成果
期刊论文数量(15)
专著数量(0)
科研奖励数量(0)
会议论文数量(10)
专利数量(6)
世界一流大学研究生培养模式讨论——以人工智能课程为例
- DOI:--
- 发表时间:2018
- 期刊:计算机工程与科学
- 影响因子:--
- 作者:周鋆;曾平;杜振国;唐国明
- 通讯作者:唐国明
The Effective Methods for Intrusion Detection With Limited Network Attack Data: Multi-Task Learning and Oversampling
有限网络攻击数据入侵检测的有效方法:多任务学习和过采样
- DOI:10.1109/access.2020.3029100
- 发表时间:2020
- 期刊:IEEE Access
- 影响因子:3.9
- 作者:Sun Lijian;Zhou Yun;Wang Yanjuan;Zhu Cheng;Zhang Weiming
- 通讯作者:Zhang Weiming
基于串行分类算法的不平衡时间序列多分类方法
- DOI:--
- 发表时间:2019
- 期刊:南京师范大学学报(工程技术版)
- 影响因子:--
- 作者:陈俐名;黄诗茹;修保新;周鋆
- 通讯作者:周鋆
An ensemble learning approach for XSS attack detection with domain knowledge and threat intelligence
一种利用领域知识和威胁情报检测 XSS 攻击的集成学习方法
- DOI:10.1016/j.cose.2018.12.016
- 发表时间:2019-05
- 期刊:Computers & Security
- 影响因子:5.6
- 作者:Zhou Yun;Wang Peichao
- 通讯作者:Wang Peichao
Adaptive sampling using self-paced learning for imbalanced cancer data pre-diagnosis
使用自定进度学习的自适应采样来进行不平衡的癌症数据预诊断
- DOI:10.1016/j.eswa.2020.113334
- 发表时间:2020-08
- 期刊:Expert Systems with Applications
- 影响因子:8.5
- 作者:Wang Qingyong;Zhou Yun;Zhang Weiming;Tang Zhangui;Chen Xiaojing
- 通讯作者:Chen Xiaojing
数据更新时间:{{ journalArticles.updateTime }}
{{
item.title }}
{{ item.translation_title }}
- DOI:{{ item.doi || "--"}}
- 发表时间:{{ item.publish_year || "--" }}
- 期刊:{{ item.journal_name }}
- 影响因子:{{ item.factor || "--"}}
- 作者:{{ item.authors }}
- 通讯作者:{{ item.author }}
数据更新时间:{{ journalArticles.updateTime }}
{{ item.title }}
- 作者:{{ item.authors }}
数据更新时间:{{ monograph.updateTime }}
{{ item.title }}
- 作者:{{ item.authors }}
数据更新时间:{{ sciAawards.updateTime }}
{{ item.title }}
- 作者:{{ item.authors }}
数据更新时间:{{ conferencePapers.updateTime }}
{{ item.title }}
- 作者:{{ item.authors }}
数据更新时间:{{ patent.updateTime }}
其他文献
基于ePCANS模型的C2组织本体建模方法
- DOI:--
- 发表时间:2012
- 期刊:火力与指挥控制
- 影响因子:--
- 作者:成清;黄金才;刘忠;周鋆
- 通讯作者:周鋆
一种共面带状线馈电的圆极化双频微带天线
- DOI:--
- 发表时间:--
- 期刊:微波学报
- 影响因子:--
- 作者:吕艳青;杨雪霞;周鋆;江超
- 通讯作者:江超
其他文献
{{
item.title }}
{{ item.translation_title }}
- DOI:{{ item.doi || "--" }}
- 发表时间:{{ item.publish_year || "--"}}
- 期刊:{{ item.journal_name }}
- 影响因子:{{ item.factor || "--" }}
- 作者:{{ item.authors }}
- 通讯作者:{{ item.author }}
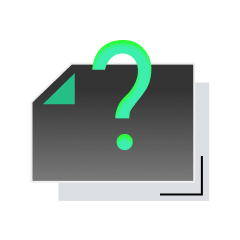
内容获取失败,请点击重试
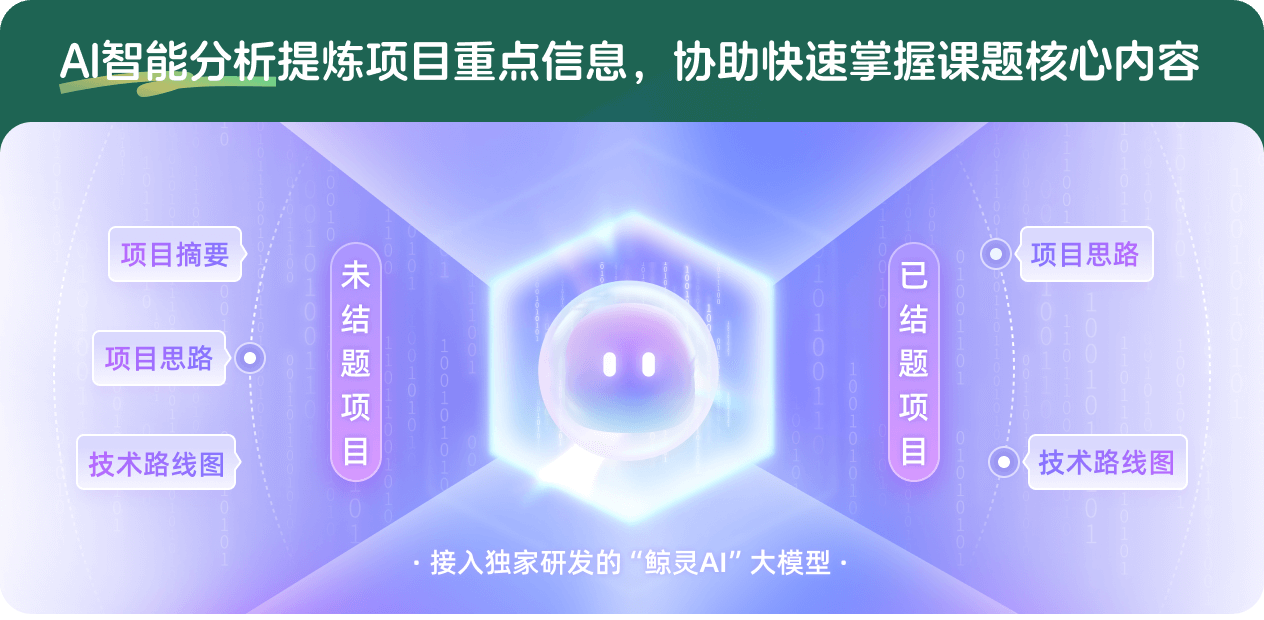
查看分析示例
此项目为已结题,我已根据课题信息分析并撰写以下内容,帮您拓宽课题思路:
AI项目摘要
AI项目思路
AI技术路线图
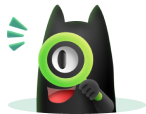
请为本次AI项目解读的内容对您的实用性打分
非常不实用
非常实用
1
2
3
4
5
6
7
8
9
10
您认为此功能如何分析更能满足您的需求,请填写您的反馈:
周鋆的其他基金
强对抗环境下智能算法鲁棒性机制研究
- 批准号:
- 批准年份:2022
- 资助金额:53 万元
- 项目类别:面上项目
强对抗环境下智能算法鲁棒性机制研究
- 批准号:62276272
- 批准年份:2022
- 资助金额:53.00 万元
- 项目类别:面上项目
相似国自然基金
{{ item.name }}
- 批准号:{{ item.ratify_no }}
- 批准年份:{{ item.approval_year }}
- 资助金额:{{ item.support_num }}
- 项目类别:{{ item.project_type }}
相似海外基金
{{
item.name }}
{{ item.translate_name }}
- 批准号:{{ item.ratify_no }}
- 财政年份:{{ item.approval_year }}
- 资助金额:{{ item.support_num }}
- 项目类别:{{ item.project_type }}