面向电子健康档案的患者健康轨迹动态过程建模及在呼吸道疾病的临床应用研究
项目介绍
AI项目解读
基本信息
- 批准号:U1609220
- 项目类别:联合基金项目
- 资助金额:210.0万
- 负责人:
- 依托单位:
- 学科分类:F0214.新型计算及其应用基础
- 结题年份:2020
- 批准年份:2016
- 项目状态:已结题
- 起止时间:2017-01-01 至2020-12-31
- 项目参与者:王苹莉; 舒林华; 浦剑; 曹桂涛; 金博; 严骏驰; 杨燕; 蒋鲲; 周异;
- 关键词:
项目摘要
In the healthcare field, electronic health records contain great scientific and marketing values by having rich clinical data that enable the study of disease progression and prognosis, both at individual as well as at the population level. Traditional methods for electronic health records failed to make full use of the data time information which is essential for accurate modeling of health trajectories. The focus of this project is the dynamic modeling of patient health trajectories represented in electronic health records with the goals to reveal the principles behind disease progression over time and lay the theoretical and algorithmic foundations for promoting the development of precision medicine. This project mainly covers the following topics: 1) network-based patient health trajectory modeling; 2) patient health trajectory clustering and segmentation; 3) study of noisy and missing health data; 4) typical applications and clinical case studies of health trajectory modeling (e.g. the clinical application of respiratory disease). The relevant research achievements are expected to not only provide new ideas and solutions for patient disease pathways, stratification of patient population and deep disease phenotyping, but also increase the availability and integrity of electronic health records by dealing with noisy and missing data. They are therefore both of theoretical and practical importance. The applicants have accumulated rich experiences in data mining and machine learning, by whom a feasible project plan is presented. These facts lay the foundation for the success of the project.
在医疗健康领域,电子健康记录中富含的临床数据,它使从个体到群体层面研究疾病发展与预后规律成为可能,具有极大的科学和市场价值。针对电子健康记录的传统研究方法未能充分利用数据中时间信息,因而不能准确对健康轨迹建模。本课题旨在基于电子健康记录对患者健康轨迹中蕴含的动态特性建模,揭示疾病随着时间变化过程背后的原理,为促进精准医疗发展提供相关的理论和算法基础。本课题的主要研究内容包括:1)基于网络的患者健康轨迹联合建模;2)患者健康轨迹聚类以及分割;3)健康数据丢失和噪声处理;4)健康轨迹建模的典型应用和临床案例分析(如呼吸道疾病的临床应用)。本课题的相关研究成果预计将为患者疾病预测、患者群体分类、疾病深度表现型分析等提供新的解决思路和方法,通过去噪和补全数据提升电子健康记录的完整性和可用性,具有重要的理论实践意义。申请人在数据挖掘和机器学习方面积累充分,研究方案切实可行,能保证本课题顺利完成。
结项摘要
近年来,随着数据科学与人工智能的发展,尤其是在精准医疗领域,健康轨迹数据建模已然成为实际应用问题中普遍存在的问题,推断与控制算法成为学术界和工业界都共同关注的重要需求。本项目针对这些重要需求,针对医疗健康轨迹数据数据,基于实际应用问题中的结构特性,设计随机点过程求解算法,并将其广泛应用于机器学习等应用中。..项目组与浙江大学、上海交通大学等国内外高校开展了深入广泛地合作,并超额完成预期任务。在国内外知名刊物发表期刊论文14篇,会议论文26篇,其中CCF A类论文16篇。本项目按照申请书设定的技术路线与年度计划有序进行。本项目在大规模事件数据建模方面的主要贡献包括:1)基于网络的患者健康轨迹联合建模;2)患者健康轨迹聚类以及分割;3)健康数据丢失和噪声处理;4)健康轨迹建模的典型应用和临床案例分析(如呼吸道疾病的临床应用)。本课题的相关研究成果预计将为患者疾病预测、患者群体分类、疾病深度表现型分析等提供新的解决思路和方法,通过去噪和补全数据提升电子健康记录的完整性和可用性,具有重要的理论实践意义。..本项目研究一系列精准医疗应用中的健康轨迹建模推断与控制,并取得了突破性的计算效果。
项目成果
期刊论文数量(14)
专著数量(0)
科研奖励数量(0)
会议论文数量(24)
专利数量(6)
Liver fibrosis c lassification based on transfer learning and an FCNet for ultrasound images
基于转移学习和超声图像 FCNet 的肝纤维化分类
- DOI:--
- 发表时间:2017
- 期刊:IEEE Access
- 影响因子:3.9
- 作者:Dan Meng;Libo Zhang;Guitao Cao;Bing Hu;Wenming Cao
- 通讯作者:Wenming Cao
Tongue Images Classification Based on Constrained High Dispersal Network.
基于约束高弥散网络的舌头图像分类
- DOI:10.1155/2017/7452427
- 发表时间:2017
- 期刊:Evidence-based complementary and alternative medicine : eCAM
- 影响因子:--
- 作者:Meng D;Cao G;Duan Y;Zhu M;Tu L;Xu D;Xu J
- 通讯作者:Xu J
An Empirical Study for Adopting Machine Learning Approaches for Gas Pipeline Flow Prediction
采用机器学习方法进行天然气管道流量预测的实证研究
- DOI:10.1155/2020/7842847
- 发表时间:2020-09
- 期刊:Mathematical Problems in Engineering
- 影响因子:--
- 作者:Guoliang Shen;Mufan Li;Jiale Lin;Jie Bao;Tao He
- 通讯作者:Tao He
EventThread: Visual Summarization and Stage Analysis of Event Sequence Data
EventThread:事件序列数据的可视化总结和阶段分析
- DOI:10.1109/tvcg.2017.2745320
- 发表时间:2018-01-01
- 期刊:IEEE TRANSACTIONS ON VISUALIZATION AND COMPUTER GRAPHICS
- 影响因子:5.2
- 作者:Guo, Shunan;Xu, Ke;Cao, Nan
- 通讯作者:Cao, Nan
Emerging Blood-Based Biomarkers for Predicting Response to Checkpoint Immunotherapy in Non-Small-Cell Lung Cancer.
用于预测非小细胞肺癌检查点免疫治疗反应的新兴血液生物标志物
- DOI:10.3389/fimmu.2020.603157
- 发表时间:2020
- 期刊:Frontiers in immunology
- 影响因子:7.3
- 作者:Li S;Zhang C;Pang G;Wang P
- 通讯作者:Wang P
数据更新时间:{{ journalArticles.updateTime }}
{{
item.title }}
{{ item.translation_title }}
- DOI:{{ item.doi || "--"}}
- 发表时间:{{ item.publish_year || "--" }}
- 期刊:{{ item.journal_name }}
- 影响因子:{{ item.factor || "--"}}
- 作者:{{ item.authors }}
- 通讯作者:{{ item.author }}
数据更新时间:{{ journalArticles.updateTime }}
{{ item.title }}
- 作者:{{ item.authors }}
数据更新时间:{{ monograph.updateTime }}
{{ item.title }}
- 作者:{{ item.authors }}
数据更新时间:{{ sciAawards.updateTime }}
{{ item.title }}
- 作者:{{ item.authors }}
数据更新时间:{{ conferencePapers.updateTime }}
{{ item.title }}
- 作者:{{ item.authors }}
数据更新时间:{{ patent.updateTime }}
其他文献
影像组学的回顾与展望
- DOI:--
- 发表时间:2017
- 期刊:上海医学
- 影响因子:--
- 作者:查宏远;浦剑;李郁欣
- 通讯作者:李郁欣
其他文献
{{
item.title }}
{{ item.translation_title }}
- DOI:{{ item.doi || "--" }}
- 发表时间:{{ item.publish_year || "--"}}
- 期刊:{{ item.journal_name }}
- 影响因子:{{ item.factor || "--" }}
- 作者:{{ item.authors }}
- 通讯作者:{{ item.author }}
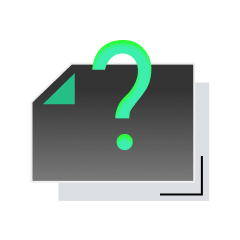
内容获取失败,请点击重试
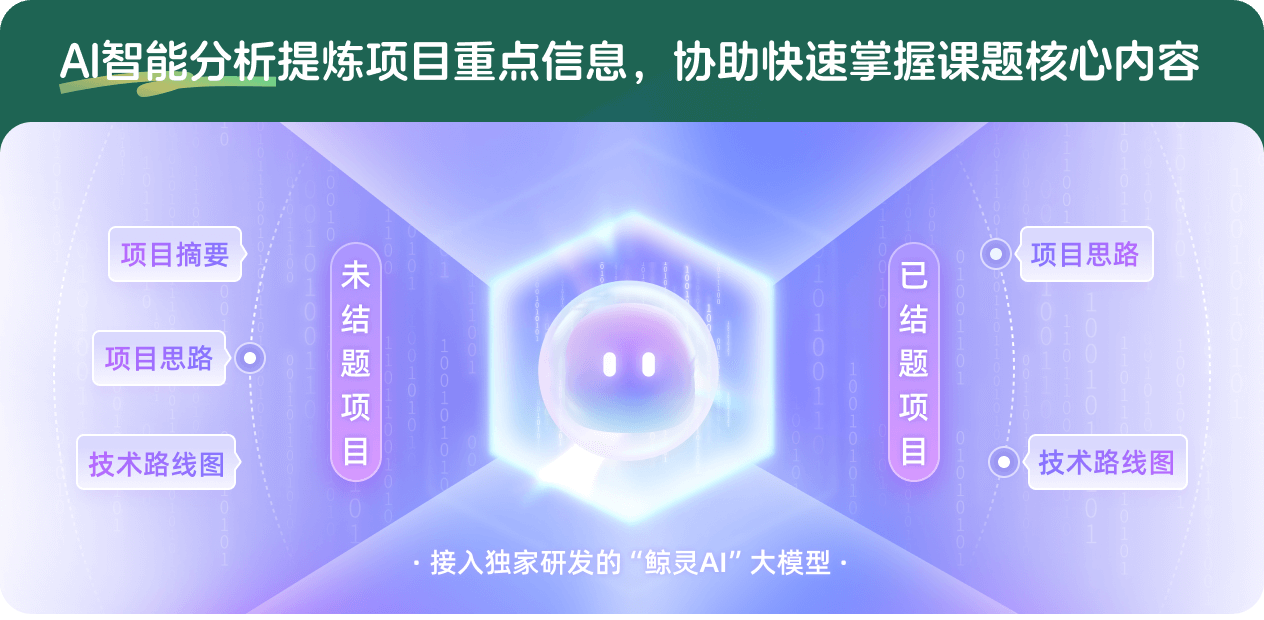
查看分析示例
此项目为已结题,我已根据课题信息分析并撰写以下内容,帮您拓宽课题思路:
AI项目摘要
AI项目思路
AI技术路线图
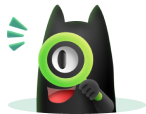
请为本次AI项目解读的内容对您的实用性打分
非常不实用
非常实用
1
2
3
4
5
6
7
8
9
10
您认为此功能如何分析更能满足您的需求,请填写您的反馈:
查宏远的其他基金
基于随机点过程的大规模事件数据的建模推断与控制
- 批准号:61672231
- 批准年份:2016
- 资助金额:65.0 万元
- 项目类别:面上项目
相似国自然基金
{{ item.name }}
- 批准号:{{ item.ratify_no }}
- 批准年份:{{ item.approval_year }}
- 资助金额:{{ item.support_num }}
- 项目类别:{{ item.project_type }}
相似海外基金
{{
item.name }}
{{ item.translate_name }}
- 批准号:{{ item.ratify_no }}
- 财政年份:{{ item.approval_year }}
- 资助金额:{{ item.support_num }}
- 项目类别:{{ item.project_type }}