多模式计算大数据系统资源的高效能优化方法研究
项目介绍
AI项目解读
基本信息
- 批准号:61906065
- 项目类别:青年科学基金项目
- 资助金额:24.0万
- 负责人:
- 依托单位:
- 学科分类:F0608.智能系统与人工智能安全
- 结题年份:2022
- 批准年份:2019
- 项目状态:已结题
- 起止时间:2020-01-01 至2022-12-31
- 项目参与者:--
- 关键词:
项目摘要
The current rough resource management and blind computing performance pursuit have been detrimental to the development of big data system. It is of great significance to study high energy-efficiency computing for this system. How to meet the dynamic computing requirements with the least resources under the system Qos constraints is one of the most important ways to achieve high energy-efficiency computing..This project is based on the development trend of big data systems supporting multiple computing models. Firstly, the characteristics, constraints and commonalities of big data applications, resource requests and resource management modes of the type system are analyzed, and a resource dynamic optimization model is established. Then, the dynamic resource providing optimization under the background of complex resource request is studied, and a deep reinforcement learning based hyper-heuristic prediction method is proposed to meet the high-precision requirement of resource request estimation. Optimize the number of active servers for the system based on these studies. Finally, this project studies the fair and efficient allocation of multi-resource under the condition of certain resource providing and practical application constraints. We propose a parallel collaborative optimization method that optimizes performance and rate to reduce server fragmentation resources. A parallel collaborative optimization method which can balance the optimization performance and rate of the algorithm is proposed to reduce server fragmentation resources.. The project takes the high energy-efficiency computing of multi-mode big data system as the engineering application background, and studies the key scientific problems of resource dynamic providing and allocation optimization. The project plans to propose efficient and practical technical methods to support the sustainable development of big data.
当前大数据系统粗犷资源管理与一味追求计算性能的发展方式已遭遇瓶颈,研究其高效能计算有着重要的意义。如何在系统Qos约束下,以最少的资源满足动态的计算需求是实现系统高效能计算最主要的途径之一。.本课题从大数据系统支持多计算模式发展趋势出发。首先,对该类型系统的大数据应用、资源请求、资源管理模式等特征、约束、共性进行分析,建立资源动态优化模型;然后,研究复杂资源请求背景下的资源动态供给优化,提出深度强化超启发预测方法,以满足资源请求预估的高精度需求,优化系统的活跃服务器数量;最后,在一定资源供给与实际约束条件下,研究多维资源高效公平分配优化,提出兼顾优化性能与速率的并行协同优化方法,以减少服务器碎片化资源。.课题以实际工程应用为背景,研究支持多计算模式大数据系统高效能计算的资源动态供给与分配优化的关键科学问题,提出高效、实际可行的技术方法,为大数据可持续发展提供助力。
结项摘要
当前大数据系统粗犷资源管理与一味追求计算性能的发展方式,造成了巨大的能耗开销与资源浪费。如何在系统Qos约束下,以最少的资源满足动态的计算需求是实现系统高效能计算最主要的途径之一。.项目从大数据系统支持多计算模式发展趋势出发。我们的研究内容包括:1)研究多模式计算系统的大数据应用、资源请求、资源管理模式等的特征、约束与共性,建立资源动态优化模型;2)研究复杂资源请求背景下的资源动态供给优化方法,以满足资源请求预估的高精度需求,优化系统的活跃服务器数量;3)研究在一定资源供给与实际约束条件下,多维资源高效公平分配优化方法,以减少服务器碎片化资源。.依托项目支持,项目组取得了以下研究成果:1)在问题模型方面,提出了一种顾效率优化公平约束的多维资源优化模型、一种兼顾通信和计算资源的联合优化模型、一种面向动态服务器供给的系统收益优化模型;2)在资源动态供给优化方法方面,提出了一种在线超启发式预测技术、一种基于多样性感知的集成预测算法;3)在资源高效分配优化方法方面,提出了大作业服务器期望剩余资源最少优先的启发式方法、一种基于深度强化学习的资源优化方法、一种分层次的资源优化方法。这些方法从不同角度针对不同类型的多模式计算系统提高了其计算效能,将为大数据可持续发展提供助力。
项目成果
期刊论文数量(6)
专著数量(0)
科研奖励数量(0)
会议论文数量(0)
专利数量(1)
面向复杂约束优化问题的进化算法综述
- DOI:10.13328/j.cnki.jos.006711
- 发表时间:2022
- 期刊:软件学报
- 影响因子:--
- 作者:陈少淼;陈瑞;梁伟;李仁发;李智勇
- 通讯作者:李智勇
An improved K-prototype based cluster algorithm for mixed educational data mining
一种改进的基于K原型的混合教育数据挖掘聚类算法
- DOI:--
- 发表时间:2022
- 期刊:Internationa l Journal of Embedded Systems
- 影响因子:--
- 作者:Yuan Wang;Liping Yang;Jun Wu;Shaomiao Chen
- 通讯作者:Shaomiao Chen
DRJOA: intelligent resource management optimization through deep reinforcement learning approach in edge computing
DRJOA:通过边缘计算中的深度强化学习方法实现智能资源管理优化
- DOI:10.1007/s10586-022-03768-z
- 发表时间:2022-12-15
- 期刊:CLUSTER COMPUTING-THE JOURNAL OF NETWORKS SOFTWARE TOOLS AND APPLICATIONS
- 影响因子:4.4
- 作者:Chen, Yifan;Chen, Shaomiao;Li, Zhiyong
- 通讯作者:Li, Zhiyong
HIQCO: A hierarchical optimization method for computation offloading and resource optimization in multi-cell mobile-edge computing systems
HIQCO:一种用于多小区移动边缘计算系统中计算卸载和资源优化的分层优化方法
- DOI:10.1109/access.2020.2977988
- 发表时间:2020
- 期刊:IEEE Access
- 影响因子:3.9
- 作者:Zhiyong Li;Chen Du;Shaomiao Chen
- 通讯作者:Shaomiao Chen
Diversity Aware-based Sequential Ensemble Learning for Robust Anomaly Detection
基于多样性感知的顺序集成学习,用于鲁棒异常检测
- DOI:10.1109/access.2020.2976850
- 发表时间:2020
- 期刊:IEEE Access
- 影响因子:3.9
- 作者:Jia Zhang;Zhiyong Li;Shaomiao Chen
- 通讯作者:Shaomiao Chen
数据更新时间:{{ journalArticles.updateTime }}
{{
item.title }}
{{ item.translation_title }}
- DOI:{{ item.doi || "--"}}
- 发表时间:{{ item.publish_year || "--" }}
- 期刊:{{ item.journal_name }}
- 影响因子:{{ item.factor || "--"}}
- 作者:{{ item.authors }}
- 通讯作者:{{ item.author }}
数据更新时间:{{ journalArticles.updateTime }}
{{ item.title }}
- 作者:{{ item.authors }}
数据更新时间:{{ monograph.updateTime }}
{{ item.title }}
- 作者:{{ item.authors }}
数据更新时间:{{ sciAawards.updateTime }}
{{ item.title }}
- 作者:{{ item.authors }}
数据更新时间:{{ conferencePapers.updateTime }}
{{ item.title }}
- 作者:{{ item.authors }}
数据更新时间:{{ patent.updateTime }}
其他文献
约束优化进化算法综述
- DOI:--
- 发表时间:2017
- 期刊:软件学报
- 影响因子:--
- 作者:李智勇;黄滔;陈少淼;李仁发
- 通讯作者:李仁发
基于生态策略的动态多目标优化算法
- DOI:--
- 发表时间:2014
- 期刊:计算机研究与发展
- 影响因子:--
- 作者:张世文;李智勇;陈少淼;李仁发
- 通讯作者:李仁发
云环境下超启发式能耗感知调度算法
- DOI:--
- 发表时间:2016
- 期刊:计算机工程与应用
- 影响因子:--
- 作者:陈少淼;李智勇;杨波;李彦武
- 通讯作者:李彦武
异构云环境多目标Memetic优化任务调度方法
- DOI:--
- 发表时间:2016
- 期刊:计算机学报
- 影响因子:--
- 作者:李智勇;陈少淼;杨波;李仁发
- 通讯作者:李仁发
其他文献
{{
item.title }}
{{ item.translation_title }}
- DOI:{{ item.doi || "--" }}
- 发表时间:{{ item.publish_year || "--"}}
- 期刊:{{ item.journal_name }}
- 影响因子:{{ item.factor || "--" }}
- 作者:{{ item.authors }}
- 通讯作者:{{ item.author }}
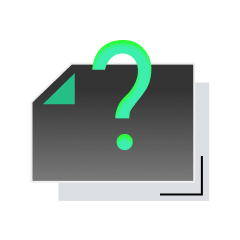
内容获取失败,请点击重试
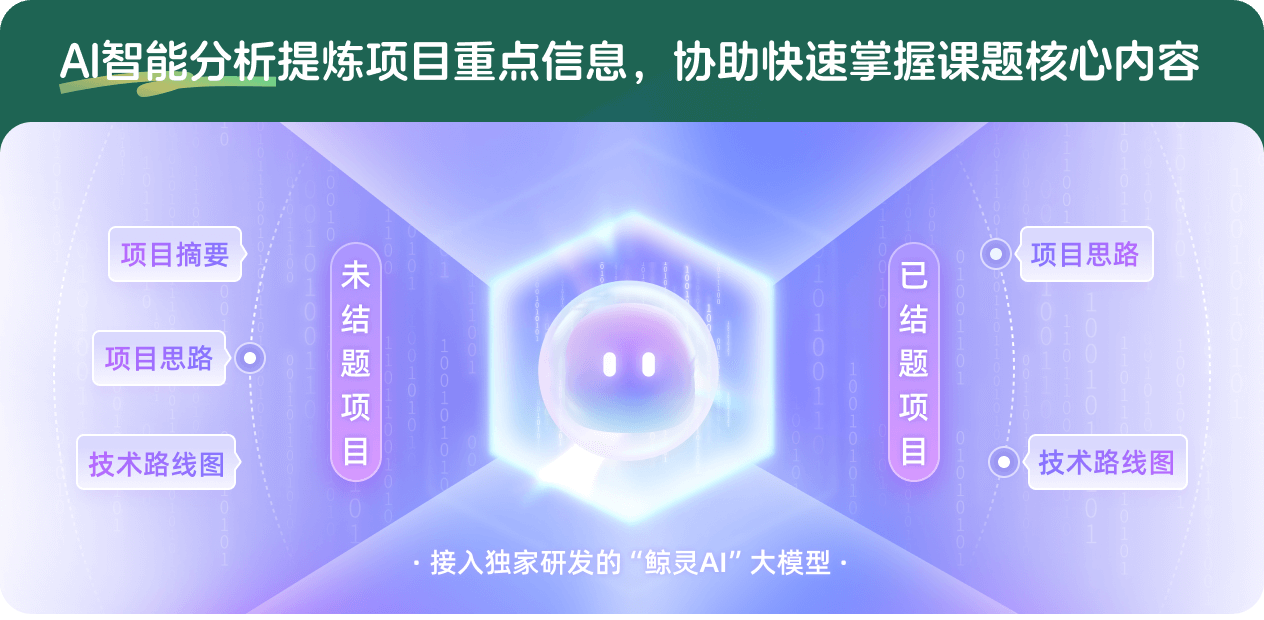
查看分析示例
此项目为已结题,我已根据课题信息分析并撰写以下内容,帮您拓宽课题思路:
AI项目摘要
AI项目思路
AI技术路线图
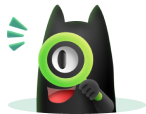
请为本次AI项目解读的内容对您的实用性打分
非常不实用
非常实用
1
2
3
4
5
6
7
8
9
10
您认为此功能如何分析更能满足您的需求,请填写您的反馈:
相似国自然基金
{{ item.name }}
- 批准号:{{ item.ratify_no }}
- 批准年份:{{ item.approval_year }}
- 资助金额:{{ item.support_num }}
- 项目类别:{{ item.project_type }}
相似海外基金
{{
item.name }}
{{ item.translate_name }}
- 批准号:{{ item.ratify_no }}
- 财政年份:{{ item.approval_year }}
- 资助金额:{{ item.support_num }}
- 项目类别:{{ item.project_type }}