基于深度学习的帧内预测编码及其网络的加速方法研究
项目介绍
AI项目解读
基本信息
- 批准号:61802105
- 项目类别:青年科学基金项目
- 资助金额:25.0万
- 负责人:
- 依托单位:
- 学科分类:F0210.计算机图像视频处理与多媒体技术
- 结题年份:2021
- 批准年份:2018
- 项目状态:已结题
- 起止时间:2019-01-01 至2021-12-31
- 项目参与者:郭凯; 王硕; 贲辉霞; 徐晨敏; 陈雷; 钱彪; 宋培培;
- 关键词:
项目摘要
The existing intra prediction encoding cannot meet the compression performance requirement for higher resolution video. The deep learning based intra prediction encoding is an effective way to improve compression performance. However, deep learning has the drawbacks of complex network and long computation time, which is the main obstacle for its application to the compression coding algorithm. Therefore, this project plans to research on the deep learning based intra-prediction encoding algorithm, model compression and VLSI acceleration of deep neural networks. The details are as follows: 1) An efficient deep learning based intra prediction encoding algorithm is designed, which can improve the prediction efficiency of complex structure and compression efficiency. 2) An efficient quantization and data coding methods of deep neural network parameters is studied, which can reduce the network complexity while ensuring the performance and benefiting to the hardware implementation; 3) Based on the quantized network, public operations in convolution are extracted and achieved in efficient basic units to accelerate the deep neural network and reduce computing time. This project can be the technical foundation for 8K television technology and the next generation coding standards, and it is conducive to the application of deep learning.
现有帧内预测编码难以满足更高分辨率视频压缩的性能需求,基于深度学习的帧内预测编码是提升压缩性能的有效途径。但深度学习中存在着网络结构过于复杂、计算时间过长的问题,该问题是将其应用于压缩编码的主要障碍。因此,本项目拟从基于深度学习的帧内预测编码、深度学习网络的模型压缩以及网络结构的VLSI加速三个方面展开研究。具体内容如下:1)研究基于深度学习的帧内预测编码算法,以提升对复杂纹理待编码块的预测效率,从而提高帧内预测编码的压缩性能;2)研究深度学习网络参数的高效量化及数据编码方法,在保证网络性能且有利于硬件实现的前提下,降低网络复杂度;3)在量化网络的基础上,分析并提取出卷积运算中的公共操作,设计高效的基本运算单元并合理布局,以实现网络结构的VLSI加速。本项目可以为8K电视技术的普及与下一代压缩编码标准的制定提供技术基础,同时有利于深度学习技术的应用推广。
结项摘要
现有帧内预测编码难以满足更高分辨率视频压缩的性能需求,基于深度学习的帧内预测编码是提升压缩性能的有效途径。但深度学习中存在着网络结构过于复杂、计算时间过长的问题,该问题是将其应用于压缩编码的主要障碍。针对以上问题,本项目分别从帧内预测编码算法、深度学习网络的模型压缩以及VLSI加速三个方面进行了研究。具体包括:1)深入分析遥感图像及人脸深度图像的数据特征,有针对性地设计出高效的帧内预测编码方法,用于提升图像的压缩编码效率;2)基于知识蒸馏及预量化技术,设计并提出高效的网络结构剪枝及权重参数量化方法,用于在尽量不损失网络性能的前提下,降低网络结构的运算复杂度及存储数据量;3)针对深度神经网络中最为常用的卷积运算部分,设计并提出一种高效的卷积运算VLSI加速电路,用于完成卷积运算的加速电路设计。本项目可以为下一代压缩编码标准的制定提供技术基础,并为深度学习技术的应用推广提供理论基础和关键技术支持。
项目成果
期刊论文数量(2)
专著数量(0)
科研奖励数量(0)
会议论文数量(0)
专利数量(4)
A novel lossless compression encoding framework for SAR remote sensing images
一种新型SAR遥感图像无损压缩编码框架
- DOI:10.1007/s11760-020-01763-8
- 发表时间:2020
- 期刊:Signal, Image and Video Processing
- 影响因子:--
- 作者:Fan Chunxiao;Hu Zhou;Jia Lu;Min Hai
- 通讯作者:Min Hai
A novel lossless compression framework for facial depth images in expression recognition
一种新颖的表情识别中面部深度图像无损压缩框架
- DOI:10.1007/s11042-021-10796-1
- 发表时间:2021-04
- 期刊:Multimedia Tools and Applications
- 影响因子:3.6
- 作者:Fan Chunxiao;Li Fu;Jiao Yang;Liu Xueliang
- 通讯作者:Liu Xueliang
数据更新时间:{{ journalArticles.updateTime }}
{{
item.title }}
{{ item.translation_title }}
- DOI:{{ item.doi || "--"}}
- 发表时间:{{ item.publish_year || "--" }}
- 期刊:{{ item.journal_name }}
- 影响因子:{{ item.factor || "--"}}
- 作者:{{ item.authors }}
- 通讯作者:{{ item.author }}
数据更新时间:{{ journalArticles.updateTime }}
{{ item.title }}
- 作者:{{ item.authors }}
数据更新时间:{{ monograph.updateTime }}
{{ item.title }}
- 作者:{{ item.authors }}
数据更新时间:{{ sciAawards.updateTime }}
{{ item.title }}
- 作者:{{ item.authors }}
数据更新时间:{{ conferencePapers.updateTime }}
{{ item.title }}
- 作者:{{ item.authors }}
数据更新时间:{{ patent.updateTime }}
其他文献
全谱拟合定量研究气候对土壤成壤机制的约束:以鲁粤土壤剖面为例
- DOI:10.16461/j.cnki.1000-4734.2019.39.091
- 发表时间:2020
- 期刊:矿物学报
- 影响因子:--
- 作者:樊春晓;张晓科;潘宇观;蔡元峰
- 通讯作者:蔡元峰
其他文献
{{
item.title }}
{{ item.translation_title }}
- DOI:{{ item.doi || "--" }}
- 发表时间:{{ item.publish_year || "--"}}
- 期刊:{{ item.journal_name }}
- 影响因子:{{ item.factor || "--" }}
- 作者:{{ item.authors }}
- 通讯作者:{{ item.author }}
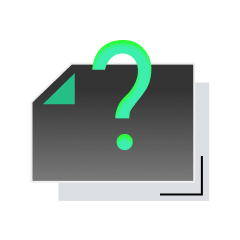
内容获取失败,请点击重试
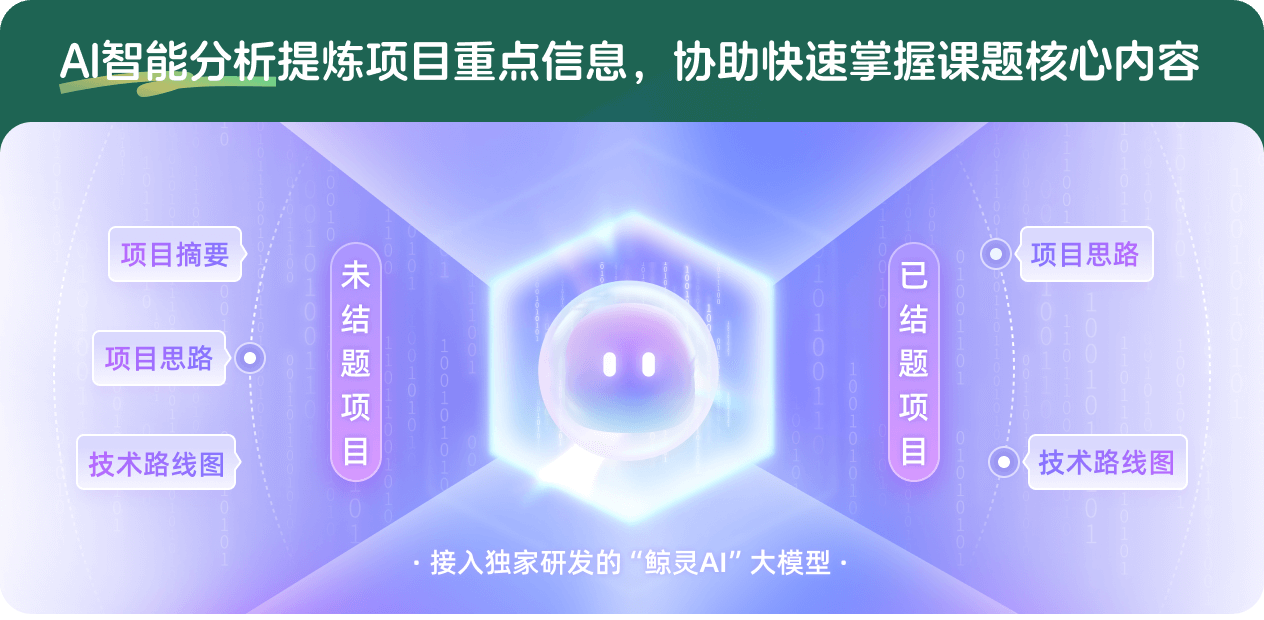
查看分析示例
此项目为已结题,我已根据课题信息分析并撰写以下内容,帮您拓宽课题思路:
AI项目摘要
AI项目思路
AI技术路线图
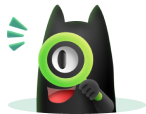
请为本次AI项目解读的内容对您的实用性打分
非常不实用
非常实用
1
2
3
4
5
6
7
8
9
10
您认为此功能如何分析更能满足您的需求,请填写您的反馈:
相似国自然基金
{{ item.name }}
- 批准号:{{ item.ratify_no }}
- 批准年份:{{ item.approval_year }}
- 资助金额:{{ item.support_num }}
- 项目类别:{{ item.project_type }}
相似海外基金
{{
item.name }}
{{ item.translate_name }}
- 批准号:{{ item.ratify_no }}
- 财政年份:{{ item.approval_year }}
- 资助金额:{{ item.support_num }}
- 项目类别:{{ item.project_type }}