基于机器学习融合精确性和多样性的电子商务协同过滤推荐方法研究
项目介绍
AI项目解读
基本信息
- 批准号:71402083
- 项目类别:青年科学基金项目
- 资助金额:20.0万
- 负责人:
- 依托单位:
- 学科分类:G0209.商务智能与数字商务
- 结题年份:2017
- 批准年份:2014
- 项目状态:已结题
- 起止时间:2015-01-01 至2017-12-31
- 项目参与者:冯仕红; 杨潇; 岳厚光; 郑绪伟; 李延改; 朱晓光;
- 关键词:
项目摘要
Resulting from the Internet related information techniques, nowadays electronic commerce (E-commerce) becomes one of the major types of business industries in the world. However, one of the major challenges of E-commerce is the “information overload” problem, i.e., the Web has been flooded with an overwhelming amount of information, which has become increasingly unmanageable and created a poverty of attention and a need to allocate that attention efficiently. As an effective technique addressing the problem, recommender systems attempt to make predictions and give recommendations based on a large collection of users’ historical behaviors and ratings. They have become a de facto standard and must-owned tools for e-commerce to promote business and to help customers discovering new products...Collaborative filtering is currently one of the most successful recommendation algorithms. It is widely-used in variety of recommender systems, effectively handling the information overloading problem in the Internet...However, there are still lots of challenges to further improve the performance of the collaborative filtering algorithms. For example, diversity is one of the most critical issues in recommender systems, but it has not been received enough attention from the academic and industry communities. In addition, the accuracy of collaborative filtering algorithms is not desirable enough resulting from the data sparsity problem...In this project, we plan to investigate a novel collaborative filtering framework, aiming to effectively improve the recommendation diversity and accuracy simultaneously based on machine learning techniques. In particular, we are going to propose a novel recommendation diversity metric based on the user-item rating matrix, which is expected to demonstrates a high positive correlation with popular content-based diversity metrics. Then we can learn an accurate and diverse set of items for recommendation to each target user based the structured support vector machine algorithm. In addition, we plan to improve the recommendation accuracy via modeling various preference interests of the target users, and incorporating the user relationship data in social networks to reduce the impact of the data sparsity problem...This project will definitely improve the profit contribution rate of the recommender systems in E-business, and thus promote the quick development of the E-commerce enterprises. In addition, the research results can also guide the practices and applications of collaborative filtering algorithms to related problems in business intelligence and data mining areas.
当前电子商务网站存在“信息超载”问题,推荐系统是解决信息超载问题的有效工具,而协同过滤是目前推荐系统中广泛使用的、最成功的推荐方法。但是,现有的电子商务协同过滤方法对推荐多样性问题没有引起足够的重视,且存在的数据稀疏等问题也导致推荐精确性也不尽如人意,制约了协同过滤方法的进一步发展。本项目拟针对融合精确性和多样性的协同过滤推荐模型的基本理论和方法展开研究,通过深入研究推荐多样性测度,基于机器学习提出一种能够将推荐多样性和精确性测度有机地融合在一起的协同过滤模型;深入挖掘用户偏好兴趣,对用户的真正意图进行建模和精确分析,并通过引入社交网络的用户关系数据以缓解数据稀疏问题,大幅度提高推荐系统的精确性和多样性。开展本课题的研究,不仅可以进一步提高推荐系统在电子商务企业的利润贡献率,推动电子商务企业的蓬勃快速发展,研究成果还能够为商务智能、数据挖掘等相关领域的实践与应用提供理论指导与方法借鉴。
结项摘要
本课题围绕当前电子商务网站存在的“信息超载”问题展开讨论,针对现有方法对推荐多样性问题没有引起足够的重视,且存在的数据稀疏等问题也导致推荐精确性也不尽如人意等问题,研究了解决该问题最有效的协同过滤推荐方法。主要创新工作包括:(1)提出了一种基于排序学习的兼顾精确性和效率的Listwise协同过滤推荐方法,并研究了排序学习的并行化框架,在确保精确性的同时大大提升了学习效率;(2)研究了融合推荐精确性和多样性的协同过滤推荐方法,并依据用户的行为日志具体讨论了用户需求的多样性;(3)研究并提出了融合社交网络信任关系和用户偏好兴趣点模型的协同过滤推荐方法,并研究了高效的图数据检索和挖掘算法;(4)研究了基于用户评分信息的推荐多样性和新奇性测度,并提出了面向新奇性测度的基本推荐方法。在本项目的资助下,研究团队在国内外权威期刊和国际知名学术会议共发表学术论文10篇,其中在SCI检索的期刊中发表论文6篇,包括3篇论文发表在国际权威学术期刊《IEEE Transactions on Knowledge and Data Engineering》、《ACM Transactions on Information Systems》和《ACM Transactions on Intelligent Systems and Technology》;4篇论文分别发表在国际权威学术会议WWW 2017、SIGIR 2017、ICDE 2016和SIGIR 2015中;获得软件著作权2项,培养博士生2人,培养硕士生3人。各项指标均超额完成了预期目标。研究成果不仅将进一步提高推荐系统在电子商务企业的利润贡献率,推动电子商务企业的蓬勃快速发展,还将为商务智能、数据挖掘等相关领域的实践与应用提供理论指导与方法借鉴。
项目成果
期刊论文数量(6)
专著数量(0)
科研奖励数量(0)
会议论文数量(4)
专利数量(0)
Behavior-Based Personalization in Web Search
网络搜索中基于行为的个性化
- DOI:10.1002/asi.23735
- 发表时间:2017
- 期刊:JOURNAL OF THE ASSOCIATION FOR INFORMATION SCIENCE AND TECHNOLOGY
- 影响因子:3.5
- 作者:Cai Fei;Wang Shuaiqiang;de Rijke Maarten
- 通讯作者:de Rijke Maarten
A Cooperative Coevolution Framework for Parallel Learning to Rank
并行学习排序的合作协同进化框架
- DOI:10.1109/tkde.2015.2453952
- 发表时间:2015-12
- 期刊:IEEE Transactions on Knowledge and Data Engineering (TKDE)
- 影响因子:--
- 作者:Byron J. Gao;Ke Wang;Hady W. Lauw;Jun Ma
- 通讯作者:Jun Ma
A survey of serendipity in recommender systems
推荐系统中的偶然性调查
- DOI:10.1016/j.knosys.2016.08.014
- 发表时间:2016-11-01
- 期刊:KNOWLEDGE-BASED SYSTEMS
- 影响因子:8.8
- 作者:Kotkov, Denis;Wang, Shuaiqiang;Veijalainen, Jari
- 通讯作者:Veijalainen, Jari
Ranking-Oriented Collaborative Filtering: A Listwise Approach
面向排名的协同过滤:列表方法
- DOI:10.1145/2960408
- 发表时间:2016-12-01
- 期刊:ACM TRANSACTIONS ON INFORMATION SYSTEMS
- 影响因子:5.6
- 作者:Wang, Shuaiqiang;Huang, Shanshan;Veijalainen, Jari
- 通讯作者:Veijalainen, Jari
数据更新时间:{{ journalArticles.updateTime }}
{{
item.title }}
{{ item.translation_title }}
- DOI:{{ item.doi || "--"}}
- 发表时间:{{ item.publish_year || "--" }}
- 期刊:{{ item.journal_name }}
- 影响因子:{{ item.factor || "--"}}
- 作者:{{ item.authors }}
- 通讯作者:{{ item.author }}
数据更新时间:{{ journalArticles.updateTime }}
{{ item.title }}
- 作者:{{ item.authors }}
数据更新时间:{{ monograph.updateTime }}
{{ item.title }}
- 作者:{{ item.authors }}
数据更新时间:{{ sciAawards.updateTime }}
{{ item.title }}
- 作者:{{ item.authors }}
数据更新时间:{{ conferencePapers.updateTime }}
{{ item.title }}
- 作者:{{ item.authors }}
数据更新时间:{{ patent.updateTime }}
其他文献
Weighted-Tau Rank: 一种采用加权Kendall Tau的面向排序的协同过滤算法
- DOI:--
- 发表时间:2014
- 期刊:中文信息学报
- 影响因子:--
- 作者:孙建凯;王帅强;马军
- 通讯作者:马军
LDA-CF:一种混合协同过滤方法
- DOI:--
- 发表时间:2014
- 期刊:中文信息学报
- 影响因子:--
- 作者:廉涛;马军;王帅强;崔超然
- 通讯作者:崔超然
其他文献
{{
item.title }}
{{ item.translation_title }}
- DOI:{{ item.doi || "--" }}
- 发表时间:{{ item.publish_year || "--"}}
- 期刊:{{ item.journal_name }}
- 影响因子:{{ item.factor || "--" }}
- 作者:{{ item.authors }}
- 通讯作者:{{ item.author }}
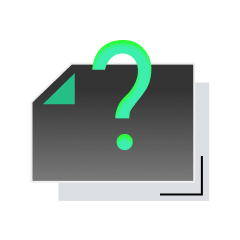
内容获取失败,请点击重试
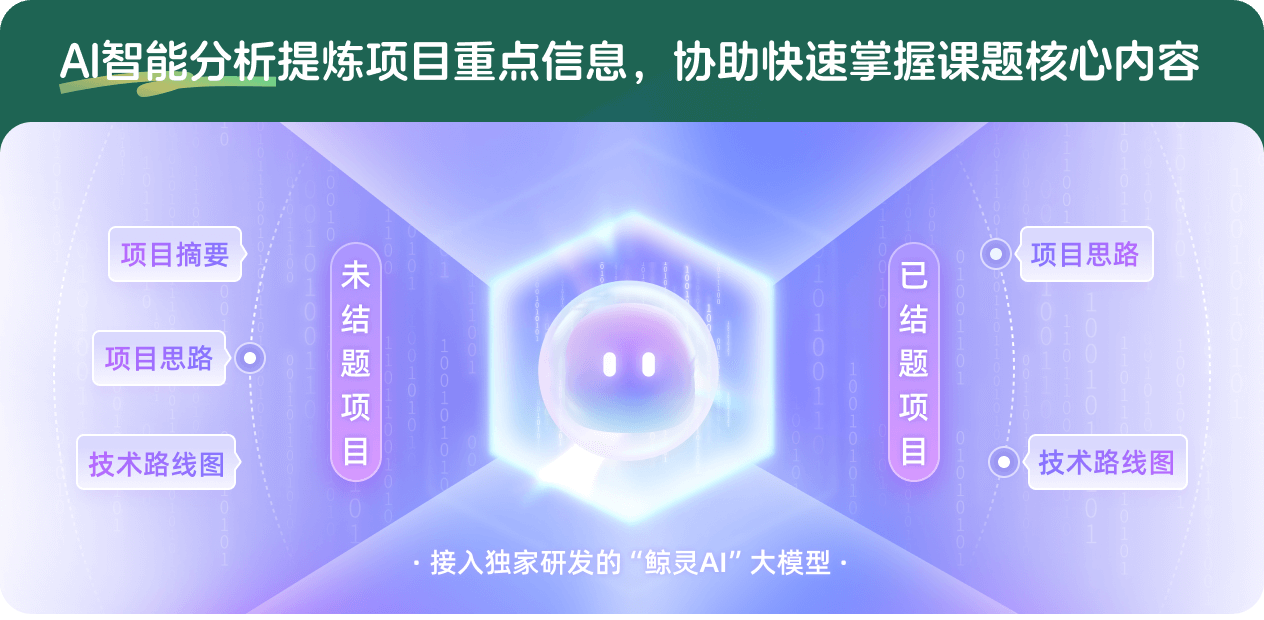
查看分析示例
此项目为已结题,我已根据课题信息分析并撰写以下内容,帮您拓宽课题思路:
AI项目摘要
AI项目思路
AI技术路线图
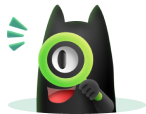
请为本次AI项目解读的内容对您的实用性打分
非常不实用
非常实用
1
2
3
4
5
6
7
8
9
10
您认为此功能如何分析更能满足您的需求,请填写您的反馈:
相似国自然基金
{{ item.name }}
- 批准号:{{ item.ratify_no }}
- 批准年份:{{ item.approval_year }}
- 资助金额:{{ item.support_num }}
- 项目类别:{{ item.project_type }}
相似海外基金
{{
item.name }}
{{ item.translate_name }}
- 批准号:{{ item.ratify_no }}
- 财政年份:{{ item.approval_year }}
- 资助金额:{{ item.support_num }}
- 项目类别:{{ item.project_type }}