求解标准形式的大规模离散不适定问题的Krylov迭代法正则化理论和算法
项目介绍
AI项目解读
基本信息
- 批准号:11771249
- 项目类别:面上项目
- 资助金额:48.0万
- 负责人:
- 依托单位:
- 学科分类:A0502.数值代数
- 结题年份:2021
- 批准年份:2017
- 项目状态:已结题
- 起止时间:2018-01-01 至2021-12-31
- 项目参与者:康文洁; 杨艳飞; 尚旭阳; 黄金枝; 李海波; 王法; 孙晓琳; 赖福辉;
- 关键词:
项目摘要
Numerical solutions of large discrete ill-posed problems are an extremely important and difficult research field. This kind of problem arises from numerous disciplines and has very extensive applications, such as mage deblurring, signal processing, geophysics, computerized tomography, heat propagation, biomedical and optical imaging, groundwater modeling, and many others. Typically, they come from discretization of the first kind Fredholm integral equation. The resulting discrete ill-posed problem is highly ill-conditioned and the right-hand side b is noisy, caused by measurement, modeling or discretization errors, and is typically contaminated by a white noise, and the singular values of the coefficient matrix A decay to zero without a noticeable gap. Under the continuous and discrete Picard conditions, the underlying continuous ill-posed problem and the discrete ill-posed problem have bounded true solutions, respectively. Because of the presence of noise and the extreme ill-conditioning of A, the naive solution of the discrete ill-posed problem is unbounded, and has bear no relation to the true solution. Therefore, one has to use regularization to extract a best possible approximation to the true solution. In principle, regularizing an ill-posed problem is to replace it by a well-posed one, such that the error is compensated by the gain in stability. For small and/or moderate sized problems, the truncated singular value decomposition (TSVD) method and standard-form Tikhonov regularization are most commonly used regularization methods, and they have been proved to be reliable and effective for computing best possible approximations to the true solution under certain assumptions on the true solution. The critical point on them is the determination of an optimal regularizartion parameter. For large problems, only iterative solvers are computationally viable, due to the computational complexity of both space and time. One major class of iterative solvers is Krylov iterative solvers that project the large problem onto a sequence of low dimensional Krylov subspaces and compute iterates to approximate the true solution. Of Krylov iterative solvers, the LSQR method and its mathematically equivalent CGLS method, which implicitly applies the Conjugate Gradient (CG) method to the normal equations, have been most commonly used. The Krylov solvers CGME and LSMR are also choices. These solvers have been known to have general regularizing effects and exhibit semi-convergence: The iterates converge to the true solution and the residual norms decrease in an initial stage; then afterwards the noise starts to deteriorate the iterates so that they start to diverge from the true solution and instead converge to the naïve solution. Since 1979, a fundamental and paramount long-standing concern is: Is the regularized solution by each of these methods at semi-convergence a best possible one? That is, is it at least as accurate as the best TSVD or Tikhonov solution? It has turned out that the behavior of an ill-posed problem critically depends on the decay rates of the singular values and the Picard condition. Depending on such decay rates, the problems are classified as three kinds: severely ill-posed, moderately and mildly ill-posed. The main goal of this project is to establish a rigorous regularization theory of CGLS, LSQR, CGME and LSMR for the three kinds of problems and for the first time give definitive conclusions on the above open question. The theory will play the central role in guiding correct use of the methods and identifying if a best possible solution has been found, and it will also derive effective developments of Krylov iterative solvers.
大规模离散不适定问题的数值求解是一个非常重要和困难的研究领域,其中的系数矩阵A的奇异值衰减到零,且随着奇异值的变小,相邻之间没有明显的隔离,右端项b是不精确的,带有(白)噪声。当准确的右端项满足Picard条件时,不适定问题的真解存在。然而,用标准方式得到的解是无界的,和真解没有任何关系。因此,必须对问题使用正则化方法,在稳定性和精度之间达到最佳的平衡,以得到最优可能的近似解。半个世纪以来,求解标准形式的大规模离散不适定问题的主要方法是以共轭梯度方法为代表的Krylov子空间迭代法,最著名和常用的是LSQR, CGLS, CGME和LSMR。但这些方法能否得到最优可能的近似解一直没有确定性的结论。本项目将建立这几个方法对严重、中度和温和不适定问题的严格正则化理论,解决1979年提出的关于方法对这三类问题能否得到最优的近似解这一公开问题,对方法的正确与合理使用提供理论指导,并用之开发新算法。
结项摘要
大规模离散不适定问题的数值求解是一个非常重要和困难的研究领域,在信号处理、图像去噪、地球物理等中有广泛的应用。问题中的系数矩阵A的奇异值衰减到零,且相邻的之间没有明显的隔离,右端项b是不精确的,带有高斯噪声。当准确的右端项满足Picard条件时,不适定问题的解存在。然而,用标准方式得到的解是无界的,和真解没有任何关系。因此,必须使用正则化方法,在稳定性和精度之间达到最佳的平衡,以得到最优可能的近似解。求解标准形式正则化的大规模离散不适定问题的主要方法是以共轭梯度方法为代表的Krylov子空间迭代法,最著名和常用的是LSQR, CGME和LSMR。但这些方法能否得到最优可能的近似解一直没有确定性的结论。本项目建立了这几个方法对严重、中度和温和不适定问题的严格正则化理论,揭示了这几个方法正则化方法相互之间的密切关系,证明了对严重不适定问题和中度不适定问题,LSQR和LSMR能得到最优可能的近似解,但CGME无论对哪类问题,得到的解一般都不是最优的。提出了求解一般形式正则化的大规模离散不适定问题的随机化算法和联合双对角化方法,分别建立了方法的正则化理论,开发了数值算法,给出了可靠的停机准则和最优正则化参数的选取方法。标准形式和一般形式正则化的大规模不适定问题的正则化分析的基本手段分别是奇异值分解(SVD)和广义奇异值分解(GSVD),本项目提出了计算部分SVD的Jacobi-Davidson型方法,找到了内部校正方程组最低求解精度要求,最大限度地提高了方法的计算效率;研究了文献中已有的将GSVD转化为两种广义特征值问题格式,证明了选取哪种格式能得到更高精度的计算解,对于计算部分GSVD的联合双对角化方法,揭示了联合双对角化方法有限精度运算下的形态。标准形式和一般形式的Tikhonov正则化问题和信頼域子问题的Lagrange乘子型形式问题密切相关。求解后者的最著名方法是广义Lanczos方法,该方法四个核心的收敛性问题中的两个一直没有结果。我们彻底解决了收敛性问题,并建立了结果相互之间的关系,为设计灵活实用的停机准则提供了可靠的理论指导。
项目成果
期刊论文数量(12)
专著数量(0)
科研奖励数量(0)
会议论文数量(0)
专利数量(0)
On choices of formulations of computing the generalized singular value decomposition of a large matrix pair
大矩阵对广义奇异值分解计算公式的选择
- DOI:10.1007/s11075-020-00984-9
- 发表时间:2019-07
- 期刊:Numerical Algorithms
- 影响因子:2.1
- 作者:Huang Jinzhi;Jia Zhongxiao
- 通讯作者:Jia Zhongxiao
Regularization properties of Krylov iterative solvers CGME and LSMR for linear discrete ill-posed problems with an application to truncated randomized SVDs
用于线性离散不适定问题的 Krylov 迭代求解器 CGME 和 LSMR 的正则化特性及其在截断随机 SVD 中的应用
- DOI:10.1007/s11075-019-00865-w
- 发表时间:2020-02-24
- 期刊:NUMERICAL ALGORITHMS
- 影响因子:2.1
- 作者:Jia, Zhongxiao
- 通讯作者:Jia, Zhongxiao
On Inner Iterations of Jacobi-Davidson Type Methods for Large SVD Computations
大型 SVD 计算的 Jacobi-Davidson 型方法的内迭代
- DOI:10.1137/18m1192019
- 发表时间:2017-11
- 期刊:SIAM Journal on Scientific Computing
- 影响因子:3.1
- 作者:Huang Jinzhi;Jia Zhongxiao
- 通讯作者:Jia Zhongxiao
The low rank approximations and Ritz values in LSQR for linear discrete ill-posed problem
线性离散不适定问题的 LSQR 中的低秩近似和 Ritz 值
- DOI:10.1088/1361-6420/ab6f42
- 发表时间:2018-11
- 期刊:Inverse Problems
- 影响因子:2.1
- 作者:Jia Zhongxiao
- 通讯作者:Jia Zhongxiao
Regularization properties of LSQR for linear discrete ill-posed problems in the multiple singular value case and best, near best and general low rank approximations
多奇异值情况下线性离散不适定问题的 LSQR 正则化特性以及最佳、近最佳和一般低秩近似
- DOI:10.1088/1361-6420/ab9c45
- 发表时间:2020-03
- 期刊:Inverse Problems
- 影响因子:2.1
- 作者:Jia Zhongxiao
- 通讯作者:Jia Zhongxiao
数据更新时间:{{ journalArticles.updateTime }}
{{
item.title }}
{{ item.translation_title }}
- DOI:{{ item.doi || "--"}}
- 发表时间:{{ item.publish_year || "--" }}
- 期刊:{{ item.journal_name }}
- 影响因子:{{ item.factor || "--"}}
- 作者:{{ item.authors }}
- 通讯作者:{{ item.author }}
数据更新时间:{{ journalArticles.updateTime }}
{{ item.title }}
- 作者:{{ item.authors }}
数据更新时间:{{ monograph.updateTime }}
{{ item.title }}
- 作者:{{ item.authors }}
数据更新时间:{{ sciAawards.updateTime }}
{{ item.title }}
- 作者:{{ item.authors }}
数据更新时间:{{ conferencePapers.updateTime }}
{{ item.title }}
- 作者:{{ item.authors }}
数据更新时间:{{ patent.updateTime }}
其他文献
Some properties of LSQR for large sparse linear least squares problems
大型稀疏线性最小二乘问题的 LSQR 的一些性质
- DOI:10.1007/s11424-010-7190-1
- 发表时间:2010-09
- 期刊:Journal of Systems Science & Complexity
- 影响因子:2.1
- 作者:贾仲孝
- 通讯作者:贾仲孝
计算大型Hermite矩阵最小特征对
- DOI:--
- 发表时间:--
- 期刊:中国科学-A辑, 2008年38卷3期(中文), 2008年51卷5期(英文版),将发表。
- 影响因子:--
- 作者:贾仲孝;王震
- 通讯作者:王震
The Rayleigh-Ritz Method, Refinement and Arnoldi Process for Periodic Matrix Pairs
周期矩阵对的 Rayleigh-Ritz 方法、细化和 Arnoldi 过程
- DOI:--
- 发表时间:--
- 期刊:Journal of Computational and Applied Mathematics
- 影响因子:2.4
- 作者:贾仲孝;Tiexiang Li;Wen-Wei Lin;Eric King-wah Chu;Hung-Yuan Fan
- 通讯作者:Hung-Yuan Fan
其他文献
{{
item.title }}
{{ item.translation_title }}
- DOI:{{ item.doi || "--" }}
- 发表时间:{{ item.publish_year || "--"}}
- 期刊:{{ item.journal_name }}
- 影响因子:{{ item.factor || "--" }}
- 作者:{{ item.authors }}
- 通讯作者:{{ item.author }}
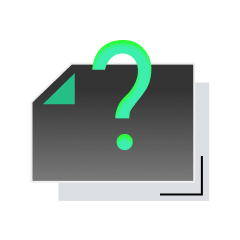
内容获取失败,请点击重试
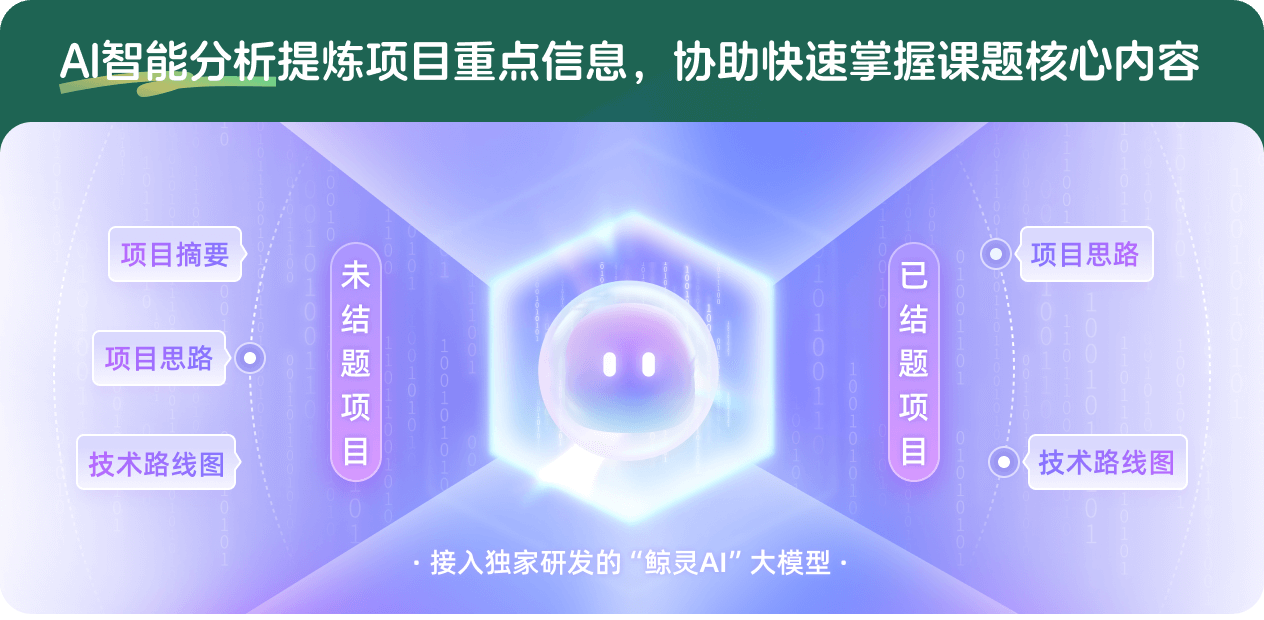
查看分析示例
此项目为已结题,我已根据课题信息分析并撰写以下内容,帮您拓宽课题思路:
AI项目摘要
AI项目思路
AI技术路线图
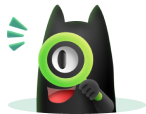
请为本次AI项目解读的内容对您的实用性打分
非常不实用
非常实用
1
2
3
4
5
6
7
8
9
10
您认为此功能如何分析更能满足您的需求,请填写您的反馈:
贾仲孝的其他基金
计算大规模矩阵的部分奇异值分解和矩阵对的部分广义奇异值分解的数值算法
- 批准号:12171273
- 批准年份:2021
- 资助金额:51 万元
- 项目类别:面上项目
大规模线性方程组的稀疏近似逆预处理方法及应用
- 批准号:11371219
- 批准年份:2013
- 资助金额:50.0 万元
- 项目类别:面上项目
第8届国际工业与应用数学大会程序委员会工作会议
- 批准号:11226021
- 批准年份:2012
- 资助金额:18.0 万元
- 项目类别:数学天元基金项目
求解大规模矩阵问题的非准确方法和全局投影方法
- 批准号:11071140
- 批准年份:2010
- 资助金额:25.0 万元
- 项目类别:面上项目
大规模矩阵特征问题及相关问题的准确和非准确数值方法
- 批准号:10771116
- 批准年份:2007
- 资助金额:18.0 万元
- 项目类别:面上项目
解大规模矩阵线性和非线性特征问题的精化投影类方法及其应用
- 批准号:10471074
- 批准年份:2004
- 资助金额:14.0 万元
- 项目类别:面上项目
相似国自然基金
{{ item.name }}
- 批准号:{{ item.ratify_no }}
- 批准年份:{{ item.approval_year }}
- 资助金额:{{ item.support_num }}
- 项目类别:{{ item.project_type }}
相似海外基金
{{
item.name }}
{{ item.translate_name }}
- 批准号:{{ item.ratify_no }}
- 财政年份:{{ item.approval_year }}
- 资助金额:{{ item.support_num }}
- 项目类别:{{ item.project_type }}